Deep learning segmentation to analyze bubble dynamics and heat transfer during boiling at various pressures
International Journal of Multiphase Flow(2023)
摘要
Today, neural networks have increasingly gained the attention of researchers and become an effective instrument for a wide range of scientific applications, including issues related to the boiling. However, there are no universal tools in the literature that would allow detecting the life cycle of individual vapor bubbles and automatically measure a wide range of main boiling characteristics based on the high-speed visualization data.In this study, the U-net and Mask R-CNN convolutional neural networks were used to detect and segment bubbles obtained by visualization from the bottom side of a transparent heater during water boiling at various subatmospheric pressures. The key feature of the trained CNN architectures is the ability to detect bubbles located on a heated wall, while ignoring the bubbles that lift-off, and to determine the moment of their de-parture. The verification of various neural networks demonstrated that the Mask R-CNN architecture is more preferable to measure dynamic boiling characteristics.Through trained convolutional neural networks, a wide array of data on local boiling characteristics, including the nucleation site density, bubbles growth rate, life-time and departure diameters, waiting time between mo-ments of bubbles departure and nucleation frequencies were automatically obtained for water boiling at various heat fluxes and pressures in the range of 42-103 kPa. Based on the parameters obtained, heat transfer simulation was carried out using various heat flux partitioning approaches and the ranges of their applicability were demonstrated.
更多查看译文
关键词
Boiling,Bubble dynamics,Heat transfer,Subatmospheric pressures,Optical diagnostics,Neural networks
AI 理解论文
溯源树
样例
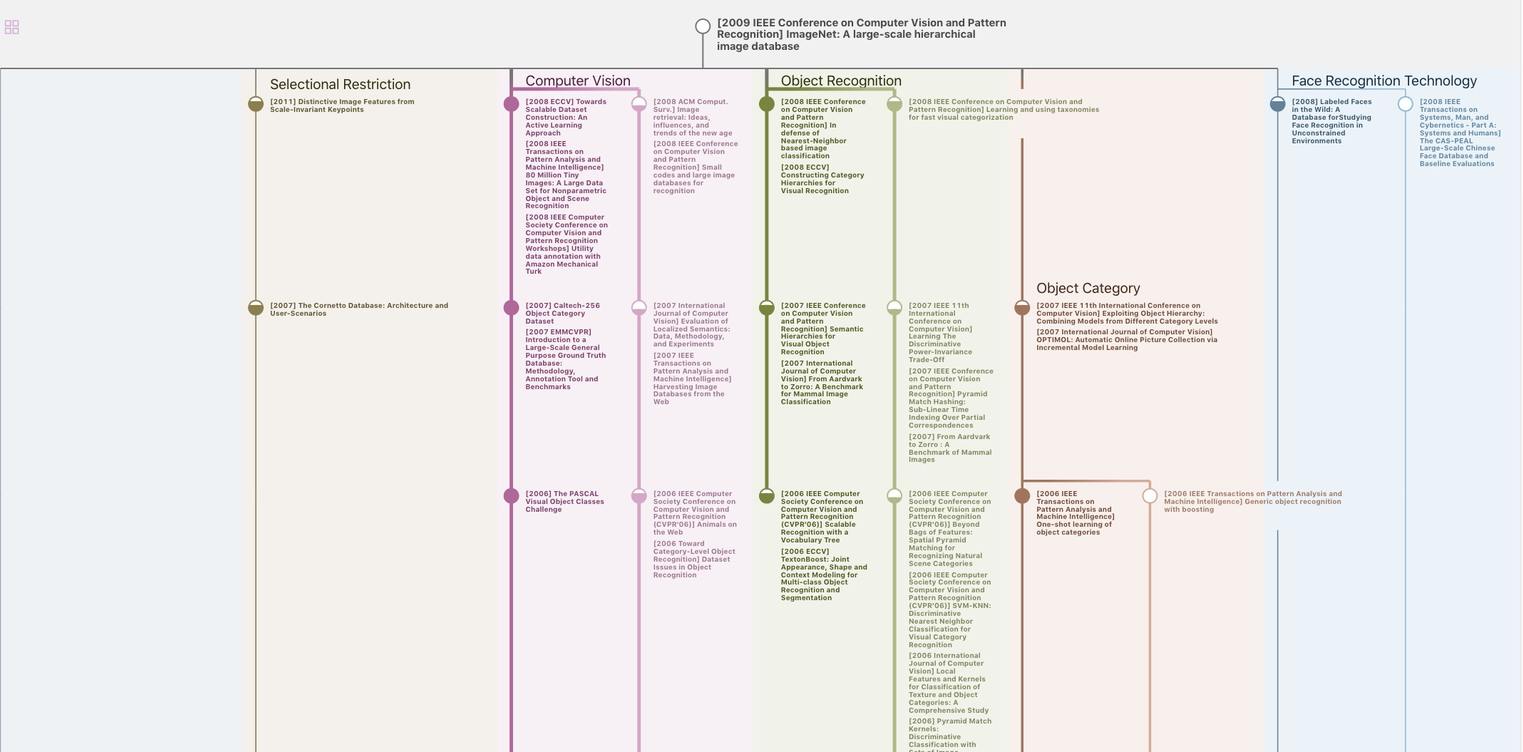
生成溯源树,研究论文发展脉络
Chat Paper
正在生成论文摘要