High-Resolution Shape Deformation Prediction in Additive Manufacturing Using 3D CNN
2022 Winter Simulation Conference (WSC)(2022)
摘要
Additive manufacturing (AM) processes usually have lower geometric quality and when compared to subtractive manufacturing processes. However, AM processes are seeing more use in the industry because they are both affordable and flexible. To address the lower geometric quality and reduced reliability drawbacks, 3D Convolutional Neural Networks (CNN) were developed and used to predict the deformations from the ideal sliced 3D object. The developed 3D CNN were tested on a live dataset consisting of 50 3D printed, 3D scanned, and aligned objects. The linear spatial resolution of these predictions is improved to 150μm with a sampling frequency of 166 units per inch compared to the standard peak resolution of 64 units across an axis. Results indicate that using the described approach provides better predictors of part geometry than the original STereoLithography (STL) file defining the part. An average increase of the F1 measure is 0.0644 over using the STL.
更多查看译文
AI 理解论文
溯源树
样例
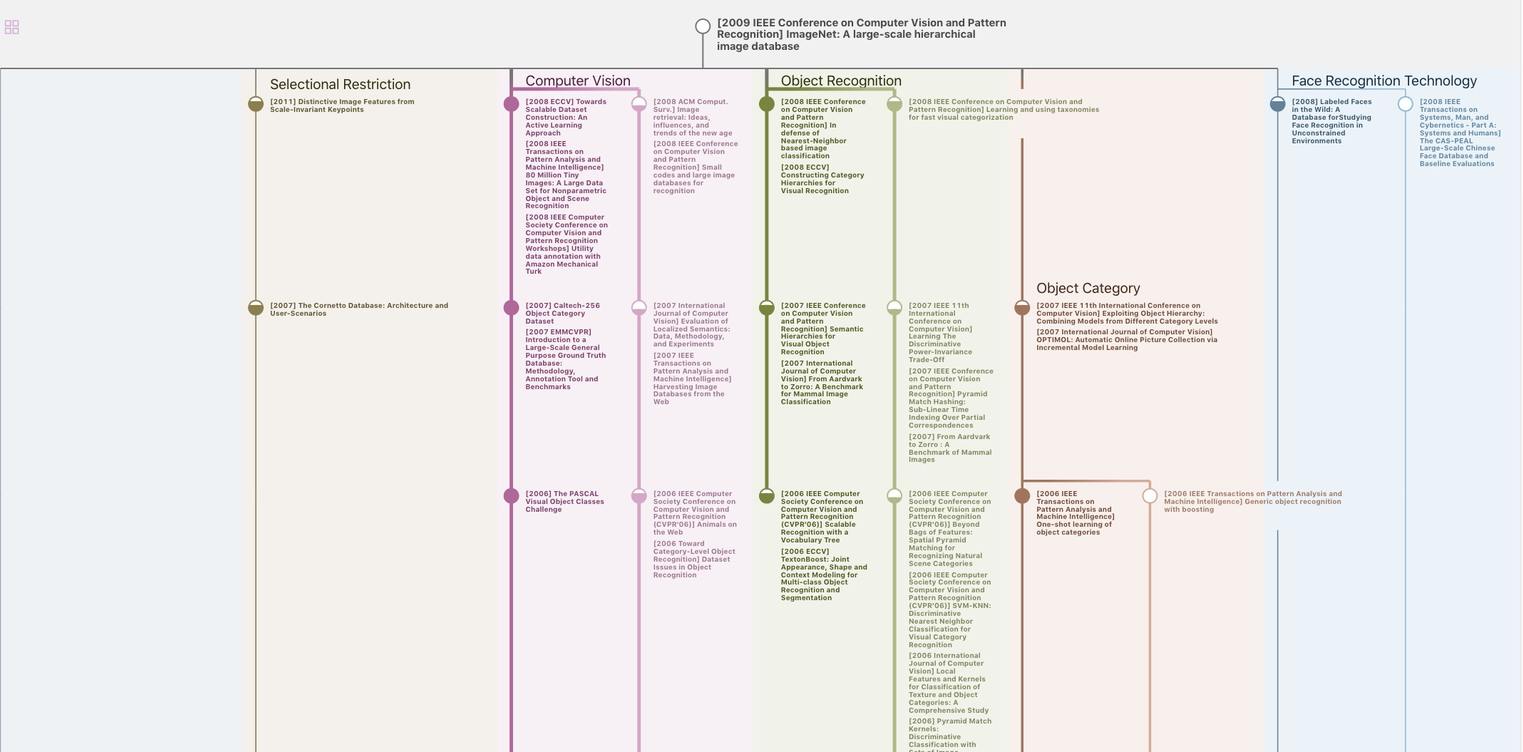
生成溯源树,研究论文发展脉络
Chat Paper
正在生成论文摘要