Evolution of Filter Bubbles and Polarization in News Recommendation
arxiv(2023)
摘要
Recent work in news recommendation has demonstrated that recommenders can over-expose users to articles that support their pre-existing opinions. However, most existing work focuses on a static setting or over a short-time window, leaving open questions about the long-term and dynamic impacts of news recommendations. In this paper, we explore these dynamic impacts through a systematic study of three research questions: 1) How do the news reading behaviors of users change after repeated long-term interactions with recommenders? 2) How do the inherent preferences of users change over time in such a dynamic recommender system? 3) Can the existing SOTA static method alleviate the problem in the dynamic environment? Concretely, we conduct a comprehensive data-driven study through simulation experiments of political polarization in news recommendations based on 40,000 annotated news articles. We find that users are rapidly exposed to more extreme content as the recommender evolves. We also find that a calibration-based intervention can slow down this polarization, but leaves open significant opportunities for future improvements
更多查看译文
关键词
news recommendation,filter bubbles,polarization
AI 理解论文
溯源树
样例
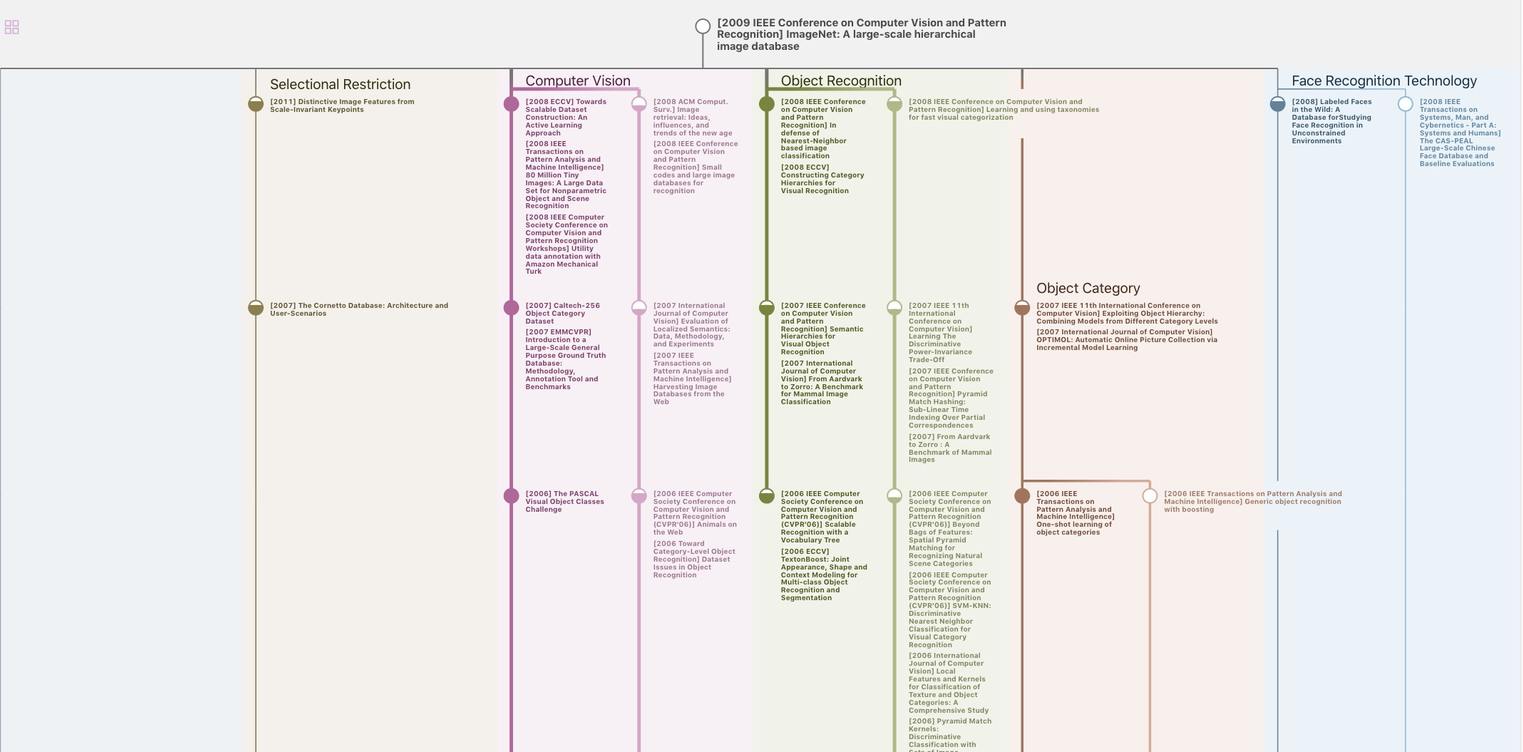
生成溯源树,研究论文发展脉络
Chat Paper
正在生成论文摘要