AutoCost: Evolving Intrinsic Cost for Zero-violation Reinforcement Learning
arxiv(2023)
摘要
Safety is a critical hurdle that limits the application of deep reinforcement learning (RL) to real-world control tasks. To this end, constrained reinforcement learning leverages cost functions to improve safety in constrained Markov decision processes. However, such constrained RL methods fail to achieve zero violation even when the cost limit is zero. This paper analyzes the reason for such failure, which suggests that a proper cost function plays an important role in constrained RL. Inspired by the analysis, we propose AutoCost, a simple yet effective framework that automatically searches for cost functions that help constrained RL to achieve zero-violation performance. We validate the proposed method and the searched cost function on the safe RL benchmark Safety Gym. We compare the performance of augmented agents that use our cost function to provide additive intrinsic costs with baseline agents that use the same policy learners but with only extrinsic costs. Results show that the converged policies with intrinsic costs in all environments achieve zero constraint violation and comparable performance with baselines.
更多查看译文
关键词
intrinsic autocost,learning,zero-violation
AI 理解论文
溯源树
样例
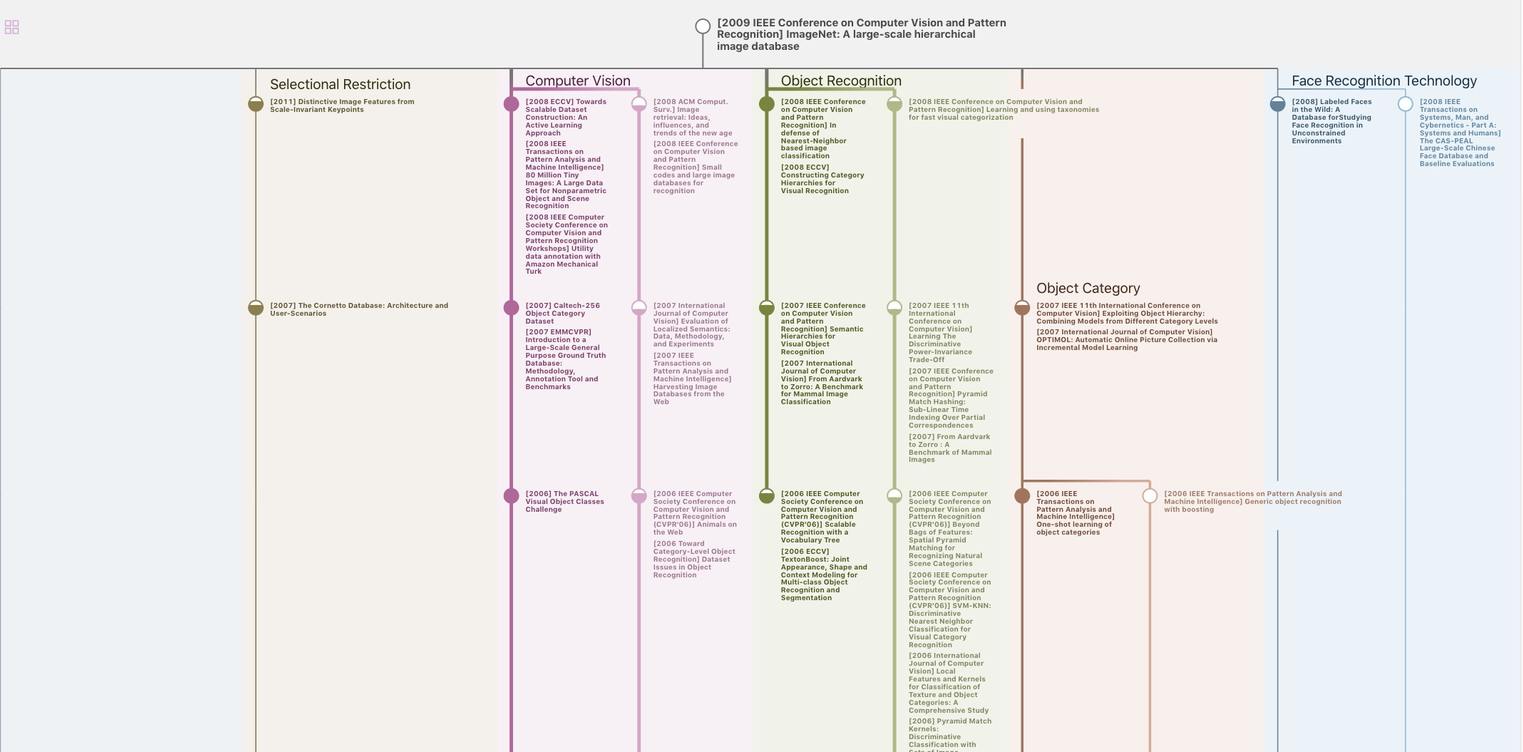
生成溯源树,研究论文发展脉络
Chat Paper
正在生成论文摘要