Neural network interpretation techniques for analysis of histological images of breast abnormalities
Гинекология(2023)
摘要
Background. Neural networks are actively used in digital pathology to analyze histological images and support medical decision-making. A common approach is to solve the classification problem, where only class labels are the only model responses. However, one should understand which areas of the image have the most significant impact on the model's response. Machine learning interpretation techniques help solve this problem. Aim. To study the consistency of different methods of neural network interpretation when classifying histological images of the breast and to obtain an expert assessment of the results of the evaluated methods. Materials and methods. We performed a preliminary analysis and pre-processing of the existing data set used to train pre-selected neural network models. The existing methods of visualizing the areas of attention of trained models on easy-to-understand data were applied, followed by verification of their correct use. The same neural network models were trained on histological data, and the selected interpretation methods were used to systematize histological images, followed by the evaluation of the results consistency and an expert assessment of the results. Results. In this paper, several methods of interpreting machine learning are studied using two different neural network architectures and a set of histological images of breast abnormalities. Results of ResNet18 and ViT-B-16 models training on a set of histological images on the test sample: accuracy metric 0.89 and 0.89, ROC_AUC metric 0.99 and 0.96, respectively. The results were also evaluated by an expert using the Label Studio tool. For each pair of images, the expert was asked to select the most appropriate answer ("Yes" or "No") to the question: "The highlighted areas generally correspond to the Malignant class." The "Yes" response rate for the ResNet_Malignant category was 0.56; for ViT_Malignant, it was 1.0. Conclusion. Interpretability experiments were conducted with two different architectures: the ResNet18 convolutional network and the ViT-B-16 attention-enhanced network. The results of the trained models were visualized using the GradCAM and Attention Rollout methods, respectively. First, experiments were conducted on a simple-to-interpret dataset to ensure they were used correctly. The methods are then applied to the set of histological images. In easy-to-understand images (cat images), the convolutional network is more consistent with human perception; on the contrary, in histological images of breast cancer, ViT-B-16 provided results much more similar to the expert's perception.
更多查看译文
关键词
digital pathology,interpretability,neural network
AI 理解论文
溯源树
样例
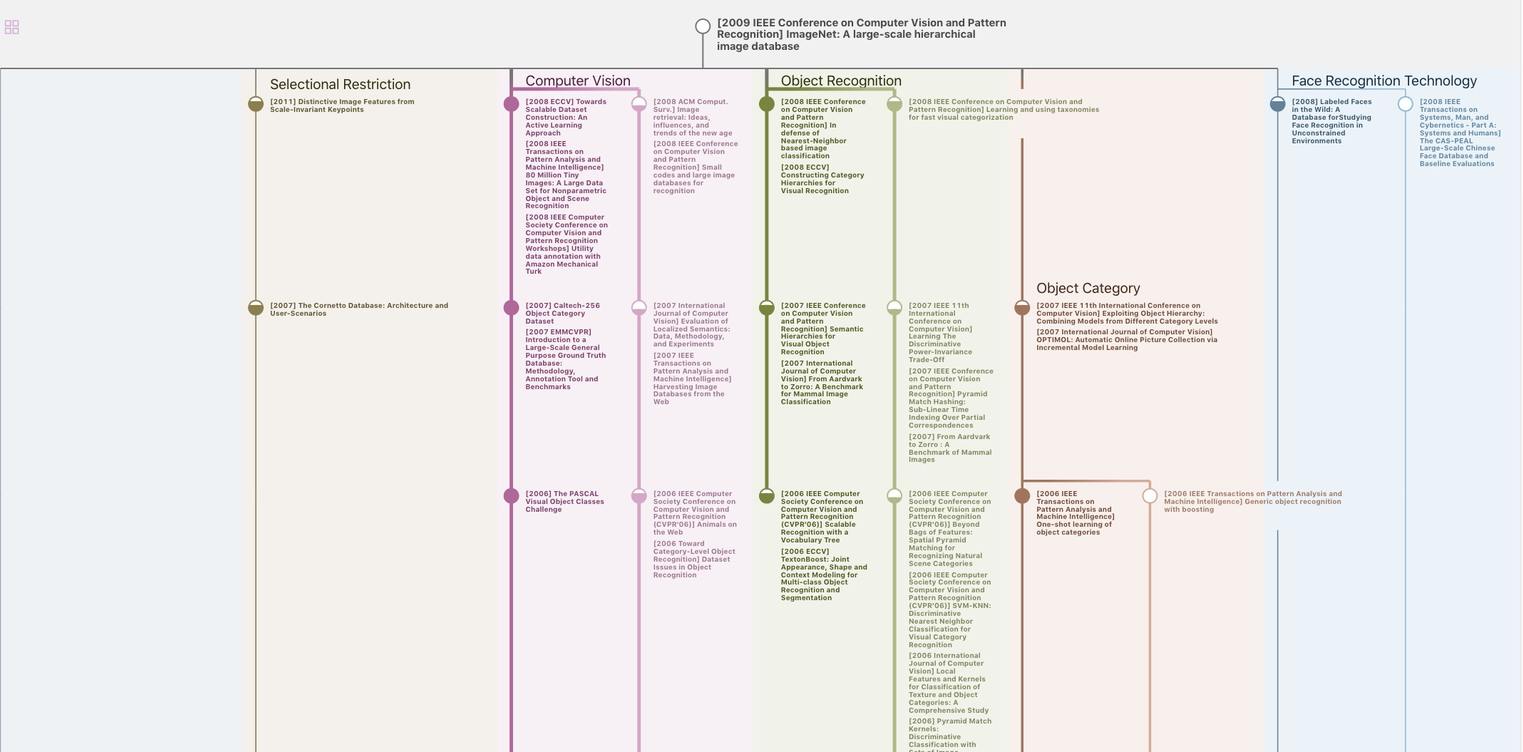
生成溯源树,研究论文发展脉络
Chat Paper
正在生成论文摘要