Investigating the Important Temporal Modulations for Deep-Learning-Based Speech Activity Detection
2022 IEEE Spoken Language Technology Workshop (SLT)(2023)
摘要
We describe a learnable modulation spectrogram feature for speech activity detection (SAD). Modulation features capture the temporal dynamics of each frequency subband. We compute learnable modulation spectrogram features by first calculating the log-mel spectrogram. Next, we filter each frequency subband with a bandpass filter that contains a learnable center frequency. The resulting SAD system was evaluated on the Fearless Steps Phase-04 SAD challenge. Experimental results showed that temporal modulations around the 4–6 Hz range are crucial for deep-learning-based SAD. These experimental results align with previous studies that found slow temporal modulation to be most important for speech-processing tasks and speech intelligibility. Additionally, we found that the learnable modulation spectrogram feature outperforms both the standard log-mel and fixed modulation spectrogram features on the Fearless Steps Phase-04 SAD test set.
更多查看译文
关键词
speech activity detection,temporal modulation
AI 理解论文
溯源树
样例
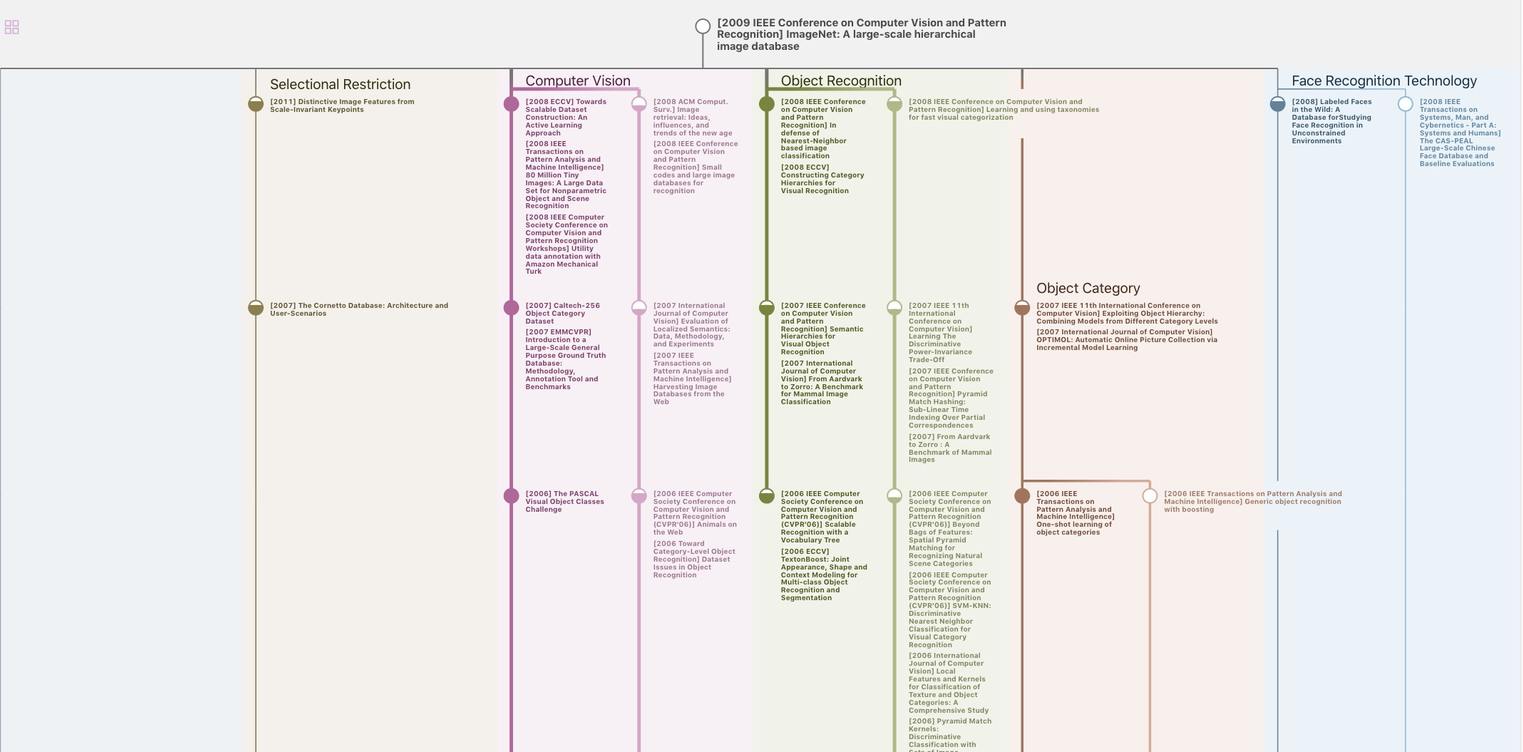
生成溯源树,研究论文发展脉络
Chat Paper
正在生成论文摘要