Modelling Long Range Dependencies in N-D: From Task-Specific to a General Purpose CNN
ICLR 2023(2023)
摘要
Performant Convolutional Neural Network (CNN) architectures must be tailored to specific tasks in order to consider the length, resolution, and dimensionality of the input data. In this work, we tackle the need for problem-specific CNN architectures. We present the Continuous Convolutional Neural Network (CCNN): a single CNN able to process data of arbitrary resolution, dimensionality and length without any structural changes. Its key component are its continuous convolutional kernels which model long-range dependencies at every layer, and thus remove the need of current CNN architectures for task-dependent downsampling and depths. We showcase the generality of our method by using the same architecture for tasks on sequential ($1{\rm D}$), visual ($2{\rm D}$) and point-cloud ($3{\rm D}$) data. Our CCNN matches and often outperforms the current state-of-the-art across all tasks considered.
更多查看译文
关键词
convolutional neural networks,continuous convolutional kernels,CNNs,continuous parameterizations,sequential data,visual data,point-cloud data
AI 理解论文
溯源树
样例
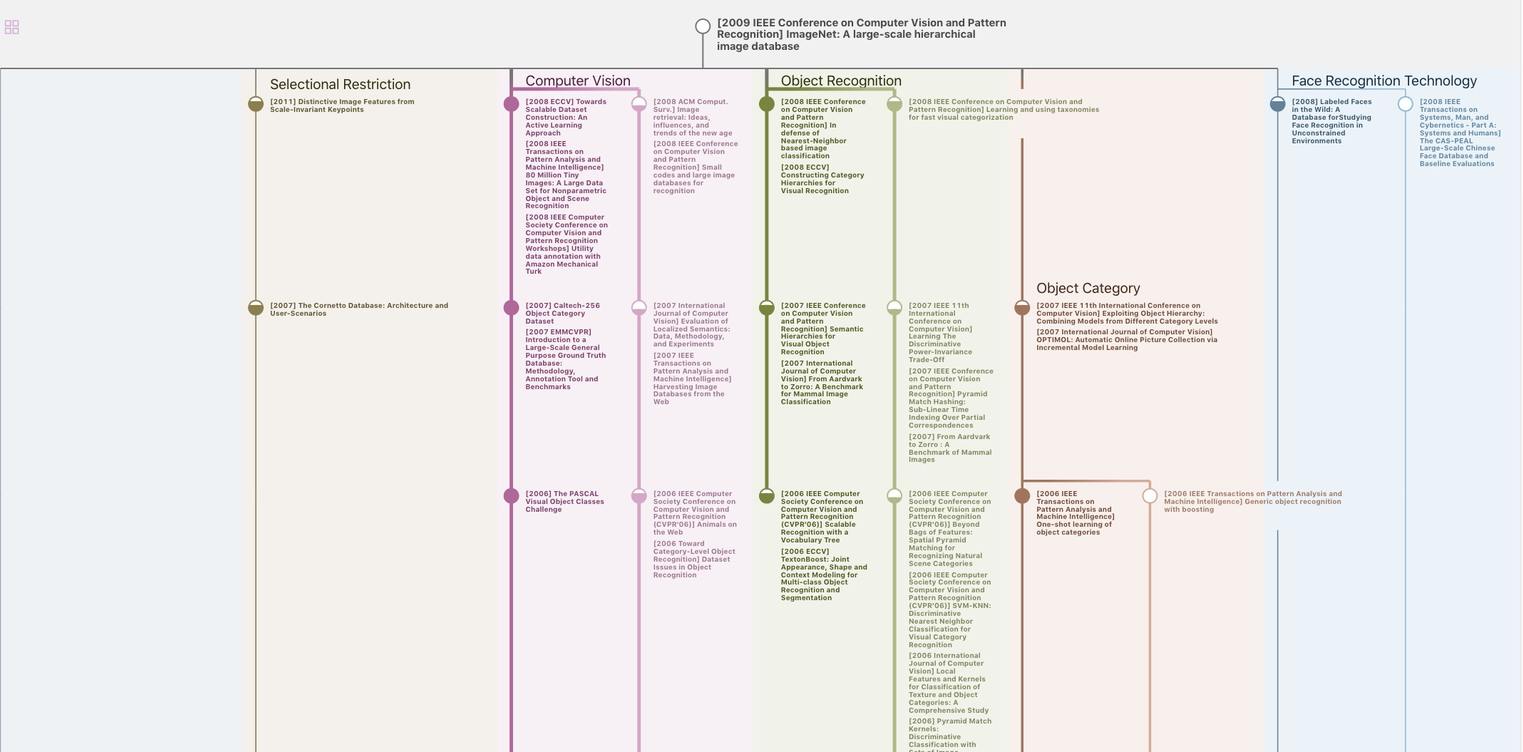
生成溯源树,研究论文发展脉络
Chat Paper
正在生成论文摘要