Expectile hidden Markov regression models for analyzing cryptocurrency returns
Statistics and Computing(2024)
摘要
In this paper we develop a linear expectile hidden Markov model for the analysis of cryptocurrency time series in a risk management framework. The methodology proposed allows to focus on extreme returns and describe their temporal evolution by introducing in the model time-dependent coefficients evolving according to a latent discrete homogeneous Markov chain. As it is often used in the expectile literature, estimation of the model parameters is based on the asymmetric normal distribution. Maximum likelihood estimates are obtained via an Expectation–Maximization algorithm using efficient M-step update formulas for all parameters. We evaluate the introduced method with both artificial data under several experimental settings and real data investigating the relationship between daily Bitcoin returns and major world market indices.
更多查看译文
关键词
Asymmetric normal distribution,Cryptocurrencies,EM algorithm,Expectile regression,Markov switching models,Time series
AI 理解论文
溯源树
样例
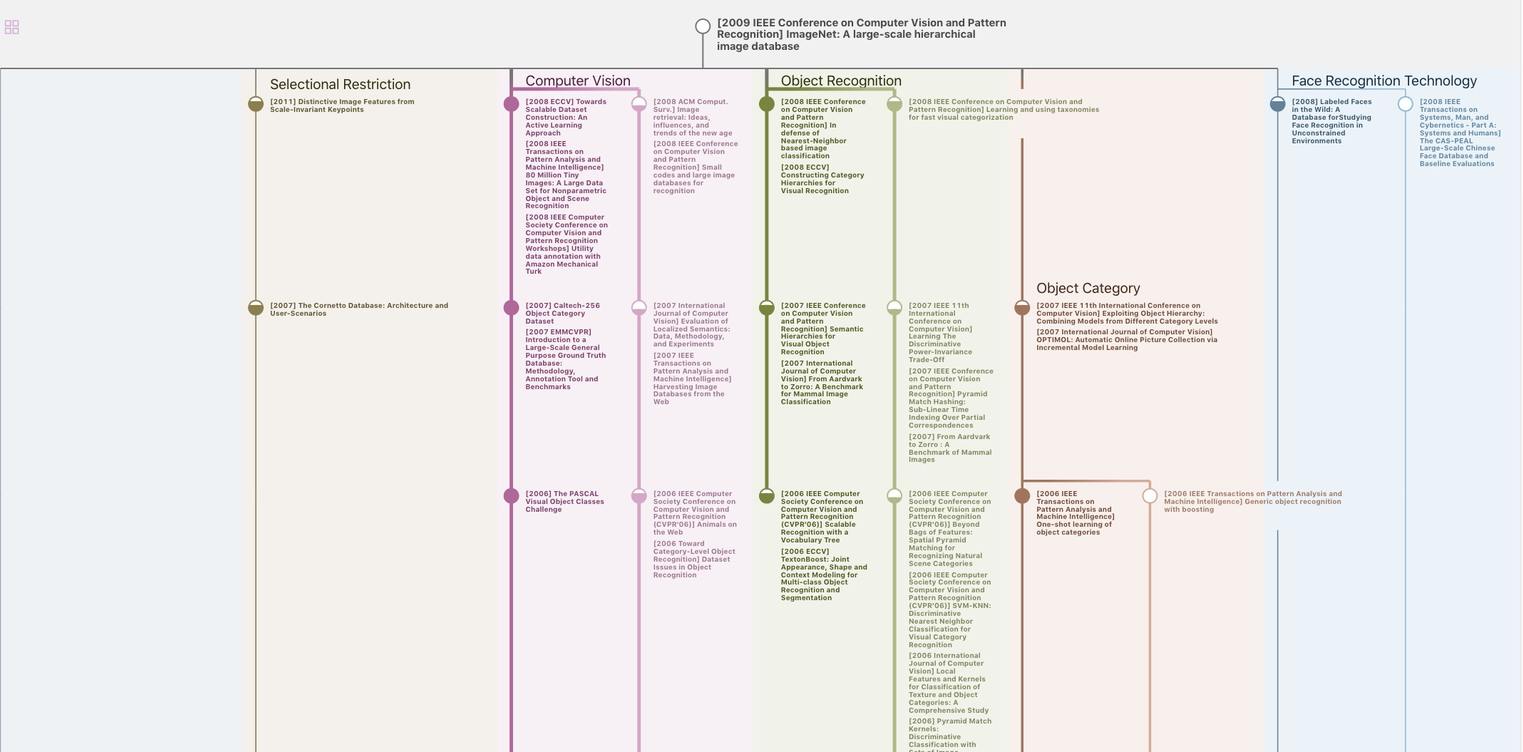
生成溯源树,研究论文发展脉络
Chat Paper
正在生成论文摘要