A novel strategy for designing the magic shotguns for distantly related target pairs.
Briefings in bioinformatics(2023)
摘要
Due to its promising capacity in improving drug efficacy, polypharmacology has emerged to be a new theme in the drug discovery of complex disease. In the process of novel multi-target drugs (MTDs) discovery, in silico strategies come to be quite essential for the advantage of high throughput and low cost. However, current researchers mostly aim at typical closely related target pairs. Because of the intricate pathogenesis networks of complex diseases, many distantly related targets are found to play crucial role in synergistic treatment. Therefore, an innovational method to develop drugs which could simultaneously target distantly related target pairs is of utmost importance. At the same time, reducing the false discovery rate in the design of MTDs remains to be the daunting technological difficulty. In this research, effective small molecule clustering in the positive dataset, together with a putative negative dataset generation strategy, was adopted in the process of model constructions. Through comprehensive assessment on 10 target pairs with hierarchical similarity-levels, the proposed strategy turned out to reduce the false discovery rate successfully. Constructed model types with much smaller numbers of inhibitor molecules gained considerable yields and showed better false-hit controllability than before. To further evaluate the generalization ability, an in-depth assessment of high-throughput virtual screening on ChEMBL database was conducted. As a result, this novel strategy could hierarchically improve the enrichment factors for each target pair (especially for those distantly related/unrelated target pairs), corresponding to target pair similarity-levels.
更多查看译文
关键词
dual inhibitor,enrichment factor,false discovery rate,polypharmacology,quantitative structure–activity relationship (QSAR),virtual screening
AI 理解论文
溯源树
样例
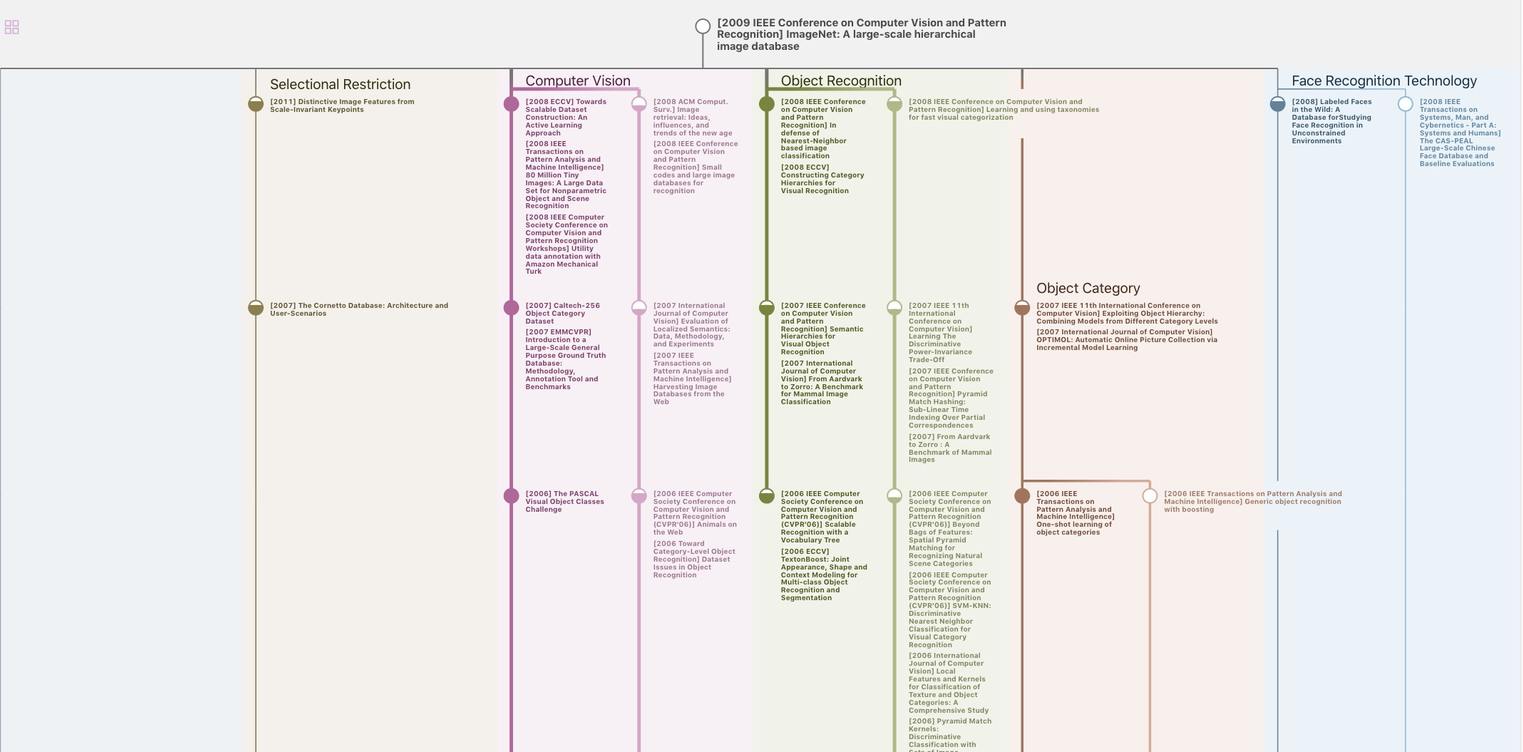
生成溯源树,研究论文发展脉络
Chat Paper
正在生成论文摘要