Learning to Rank Normalized Entropy Curves with Differentiable Window Transformation
arxiv(2023)
摘要
Recent automated machine learning systems often use learning curves ranking models to inform decisions about when to stop unpromising trials and identify better model configurations. In this paper, we present a novel learning curve ranking model specifically tailored for ranking normalized entropy (NE) learning curves, which are commonly used in online advertising and recommendation systems. Our proposed model, self-Adaptive Curve Transformation augmented Relative curve Ranking (ACTR2), features an adaptive curve transformation layer that transforms raw lifetime NE curves into composite window NE curves with the window sizes adaptively optimized based on both the position on the learning curve and the curve's dynamics. We also introduce a novel differentiable indexing method for the proposed adaptive curve transformation, which allows gradients with respect to the discrete indices to flow freely through the curve transformation layer, enabling the learned window sizes to be updated flexibly during training. Additionally, we propose a pairwise curve ranking architecture that directly models the difference between the two learning curves and is better at capturing subtle changes in relative performance that may not be evident when modeling each curve individually as the existing approaches did. Our extensive experiments on a real-world NE curve dataset demonstrate the effectiveness of each key component of ACTR2 and its improved performance over the state-of-the-art.
更多查看译文
关键词
entropy curves,differentiable window
AI 理解论文
溯源树
样例
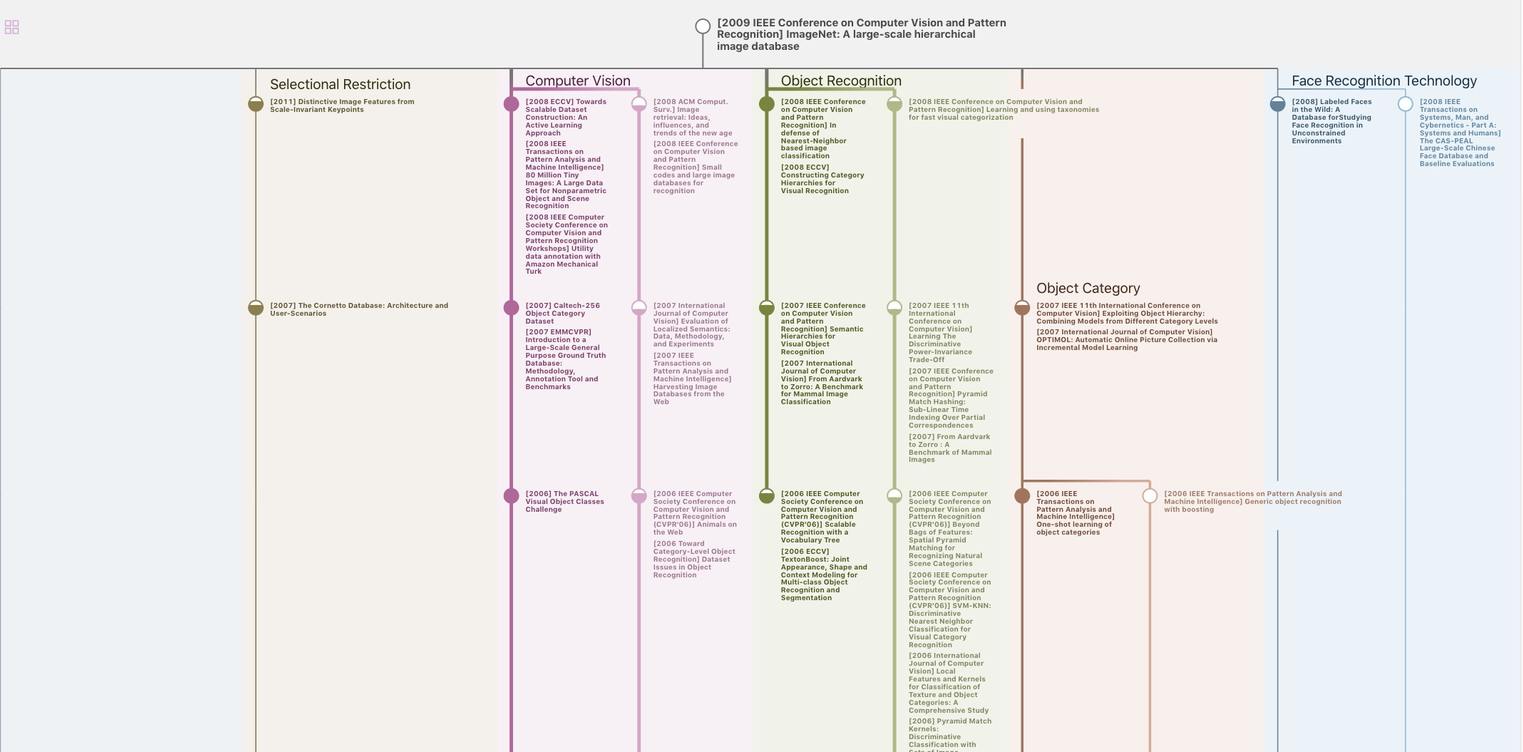
生成溯源树,研究论文发展脉络
Chat Paper
正在生成论文摘要