Towards a Unified Model for Generating Answers and Explanations in Visual Question Answering
arxiv(2023)
摘要
The field of visual question answering (VQA) has recently seen a surge in research focused on providing explanations for predicted answers. However, current systems mostly rely on separate models to predict answers and generate explanations, leading to less grounded and frequently inconsistent results. To address this, we propose a multitask learning approach towards a Unified Model for Answer and Explanation generation (UMAE). Our approach involves the addition of artificial prompt tokens to training data and fine-tuning a multimodal encoder-decoder model on a variety of VQA-related tasks. In our experiments, UMAE models surpass the prior state-of-the-art answer accuracy on A-OKVQA by 10~15%, show competitive results on OK-VQA, achieve new state-of-the-art explanation scores on A-OKVQA and VCR, and demonstrate promising out-of-domain performance on VQA-X.
更多查看译文
关键词
generating answers,explanations,unified model
AI 理解论文
溯源树
样例
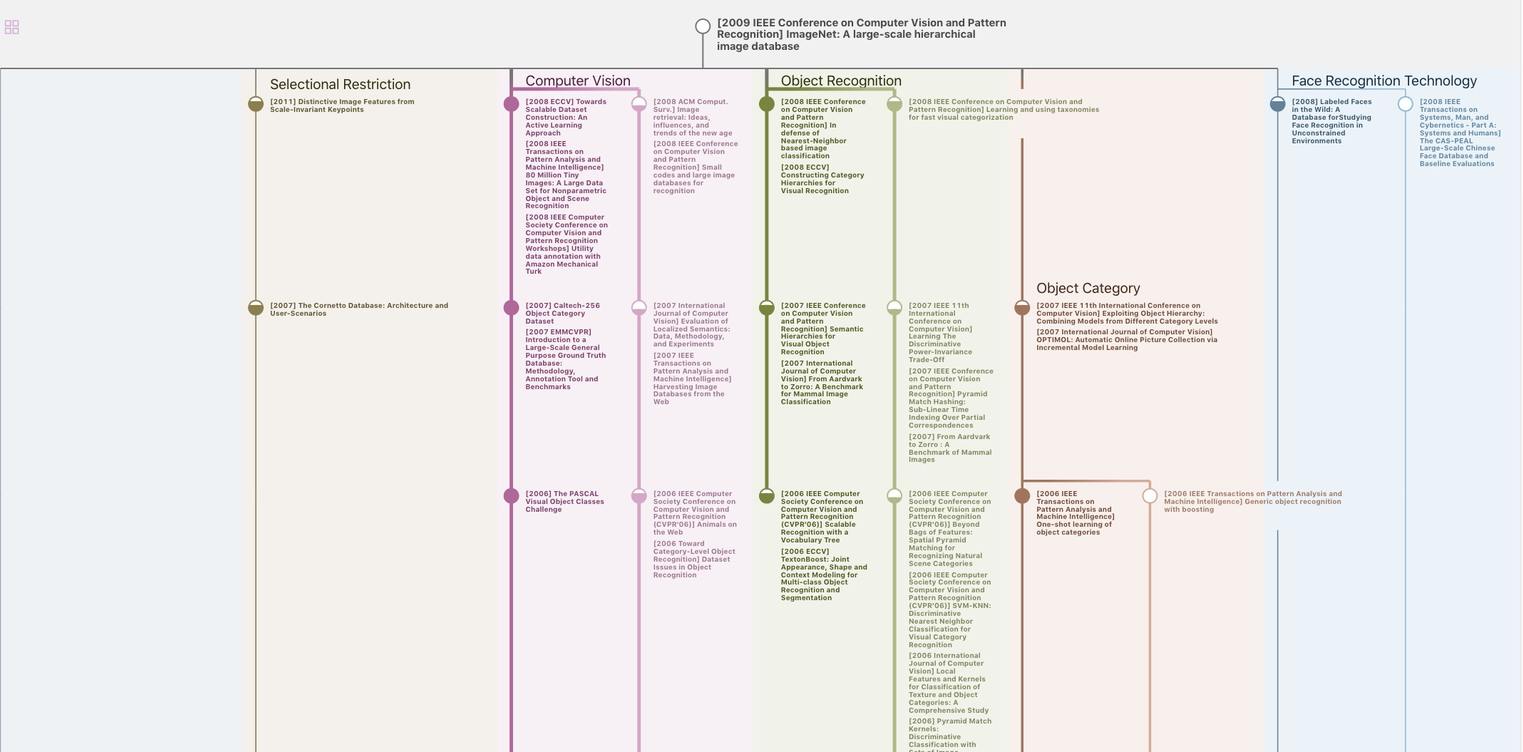
生成溯源树,研究论文发展脉络
Chat Paper
正在生成论文摘要