Probing Out-of-Distribution Robustness of Language Models with Parameter-Efficient Transfer Learning
arxiv(2023)
摘要
As the size of the pre-trained language model (PLM) continues to increase, numerous parameter-efficient transfer learning methods have been proposed recently to compensate for the tremendous cost of fine-tuning. Despite the impressive results achieved by large pre-trained language models (PLMs) and various parameter-efficient transfer learning (PETL) methods on sundry benchmarks, it remains unclear if they can handle inputs that have been distributionally shifted effectively. In this study, we systematically explore how the ability to detect out-of-distribution (OOD) changes as the size of the PLM grows or the transfer methods are altered. Specifically, we evaluated various PETL techniques, including fine-tuning, Adapter, LoRA, and prefix-tuning, on three different intention classification tasks, each utilizing various language models with different scales.
更多查看译文
关键词
language models,transfer learning,out-of-distribution,parameter-efficient
AI 理解论文
溯源树
样例
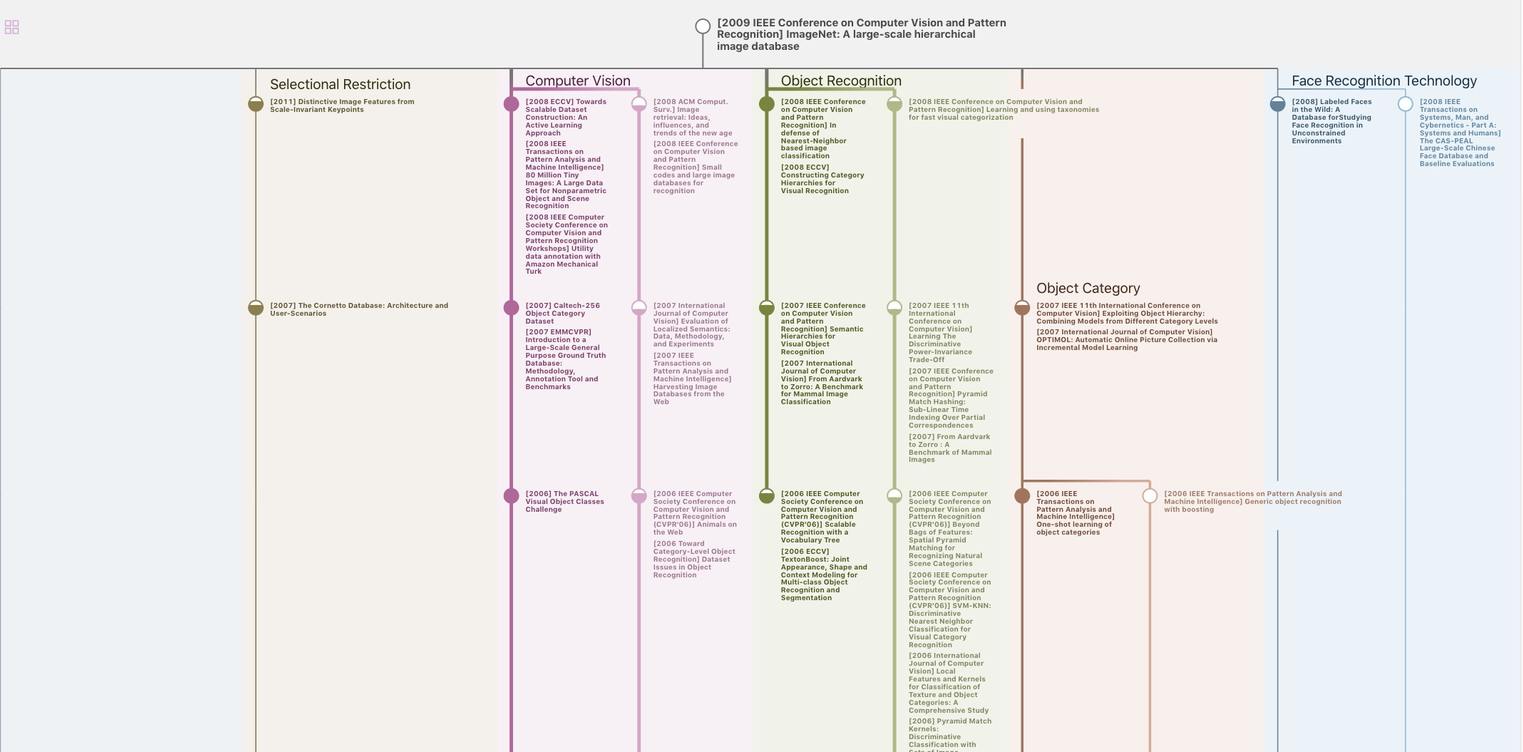
生成溯源树,研究论文发展脉络
Chat Paper
正在生成论文摘要