Modelling, mapping and monitoring of forest cover changes, using support vector machine, kernel logistic regression and naive bayes tree models with optical remote sensing data.
Heliyon(2023)
摘要
The present study is designed to monitor the spatio-temporal changes in forest cover using Remote Sensing (RS) and Geographic Information system (GIS) techniques from 1990 to 2017. Landsat data from 1990 (Thematic mapper [TM]), 2000 and 2010 (Enhanced Thematic Mapper [ETM+]), and 2013 to 2017 (Operational Land Imager/Thermal Infrared Sensor [OLI/TIRS]) were classified into the classes termed snow, water, barren land, built-up area, forest, and vegetation. The method was built using multitemporal Landsat images and the machine learning techniques Support Vector Machine (SVM), Naive Bayes Tree (NBT) and Kernel Logistic Regression (KLR). According to the results, forest area was decreased from 19,360 km (26.0%) to 18,784 km (25.2%) from 1990 to 2010, while forest area was increased from 18,640 km (25.0%) to 26,765 km (35.9%) area from 2013 to 2017 due to "One billion tree Project". According to our findings, SVM performed better than KLR and NBT on all three accuracy metrics (recall, precision, and accuracy) and the F1 score was >0.89. The study demonstrated that concurrent reforestation in barren land areas improved methods of sustaining the forest and RS and GIS into everyday forestry organization practices in Khyber Pakhtun Khwa (KPK), Pakistan. The study results were beneficial, especially at the decision-making level for the local or provincial government of KPK and for understanding the global scenario for regional planning.
更多查看译文
关键词
Change detection,Forest cover,KLR, Kernel Logistic Regression,Kernel logistic regression,LULC, Landuse land cover,Landsat,NBT, Naive Bayes Tree,Naive bayes tree,SVM, Support Vector Machine,Support vector machine
AI 理解论文
溯源树
样例
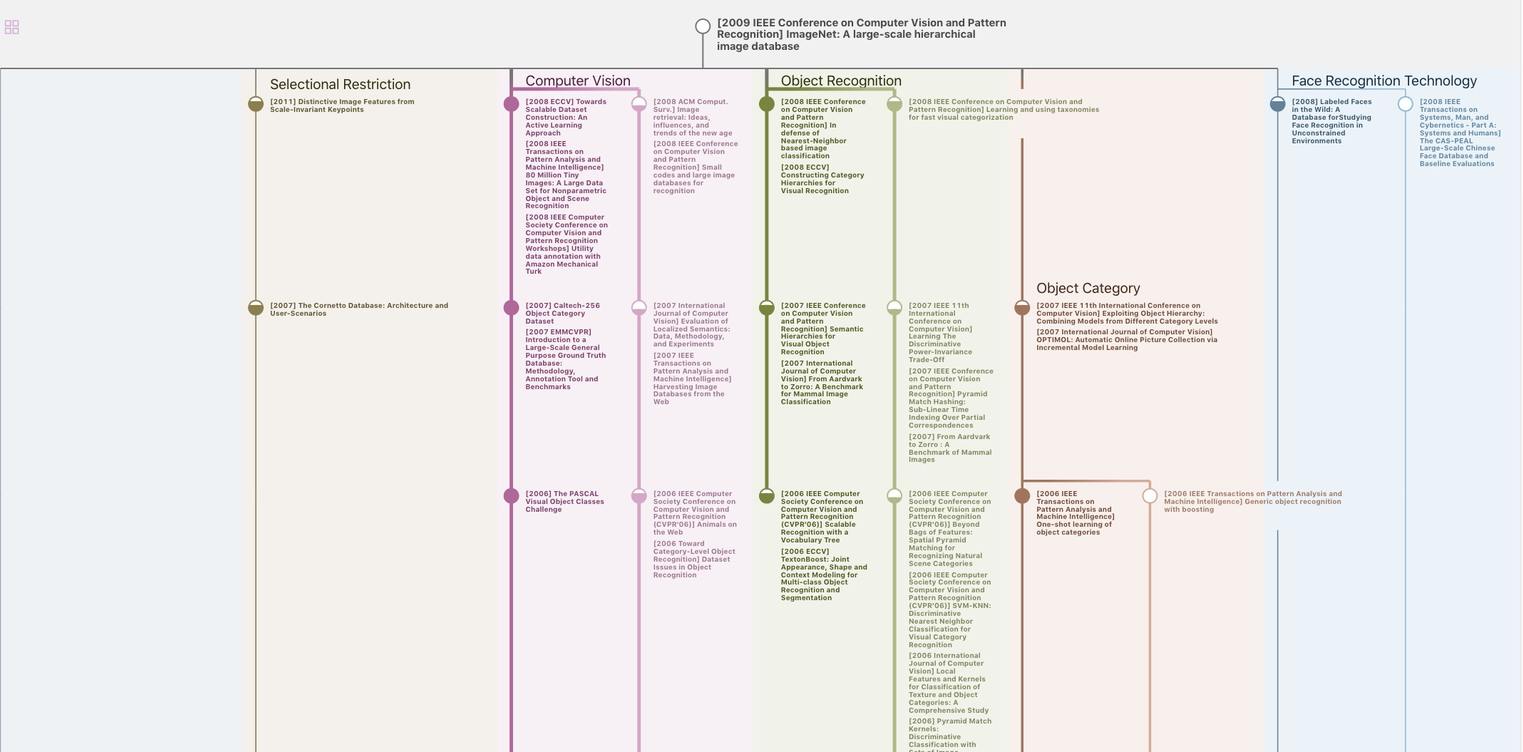
生成溯源树,研究论文发展脉络
Chat Paper
正在生成论文摘要