DCIM-GCN: Digital Computing-in-Memory to Efficiently Accelerate Graph Convolutional Networks.
ICCAD(2022)
摘要
Computing-in-memory (CIM) is emerging as a promising architecture to accelerate graph convolutional networks (GCNs) normally bounded by redundant and irregular memory transactions. Current analog based CIM requires frequent analog and digital conversions (AD/DA) that dominate the overall area and power consumption. Furthermore, the analog non-ideality degrades the accuracy and reliability of CIM. In this work, an SRAM based digital CIM system is proposed to accelerate memory intensive GCNs, namely DCIM-GCN, which covers innovations from CIM circuit level eliminating costly AD/DA converters to architecture level addressing irregularity and sparsity of graph data. DCIM-GCN achieves 2.07X, 1.76X, and 1.89× speedup and 29.98×, 1.29×, and 3.73× energy efficiency improvement on average over CIM based PIMGCN, TARe, and PIM-GCN, respectively.
更多查看译文
关键词
Graph Neural Networks,Computing-in-Memory
AI 理解论文
溯源树
样例
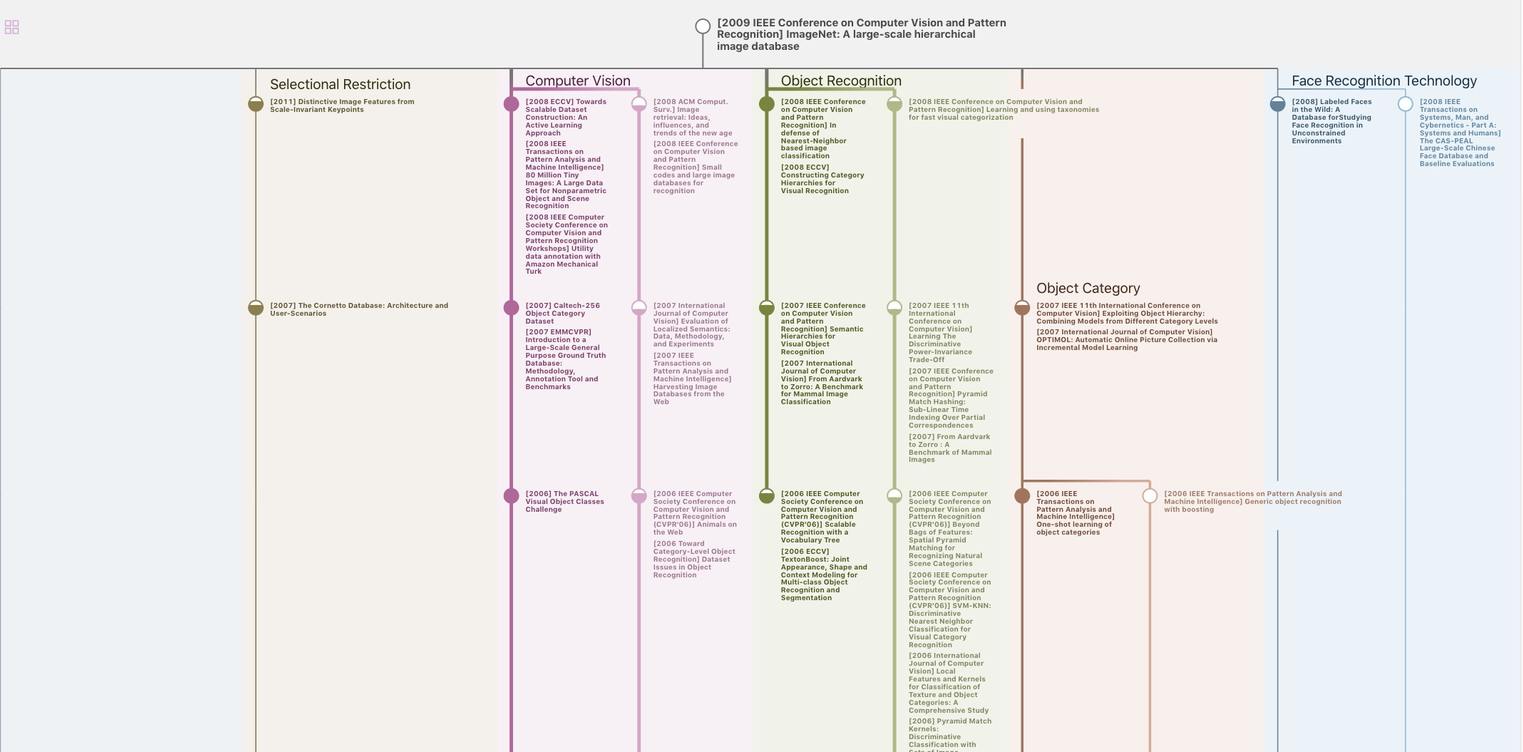
生成溯源树,研究论文发展脉络
Chat Paper
正在生成论文摘要