INDENT: Incremental Online Decision Tree Training for Domain-Specific Systems-on-Chip.
ICCAD(2022)
摘要
The performance and energy efficiency potential of heterogeneous architectures has fueled domain-specific systems-on-chip (DSSoCs) that integrate general-purpose and domain-specialized hardware accelerators. Decision trees (DTs) perform high-quality, low-latency task scheduling to utilize the massive parallelism and heterogeneity in DSSoCs effectively. However, offline trained DT scheduling policies can quickly become ineffective when applications or hardware configurations change. There is a critical need for runtime techniques to train DTs incrementally without sacrificing accuracy since current training approaches have large memory and computational power requirements. To address this need, we propose INDENT, an incremental online DT framework to update the scheduling policy and adapt it to unseen scenarios. INDENT updates DT schedulers at runtime using only 1-8% of the original training data embedded during training. Thorough evaluations with hardware platforms and DSSoC simulators demonstrate that INDENT performs within 5% of a DT trained from scratch using the entire dataset and outperforms current state-of-the-art approaches.
更多查看译文
关键词
Domain-specific system-on-chip,online learning,incremental training,decision trees,task scheduling,resource management,low-power,ultra-low latency
AI 理解论文
溯源树
样例
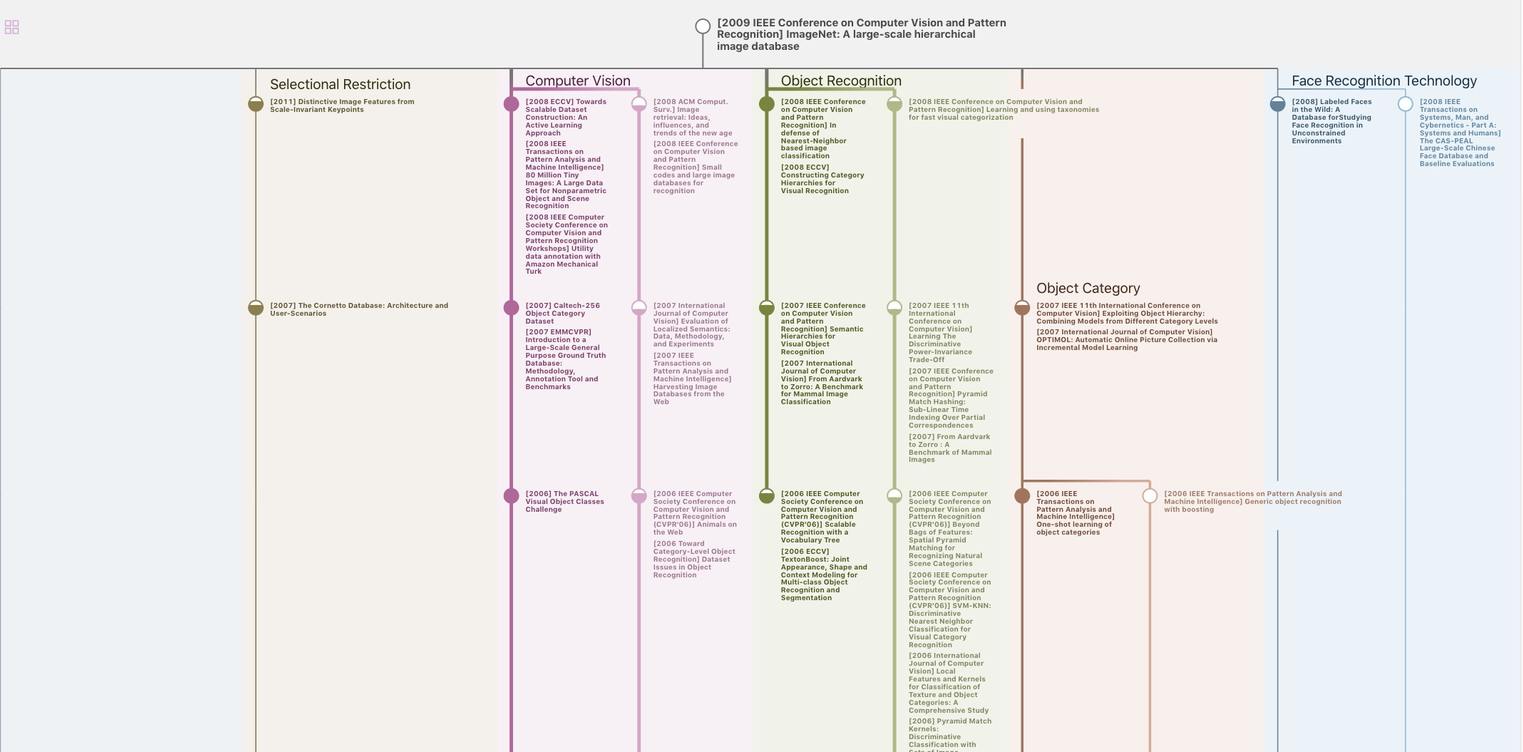
生成溯源树,研究论文发展脉络
Chat Paper
正在生成论文摘要