Biased-sample empirical likelihood weighting for missing data problems: an alternative to inverse probability weighting
Journal of the Royal Statistical Society Series B: Statistical Methodology(2023)
摘要
Abstract Inverse probability weighting (IPW) is widely used in many areas when data are subject to unrepresentativeness, missingness, or selection bias. An inevitable challenge with the use of IPW is that the IPW estimator can be remarkably unstable if some probabilities are very close to zero. To overcome this problem, at least three remedies have been developed in the literature: stabilizing, thresholding, and trimming. However, the final estimators are still IPW-type estimators, and inevitably inherit certain weaknesses of the naive IPW estimator: they may still be unstable or biased. We propose a biased-sample empirical likelihood weighting (ELW) method to serve the same general purpose as IPW, while completely overcoming the instability of IPW-type estimators by circumventing the use of inverse probabilities. The ELW weights are always well defined and easy to implement. We show theoretically that the ELW estimator is asymptotically normal and more efficient than the IPW estimator and its stabilized version for missing data problems. Our simulation results and a real data analysis indicate that the ELW estimator is shift-equivariant, nearly unbiased, and usually outperforms the IPW-type estimators in terms of mean square error.
更多查看译文
关键词
causal inference, empirical likelihood, inverse probability weighting, missing data
AI 理解论文
溯源树
样例
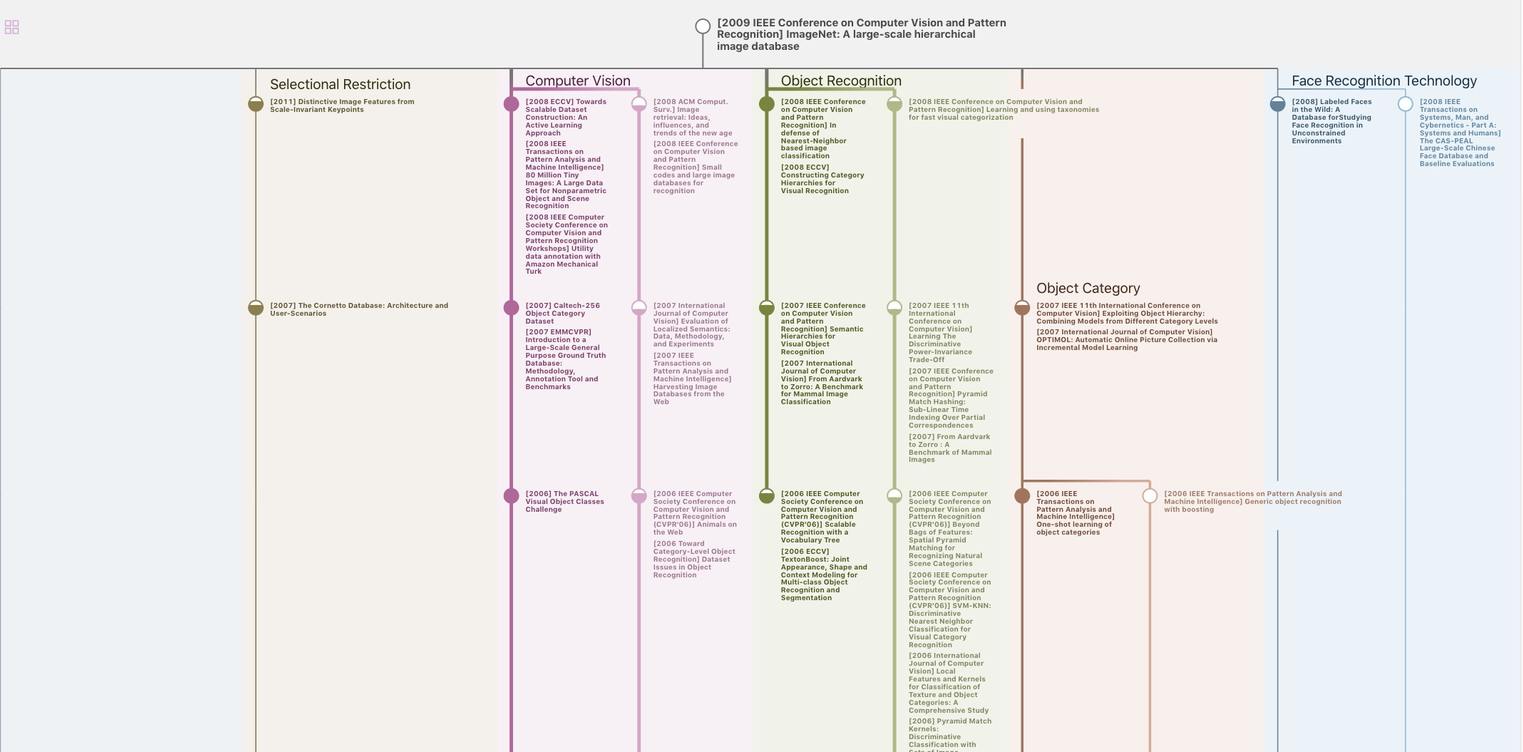
生成溯源树,研究论文发展脉络
Chat Paper
正在生成论文摘要