Ground-level gaseous pollutants (NO2, SO2, and CO) in China: daily seamless mapping and spatiotemporal variations
Atmospheric Chemistry and Physics(2023)
摘要
Gaseous pollutants at the ground level seriously threaten the urban air quality environment and public health. There are few estimates of gaseous pollutants that are spatially and temporally resolved and continuous across China. This study takes advantage of big data and artificial-intelligence technologies to generate seamless daily maps of three major ambient pollutant gases, i.e., NO2, SO2, and CO, across China from 2013 to 2020 at a uniform spatial resolution of 10 km. Cross-validation between our estimates and ground observations illustrated a high data quality on a daily basis for surface NO2, SO2, and CO concentrations, with mean coefficients of determination (root-mean-square errors) of 0.84 (7.99 mu g m(-3)), 0.84 (10.7 mu g m(-3)), and 0.80 (0.29 mg m(-3)), respectively. We found that the COVID-19 lockdown had sustained impacts on gaseous pollutants, where surface CO recovered to its normal level in China on around the 34th day after the Lunar New Year, while surface SO2 and NO2 rebounded more than 2 times slower due to more CO emissions from residents' increased indoor cooking and atmospheric oxidation capacity. Surface NO2, SO2, and CO reached their peak annual concentrations of 21.3 +/- 8.8 mu g m(-3), 23.1 +/- 13.3 mu g m(-3), and 1.01 +/- 0.29 mg m(-3) in 2013, then continuously declined over time by 12 %, 55 %, and 17 %, respectively, until 2020. The declining rates were more prominent from 2013 to 2017 due to the sharper reductions in anthropogenic emissions but have slowed down in recent years. Nevertheless, people still suffer from high-frequency risk exposure to surface NO2 in eastern China, while surface SO2 and CO have almost reached the World Health Organization (WHO) recommended short-term air quality guidelines (AQG) level since 2018, benefiting from the implemented stricter ultra-low emission standards. This reconstructed dataset of surface gaseous pollutants will benefit future (especially short-term) air pollution and environmental health-related studies.
更多查看译文
关键词
gaseous pollutants,daily seamless mapping,ground-level
AI 理解论文
溯源树
样例
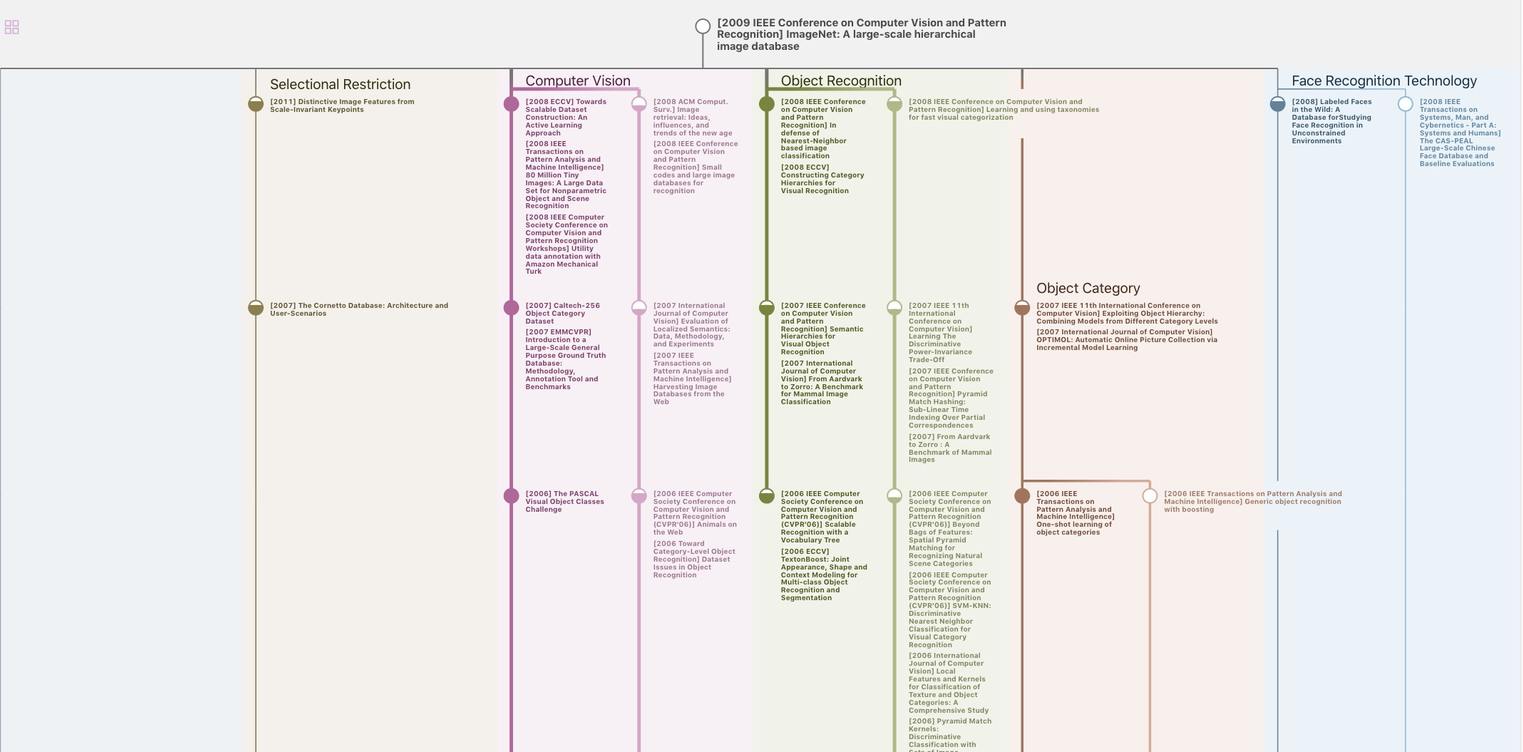
生成溯源树,研究论文发展脉络
Chat Paper
正在生成论文摘要