Gossiped and Quantized Online Multi-Kernel Learning
arxiv(2023)
摘要
In instances of online kernel learning where little prior information is available and centralized learning is unfeasible, past research has shown that distributed and online multi-kernel learning provides sub-linear regret as long as every pair of nodes in the network can communicate (i.e., the communications network is a complete graph). In addition, to manage the communication load, which is often a performance bottleneck, communications between nodes can be quantized. This letter expands on these results to non-fully connected graphs, which is often the case in wireless sensor networks. To address this challenge, we propose a gossip algorithm and provide a proof that it achieves sub-linear regret. Experiments with real datasets confirm our findings.
更多查看译文
关键词
Federated learning,gossip algorithms,kernel-based learning,quantization,sensor networks
AI 理解论文
溯源树
样例
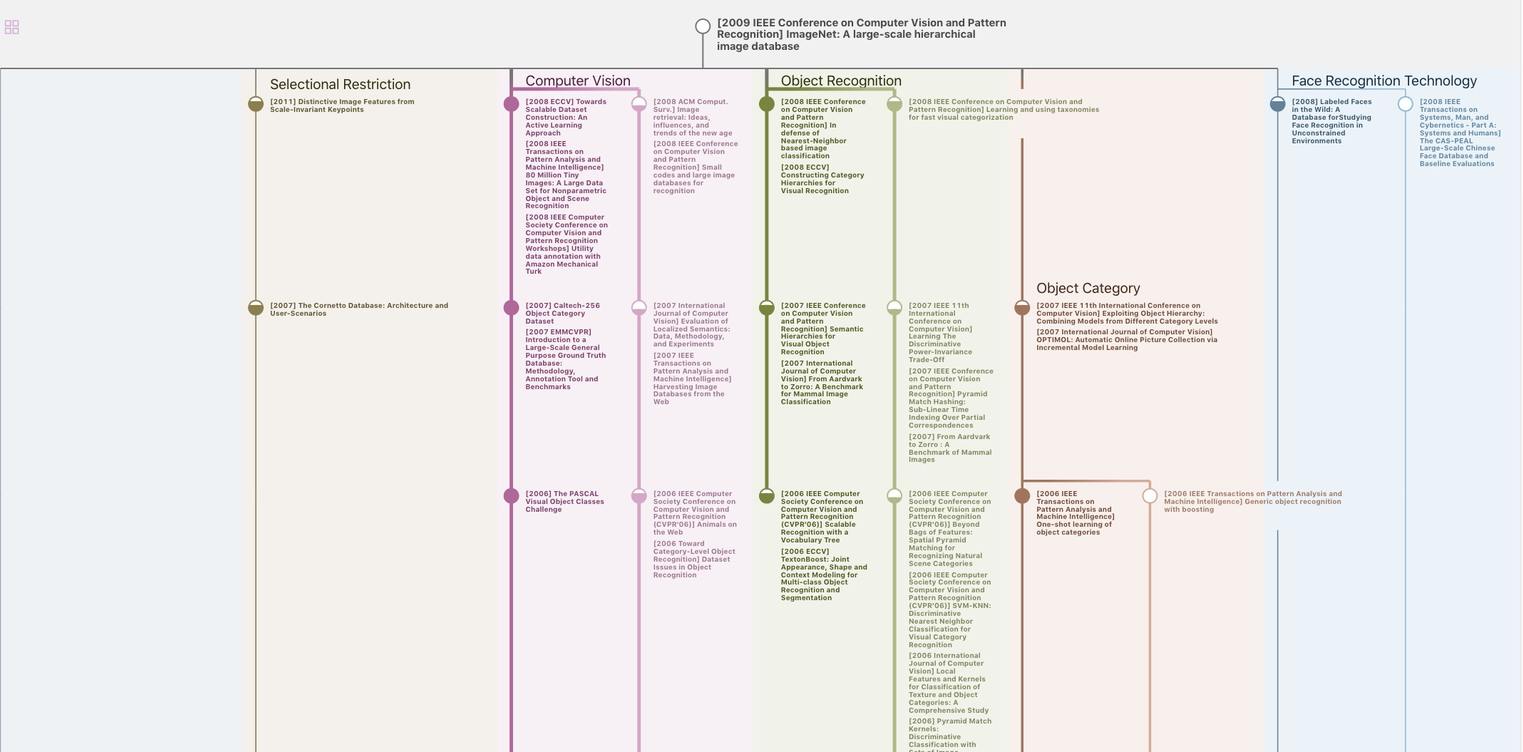
生成溯源树,研究论文发展脉络
Chat Paper
正在生成论文摘要