Low-precision post-filtering in video coding
2022 IEEE International Symposium on Multimedia (ISM)(2022)
摘要
Neural Networks (NNs) have demonstrated their effectiveness in tackling challenges involving multimedia content. In the video coding field, NNs are actively exploited as novel tools that complement conventional signal processing tools, as well as end-to-end coding solutions. Since NNs use commonly floating-point arithmetic, different results may be generated in different computing environments, leading to discrepancies and even corrupted reconstructions. Accordingly, this issue is solved by employing fixed-point arithmetic instead. This paper studies the quantisation of a 32-bit floating-point (float32) NN post-filter to 32-bit fixed-point (int32) and 16-bit fixed-point (int16). On top of the VVC Test Model (VTM) 11.0 NN-based Video Coding (NNVC) 1.0, the coding gains of the float32 post-filter are 5.01% (Y), 18.95% (Cb) and 17.33% Cr. Compared to the float32 inference, the quantised models produce coding losses: 0.01% (Y, Cb and Cr) for the int32 inference and 0.45% (Y), 1.97% (Cb) and 1.19% (Cr) for the int16 inference. Nevertheless, the fixed-point approaches achieve bit exact matches in different computing environments. Moreover, the decoding time with int16 is about half the decoding time of int32.
更多查看译文
关键词
Neural network,post-filtering,quantisation,video coding
AI 理解论文
溯源树
样例
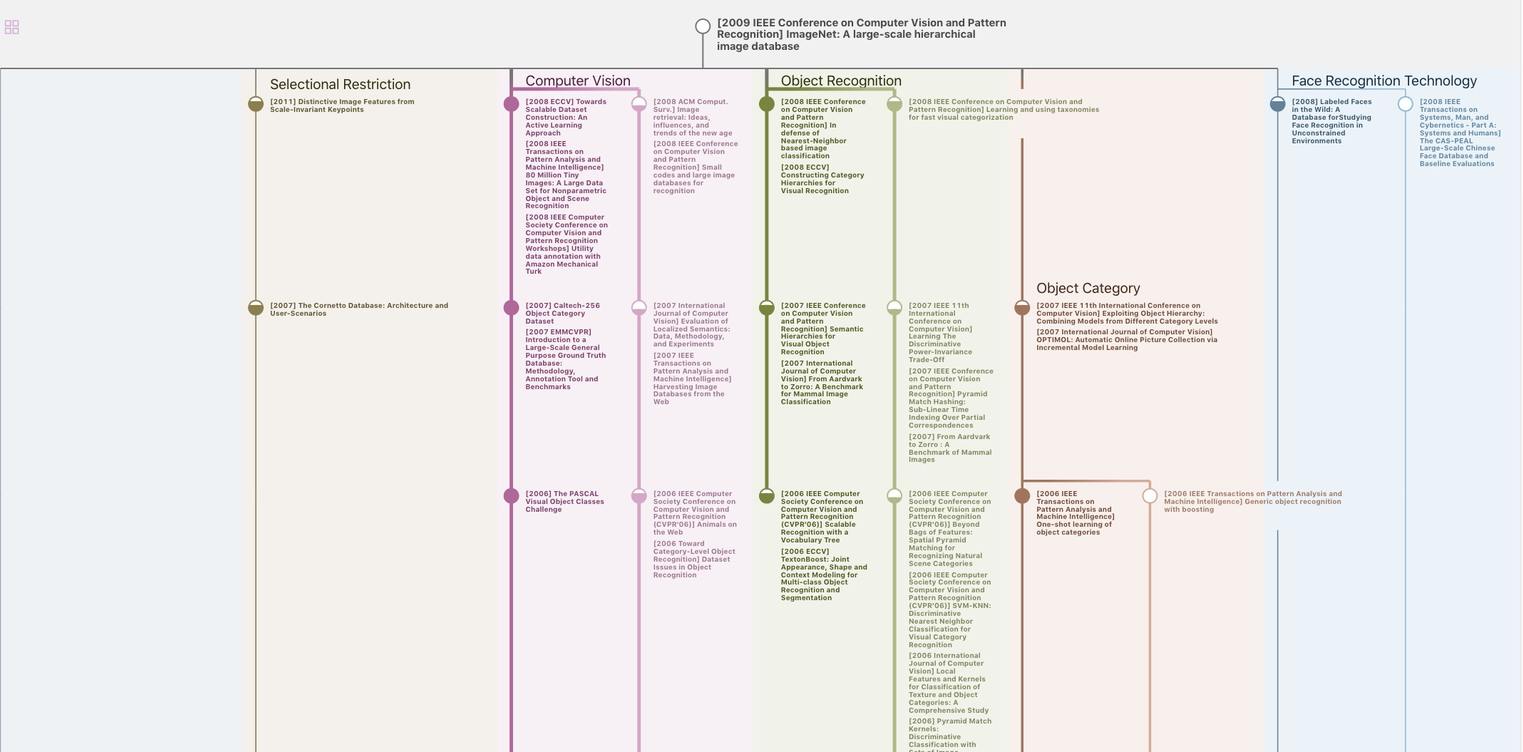
生成溯源树,研究论文发展脉络
Chat Paper
正在生成论文摘要