A Machine Learning Method for Predicting Corrosion Weight Gain of Uranium and Uranium Alloys.
Materials (Basel, Switzerland)(2023)
摘要
As an irreplaceable structural and functional material in strategic equipment, uranium and uranium alloys are generally susceptible to corrosion reactions during service, and predicting corrosion behavior has important research significance. There have been substantial studies conducted on metal corrosion research. Accelerated experiments can shorten the test time, but there are still differences in real corrosion processes. Numerical simulation methods can avoid radioactive experiments, but it is difficult to fully simulate a real corrosion environment. The modeling of real corrosion data using machine learning methods allows for effective corrosion prediction. This research used machine learning methods to study the corrosion of uranium and uranium alloys in air and established a corrosion weight gain prediction model. Eleven classic machine learning algorithms for regression were compared and a ten-fold cross validation method was used to choose the highest accuracy algorithm, which was the extra trees algorithm. Feature selection methods, including the extra trees and Pearson correlation analysis methods, were used to select the most important four factors in corrosion weight gain. As a result, the prediction accuracy of the corrosion weight gain prediction model was 96.8%, which could determine a good prediction of corrosion for uranium and uranium alloys.
更多查看译文
关键词
corrosion,extra trees,feature selection,machine learning,uranium,uranium alloy
AI 理解论文
溯源树
样例
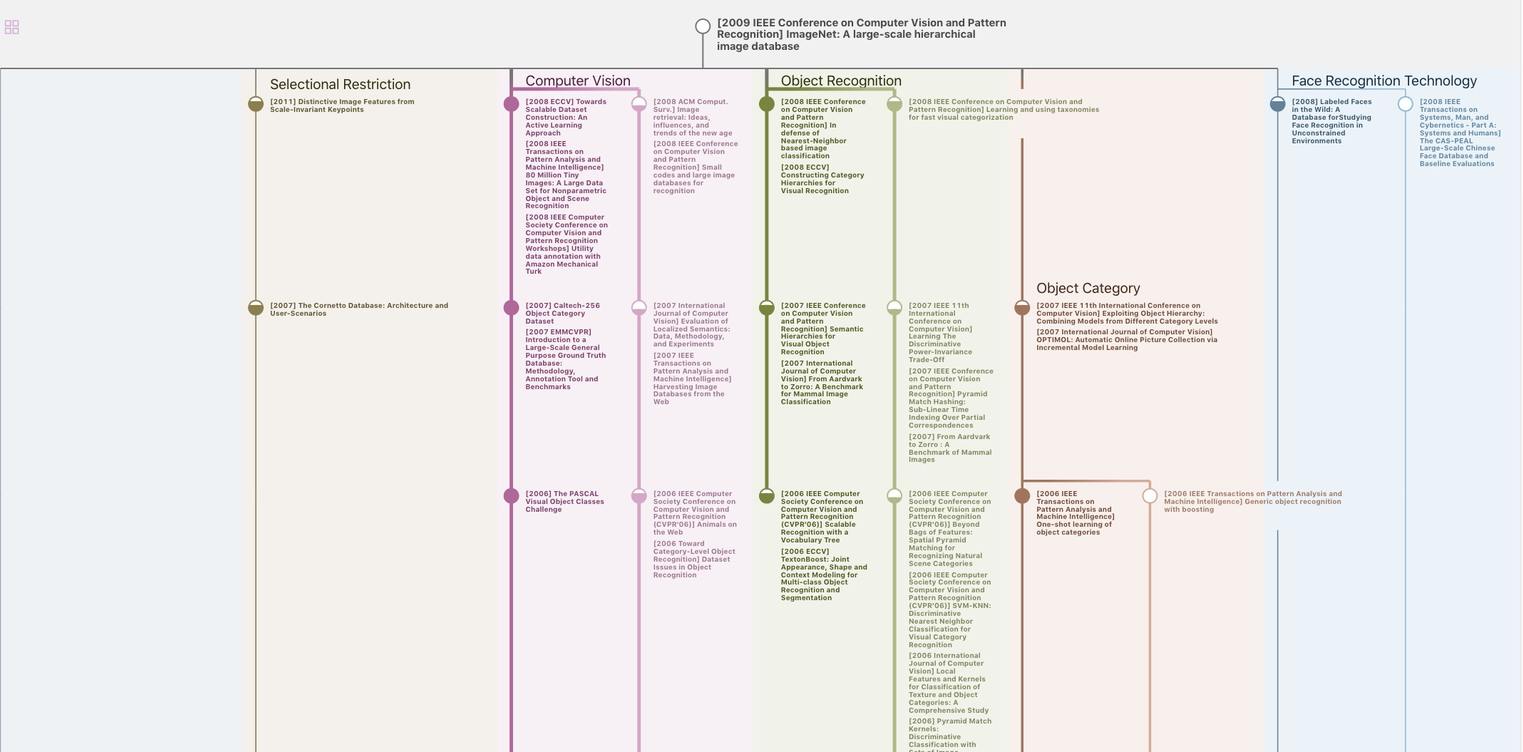
生成溯源树,研究论文发展脉络
Chat Paper
正在生成论文摘要