Qualitative Analysis of a Graph Transformer Approach to Addressing Hate Speech: Adapting to Dynamically Changing Content
arxiv(2023)
摘要
Our work advances an approach for predicting hate speech in social media, drawing out the critical need to consider the discussions that follow a post to successfully detect when hateful discourse may arise. Using graph transformer networks, coupled with modelling attention and BERT-level natural language processing, our approach can capture context and anticipate upcoming anti-social behaviour. In this paper, we offer a detailed qualitative analysis of this solution for hate speech detection in social networks, leading to insights into where the method has the most impressive outcomes in comparison with competitors and identifying scenarios where there are challenges to achieving ideal performance. Included is an exploration of the kinds of posts that permeate social media today, including the use of hateful images. This suggests avenues for extending our model to be more comprehensive. A key insight is that the focus on reasoning about the concept of context positions us well to be able to support multi-modal analysis of online posts. We conclude with a reflection on how the problem we are addressing relates especially well to the theme of dynamic change, a critical concern for all AI solutions for social impact. We also comment briefly on how mental health well-being can be advanced with our work, through curated content attuned to the extent of hate in posts.
更多查看译文
关键词
addressing hate,graph transformer approach,speech
AI 理解论文
溯源树
样例
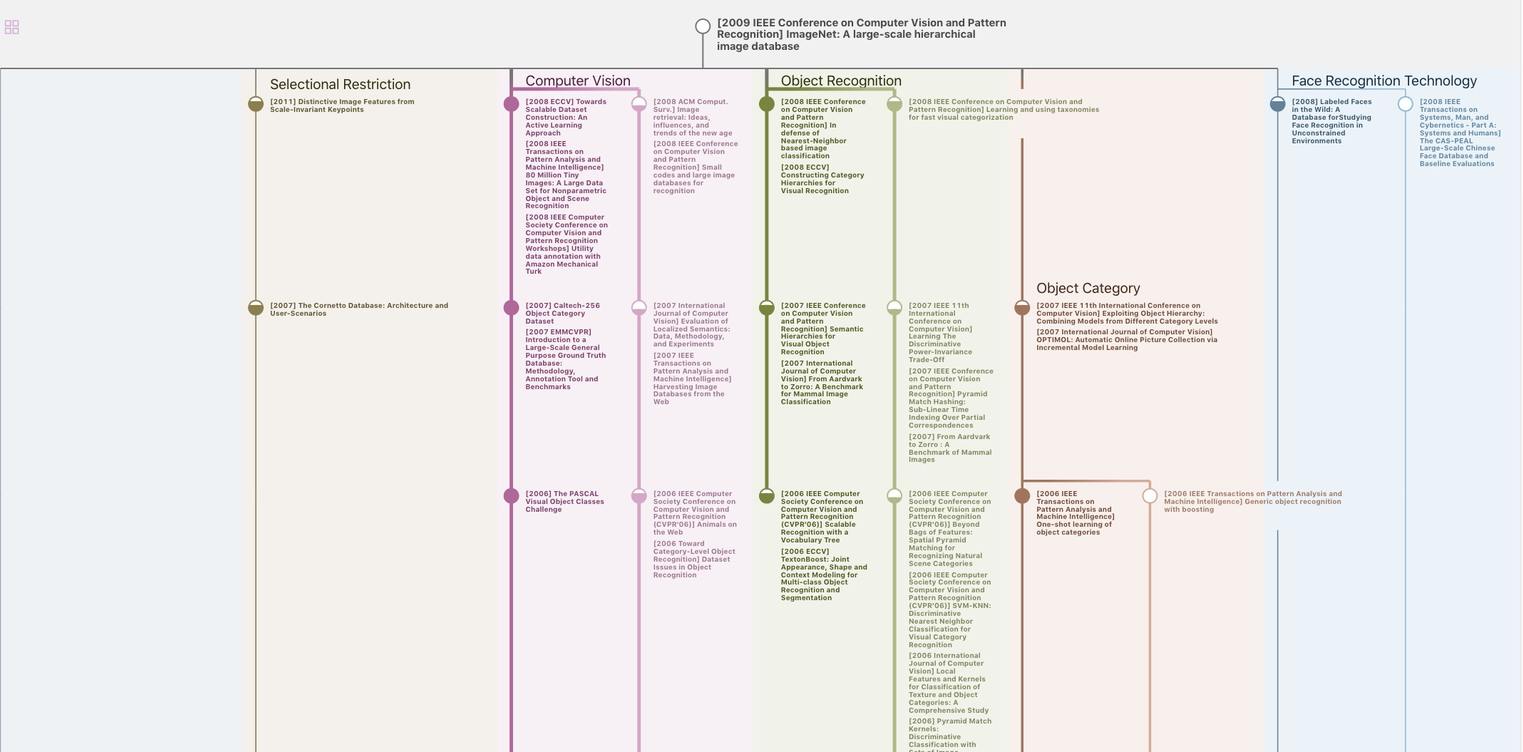
生成溯源树,研究论文发展脉络
Chat Paper
正在生成论文摘要