An Unsupervised Machine Learning Based Double Sweet Spots Classification and Evaluation Method for Tight Reservoirs
Journal of Energy Resources Technology(2023)
摘要
Abstract With the increasing exploration and development of tight sandstone gas reservoirs, it is of utmost importance to clarify the characteristics of “sweet spots” within tight gas reservoirs. Considering the complex lithology of tight gas reservoirs, fast phase transformations of sedimentary facies, and vital diagenetic transformation, there is a low success rate of reservoir prediction in the lateral direction, and heterogeneity evaluation is challenging. Establishing a convenient standard for reservoir interpretation in the early stages of development is complex, making designing hydraulic fracturing in the later phases a challenge. In this paper, we propose a detailed study of the engineering and geological double sweet spots (DSS) analysis system and the optimization of sweet spot parameters using the independent weight coefficient method. K-means++ algorithm and Gaussian mixture gradient algorithm unsupervised machine learning algorithms are used to determine the classification standard of general reservoirs and high-quality sweet spot reservoirs in the lower 1 layer of He-8 in the x block of the Sulige gas field. This application of the field example illustrates that the proposed double sweet spot classification and evaluation method can be applied to locate the reservoir's sweet spot accurately.
更多查看译文
关键词
classification,machine learning
AI 理解论文
溯源树
样例
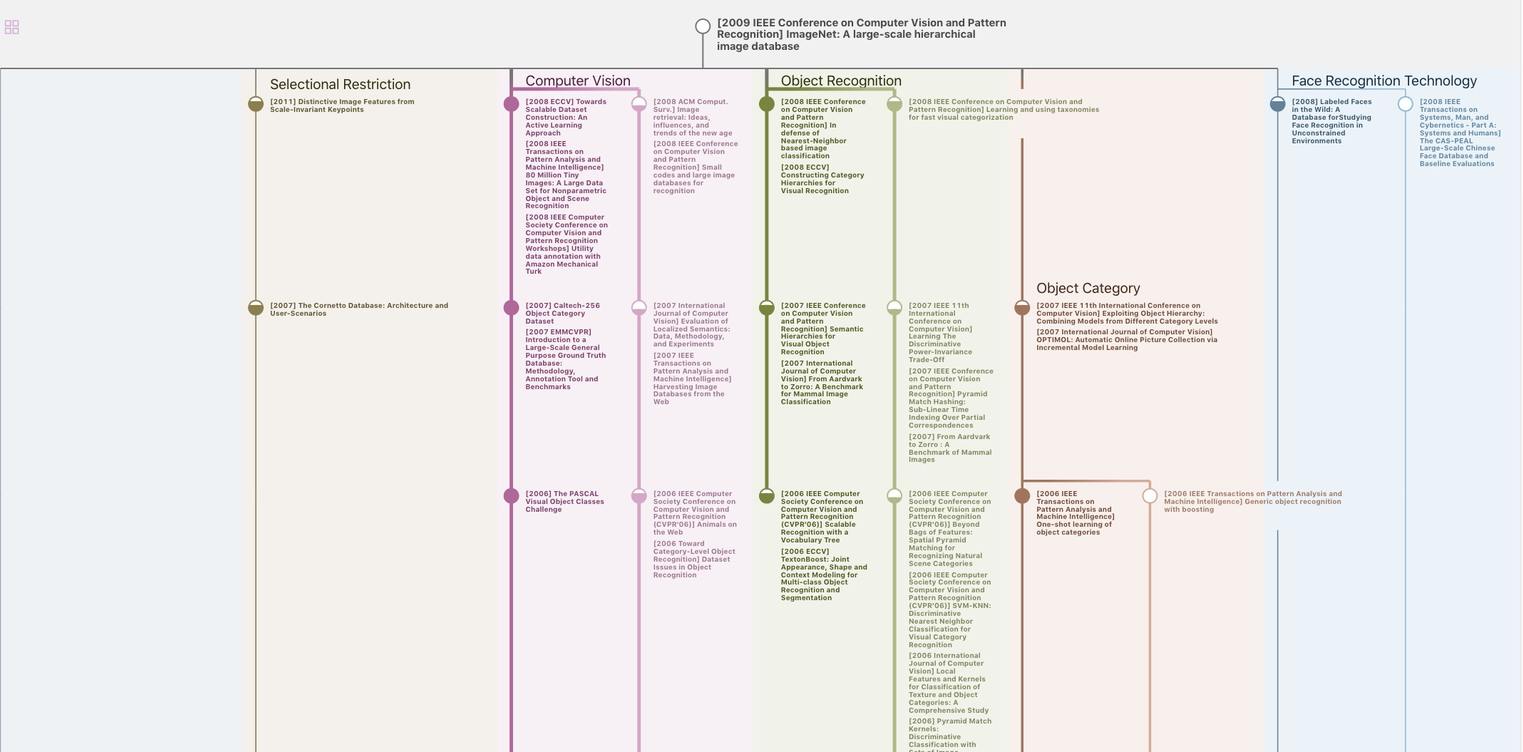
生成溯源树,研究论文发展脉络
Chat Paper
正在生成论文摘要