Conclusion-based Counter-Argument Generation
arxiv(2023)
摘要
In real-world debates, the most common way to counter an argument is to reason against its main point, that is, its conclusion. Existing work on the automatic generation of natural language counter-arguments does not address the relation to the conclusion, possibly because many arguments leave their conclusion implicit. In this paper, we hypothesize that the key to effective counter-argument generation is to explicitly model the argument's conclusion and to ensure that the stance of the generated counter is opposite to that conclusion. In particular, we propose a multitask approach that jointly learns to generate both the conclusion and the counter of an input argument. The approach employs a stance-based ranking component that selects the counter from a diverse set of generated candidates whose stance best opposes the generated conclusion. In both automatic and manual evaluation, we provide evidence that our approach generates more relevant and stance-adhering counters than strong baselines.
更多查看译文
关键词
generation,conclusion-based,counter-argument
AI 理解论文
溯源树
样例
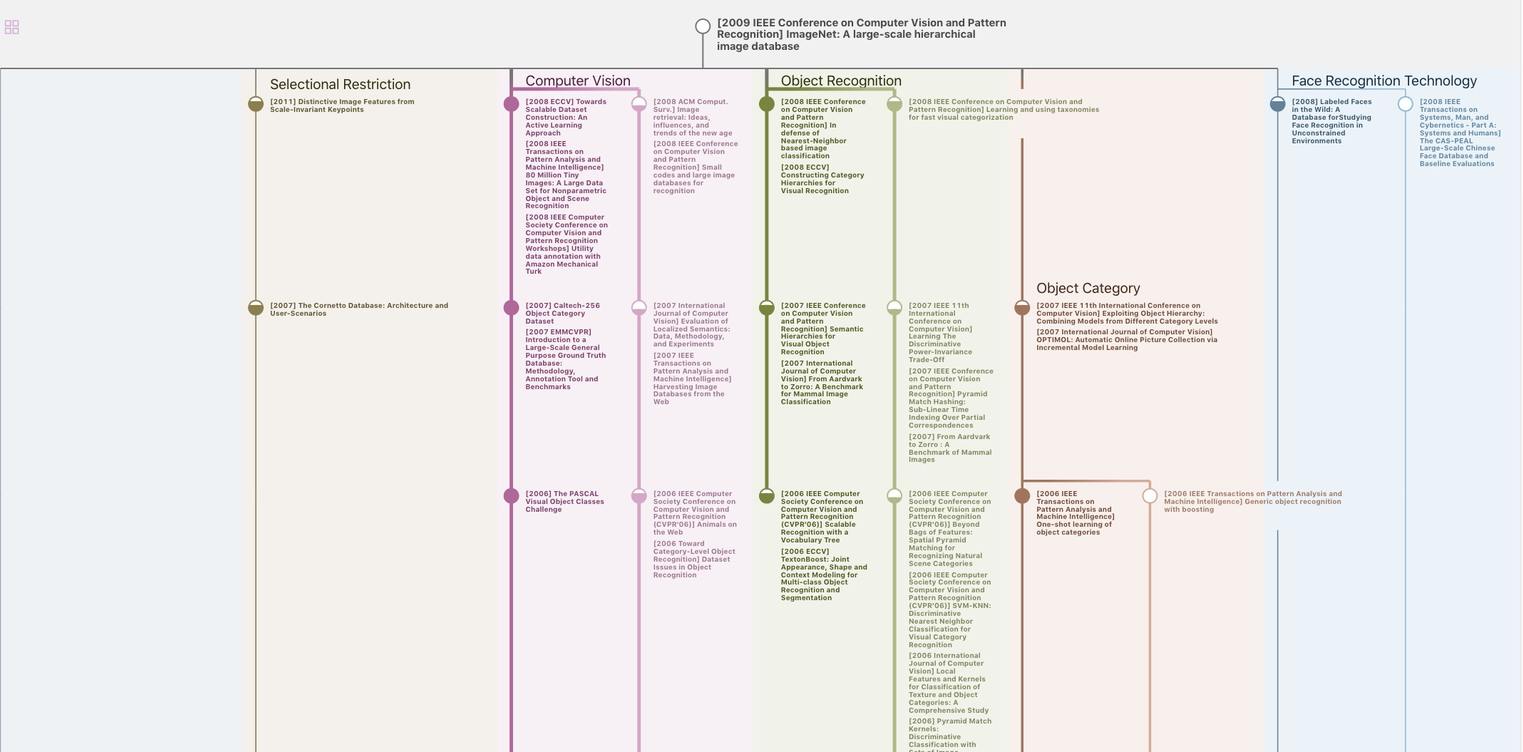
生成溯源树,研究论文发展脉络
Chat Paper
正在生成论文摘要