Graph-based Recommendation for Sparse and Heterogeneous User Interactions
CoRR(2023)
摘要
Recommender system research has oftentimes focused on approaches that operate on large-scale datasets containing millions of user interactions. However, many small businesses struggle to apply state-of-the-art models due to their very limited availability of data. We propose a graph-based recommender model which utilizes heterogeneous interactions between users and content of different types and is able to operate well on small-scale datasets. A genetic algorithm is used to find optimal weights that represent the strength of the relationship between users and content. Experiments on two real-world datasets (which we make available to the research community) show promising results (up to 7% improvement), in comparison with other state-of-the-art methods for low-data environments. These improvements are statistically significant and consistent across different data samples.
更多查看译文
关键词
Personalized page rank, Genetic algorithm, Collaborative filtering
AI 理解论文
溯源树
样例
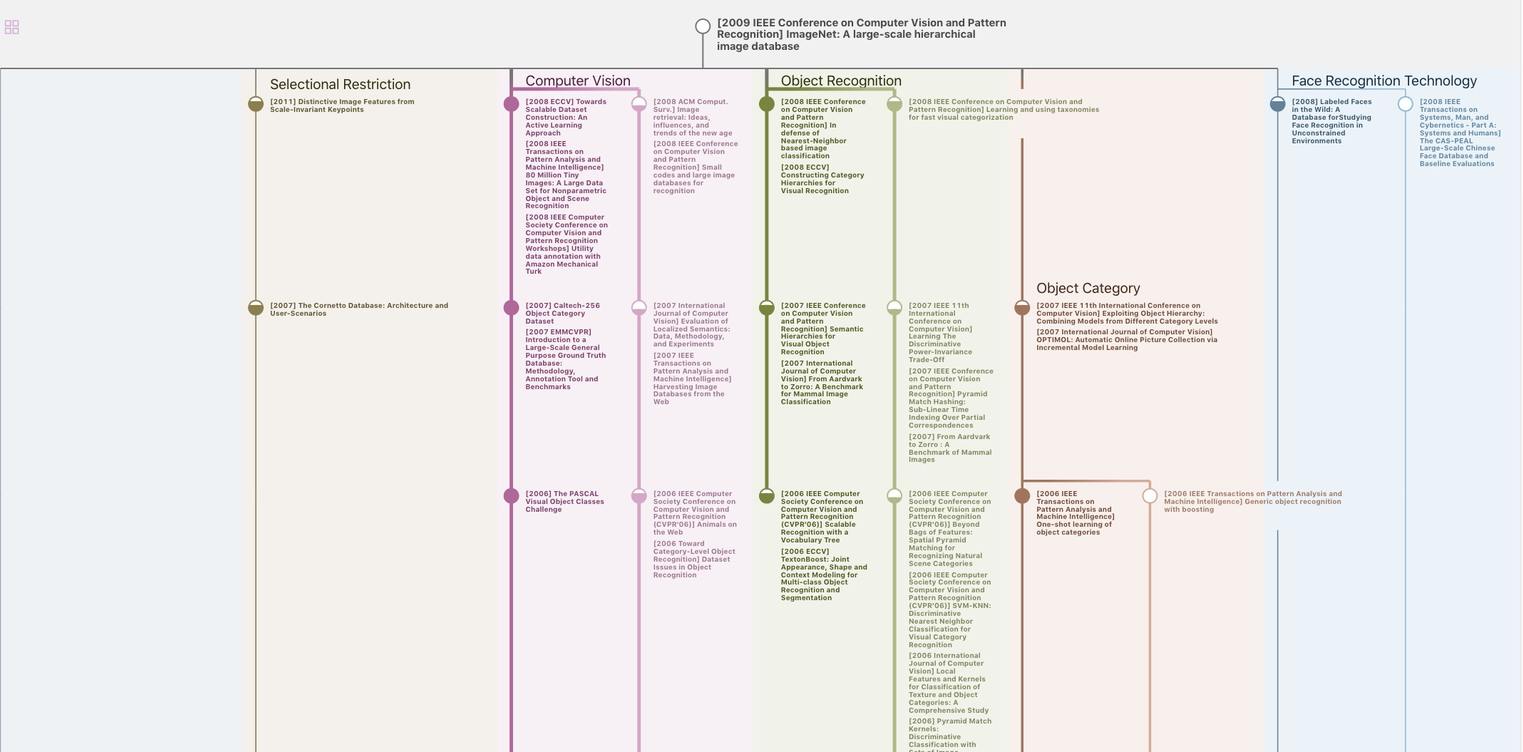
生成溯源树,研究论文发展脉络
Chat Paper
正在生成论文摘要