An investigation on worst‐case spectral reconstruction from RGB images via Radiance Mondrian World assumption
Color Research & Application(2023)
摘要
Spectral reconstruction (SR) algorithms recover hyperspectral measurements from RGB camera responses. Statistical models at different levels of complexity are used to solve the SR problem-from the simplest closed-form regression, to sparse coding, to the complex deep neural networks (DNN). Recently, these methods were benchmarked based on the mean performance of the models and on a fixed set of real-world scenes, suggesting that more complex (more non-linear) models generally deliver better SR. In this paper, we investigate the relative performances of these models in terms of a real-world worst-case imaging condition called the Radiance Mondrian World (RMW) assumption. Under the RMW, testing hyperspectral images are composed of randomly-arranged and overlapping rectangular patches, where each patch is filled with one random radiance spectrum uniformly sampled from the convex closure of all natural radiances (i.e., all spectra in the concerned hyperspectral image dataset). Surprisingly, we show that all compared algorithms-regardless of their model complexity-degrade to broadly the same level of performance on our RMW testing set. Further, by retraining all models with an RMW training set, we show that increasing model complexity also does not help learning better SR mappings from the RMW images. That is, using simple regression is as good as using a DNN. This similarity of performance is also shown to hold for images adhering to the conventional Mondrian World assumption (random reflectances lit by a single, per scene, randomly selected light source).
更多查看译文
关键词
color,hyperspectral imaging,inverse problem,multispectral imaging,spectra
AI 理解论文
溯源树
样例
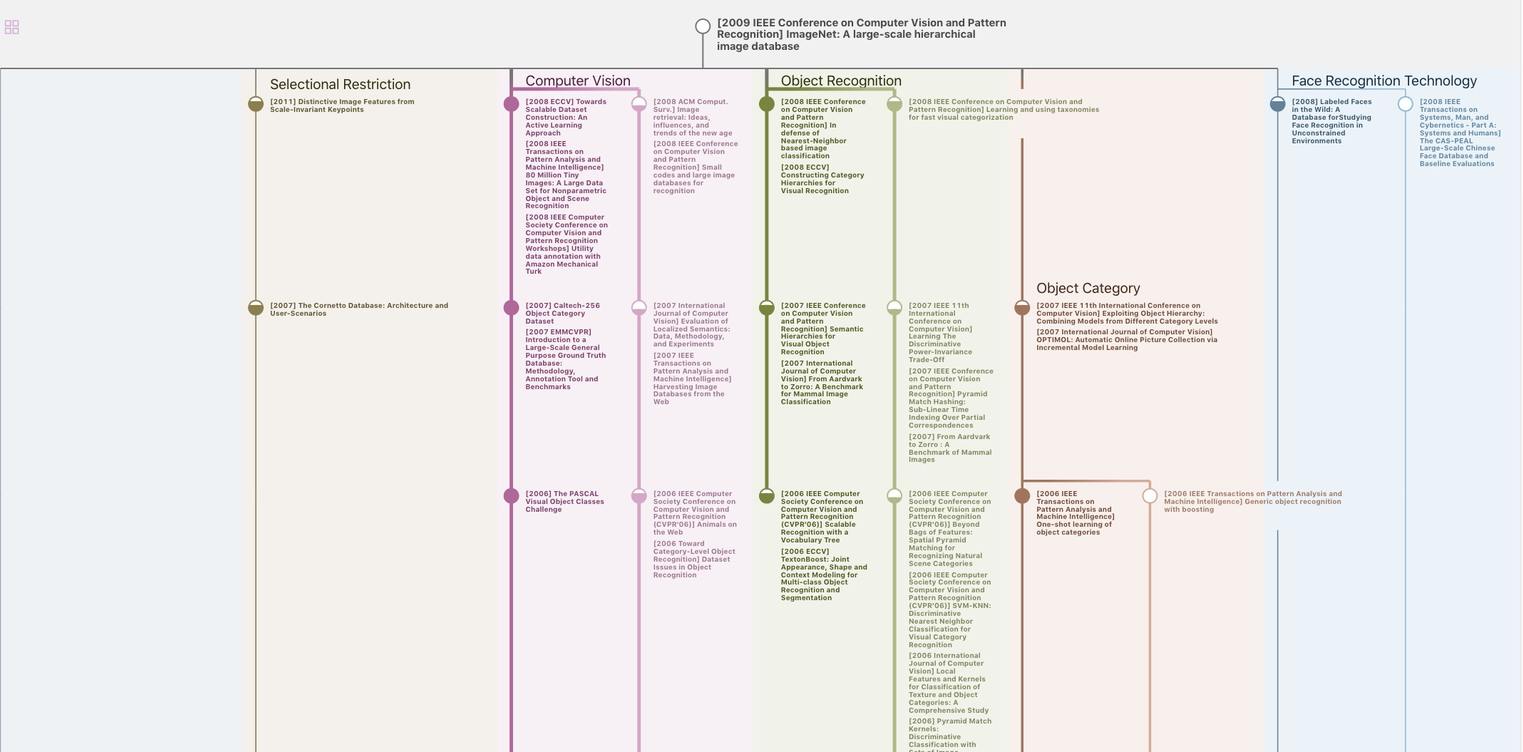
生成溯源树,研究论文发展脉络
Chat Paper
正在生成论文摘要