Improved Imagery Throughput via Cascaded Uncertainty Pruning on U-Net++.
NLDL(2023)
摘要
The extensive use of machine learning inferences in real-life earth observation and remote sensing cases has grown over recent years. Network pruning has been carefully studied in various applications to speed up the machine learning workflow, but mainstream pruning strategies often focus on specific connection significancy rather than the sample difficulty. U-Net++ as a well-versed and capable semantic segmentation deep convolutional neural network architecture, as well as its equivalents, are all facing the challenge of overconfidence, which will create barriers for a robust uncertainty-based pruning strategy to be designed. In the following study, we analyzed the efficiency of deep neural networks and semantic segmentation in satellite imagery analysis, and proposed a new tailored workf low of dynamic pruning for U-Net++ by combining the ideas of network calibration and uncertainty and defining the inference complexity of network input samples. We tested and illustrated the capability of this new workflow and delivered a successful comparative study on its effectiveness on the DeepGlobe satellite imagery road extraction dataset and how it can greatly reduce the computational cost with little performance drop.
更多查看译文
关键词
improved imagery throughput,uncertainty pruning,u-net
AI 理解论文
溯源树
样例
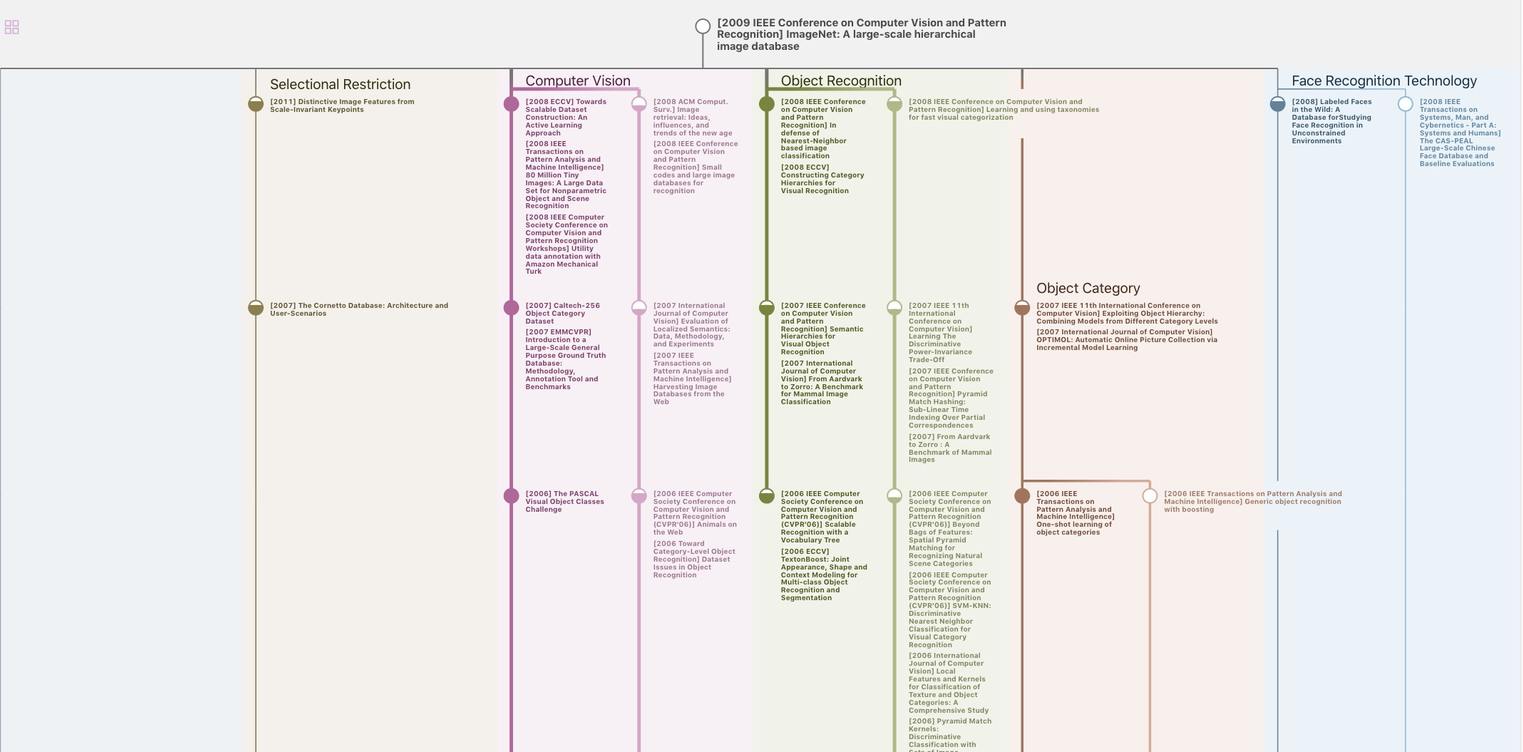
生成溯源树,研究论文发展脉络
Chat Paper
正在生成论文摘要