Variational Approach for Joint Kidney Segmentation and Registration from DCE-MRI Using Fuzzy Clustering with Shape Priors.
Biomedicines(2022)
摘要
The dynamic contrast-enhanced magnetic resonance imaging (DCE-MRI) technique has great potential in the diagnosis, therapy, and follow-up of patients with chronic kidney disease (CKD). Towards that end, precise kidney segmentation from DCE-MRI data becomes a prerequisite processing step. Exploiting the useful information about the kidney's shape in this step mandates a registration operation beforehand to relate the shape model coordinates to those of the image to be segmented. Imprecise alignment of the shape model induces errors in the segmentation results. In this paper, we propose a new variational formulation to jointly segment and register DCE-MRI kidney images based on fuzzy c-means clustering embedded within a level-set (LSet) method. The image pixels' fuzzy memberships and the spatial registration parameters are simultaneously updated in each evolution step to direct the LSet contour toward the target kidney. Results on real medical datasets of 45 subjects demonstrate the superior performance of the proposed approach, reporting a Dice similarity coefficient of 0.94 ± 0.03, Intersection-over-Union of 0.89 ± 0.05, and 2.2 ± 2.3 in 95-percentile of Hausdorff distance. Extensive experiments show that our approach outperforms several state-of-the-art LSet-based methods as well as two UNet-based deep neural models trained for the same task in terms of accuracy and consistency.
更多查看译文
关键词
DCE-MRI,U-Net,chronic kidney disease,fuzzy c-means,kidney registration,kidney segmentation,level set
AI 理解论文
溯源树
样例
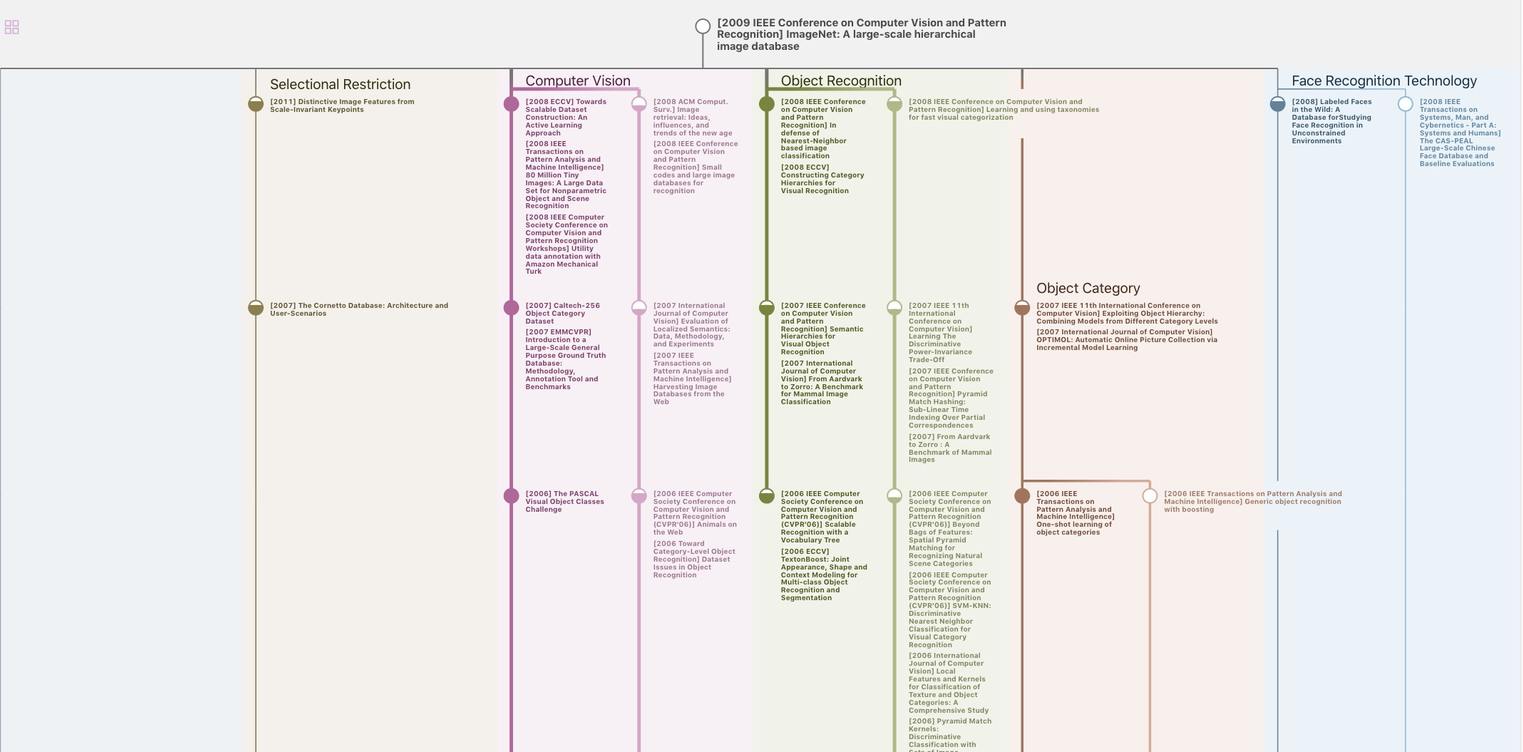
生成溯源树,研究论文发展脉络
Chat Paper
正在生成论文摘要