Reformulation of Distributionally Robust Problems Depending on Elementary Functions
arxiv(2023)
摘要
In this work, we present algorithmically tractable reformulations of distributionally robust optimization (DRO) problems. The considered ambiguity sets can exploit information on moments as well as confidence sets. Typically, reformulation approaches using duality theory need to make strong assumptions on the structure of the underlying constraints, such as convexity in the decisions or concavity in the uncertainty. In contrast, here we present a very general duality-based reformulation approach for distributionally robust problems that are allowed to depend on elementary functions, which renders the problem nonlinear and nonconvex. In order to be able to reformulate the semiinfinite constraints nevertheless, a safe approximation is presented that is realized by a discretized counterpart. Its reformulation leads to a mixed-integer positive semidefinite problem that yields sufficient conditions for distributional robustness of the original problem. For specific models with uncertainties that are only one-dimensional, it is proven that with increasingly fine discretizations, the discretized reformulation converges to the robust counterpart of the original distributionally robust problem. The approach is made concrete for a one-dimensional robust chance-constrained problem, where the discretized counterpart results in a mixed-integer linear problem. We study a challenging, fundamental task in particle separation that appears in material design. Computational results for a realistic setting show that the safe approximation yields robust solutions of high-quality within short time.
更多查看译文
AI 理解论文
溯源树
样例
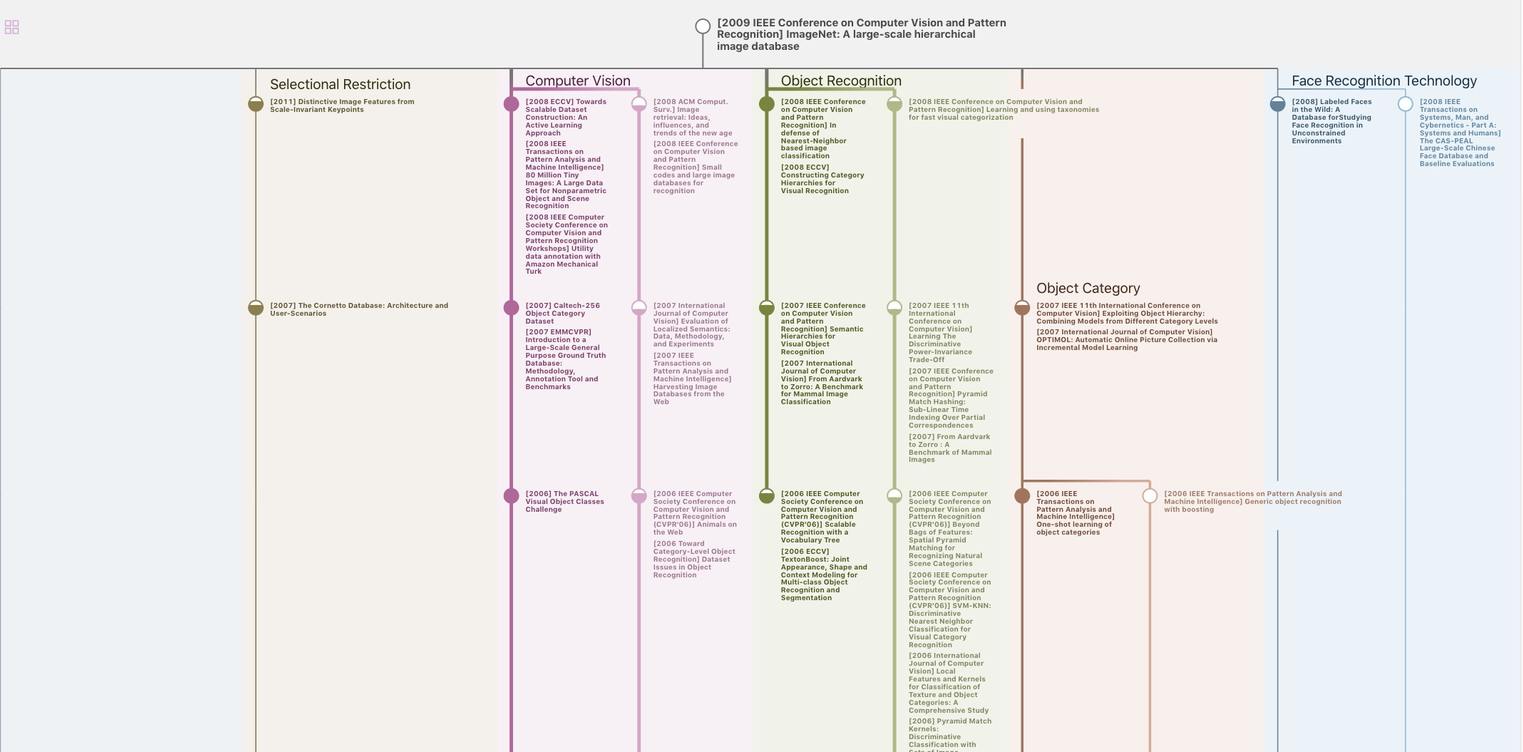
生成溯源树,研究论文发展脉络
Chat Paper
正在生成论文摘要