Learning Diversity Attributes in Multi-Session Recommendations
2022 IEEE International Conference on Big Data (Big Data)(2022)
摘要
Diversity in recommendation has been studied extensively. It has been shown that maximizing diversity subject to constrained relevance yields high user engagement over time. Existing work largely relies on setting some attributes that are used to craft an item similarity function and diversify results. In this paper, we examine the question of learning diversity attributes. That is particularly important when users receive recommendations over multiple sessions. We devise two main approaches to look for the best diversity attribute in each session: the first is a generalization of traditional diversity algorithms and the second is based on reinforcement learning. We implement both approaches and run extensive experiments on a semi-synthetic dataset. Our results demonstrate that learning diversity attributes yields a higher overall diversity than traditional diversity algorithms. We also find that training policies using reinforcement learning is more efficient in terms of response time, in particular for high dimensional data.
更多查看译文
关键词
diversity attributes,multi-session
AI 理解论文
溯源树
样例
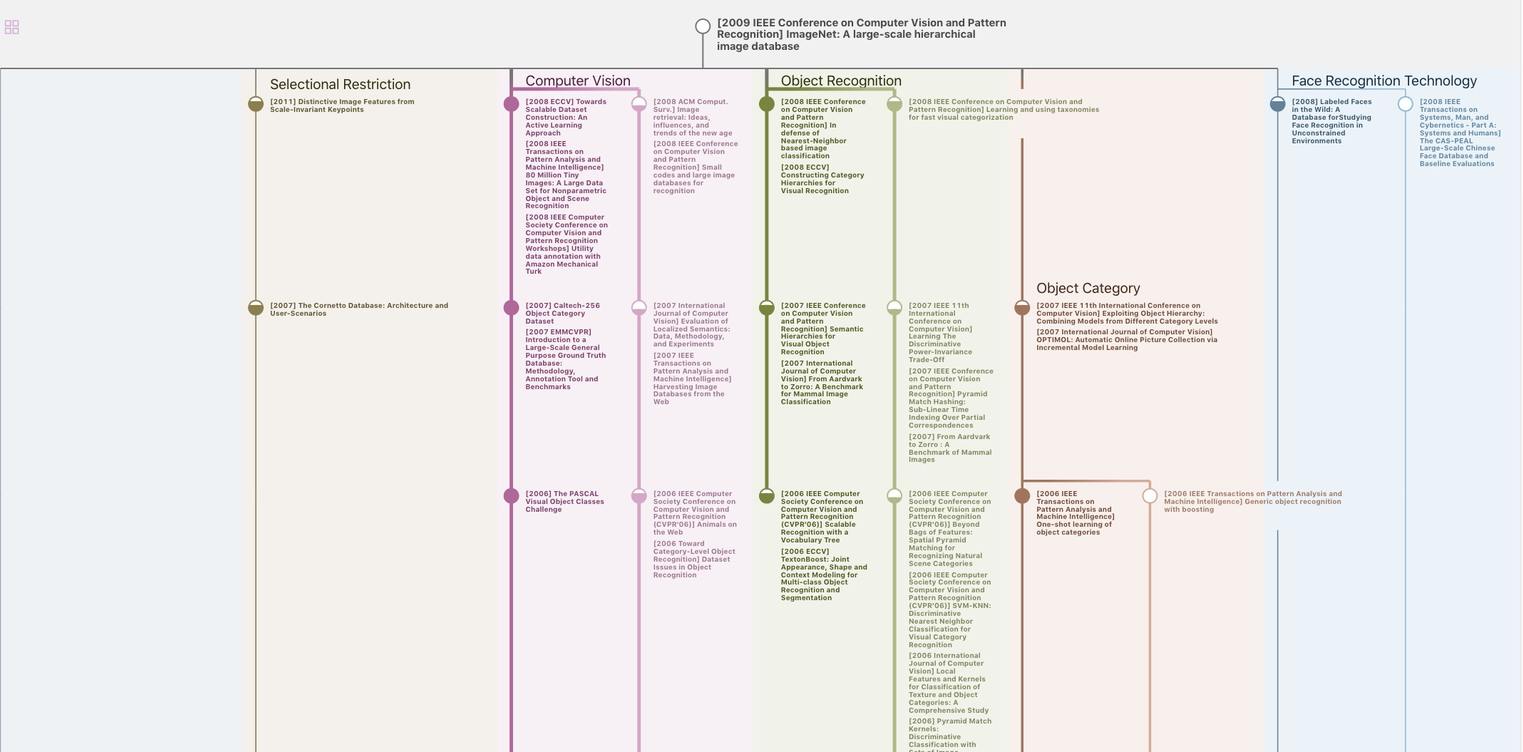
生成溯源树,研究论文发展脉络
Chat Paper
正在生成论文摘要