Off-Policy Evaluation with Out-of-Sample Guarantees
arxiv(2023)
摘要
We consider the problem of evaluating the performance of a decision policy using past observational data. The outcome of a policy is measured in terms of a loss (aka. disutility or negative reward) and the main problem is making valid inferences about its out-of-sample loss when the past data was observed under a different and possibly unknown policy. Using a sample-splitting method, we show that it is possible to draw such inferences with finite-sample coverage guarantees about the entire loss distribution, rather than just its mean. Importantly, the method takes into account model misspecifications of the past policy -- including unmeasured confounding. The evaluation method can be used to certify the performance of a policy using observational data under a specified range of credible model assumptions.
更多查看译文
关键词
evaluation,off-policy,out-of-sample
AI 理解论文
溯源树
样例
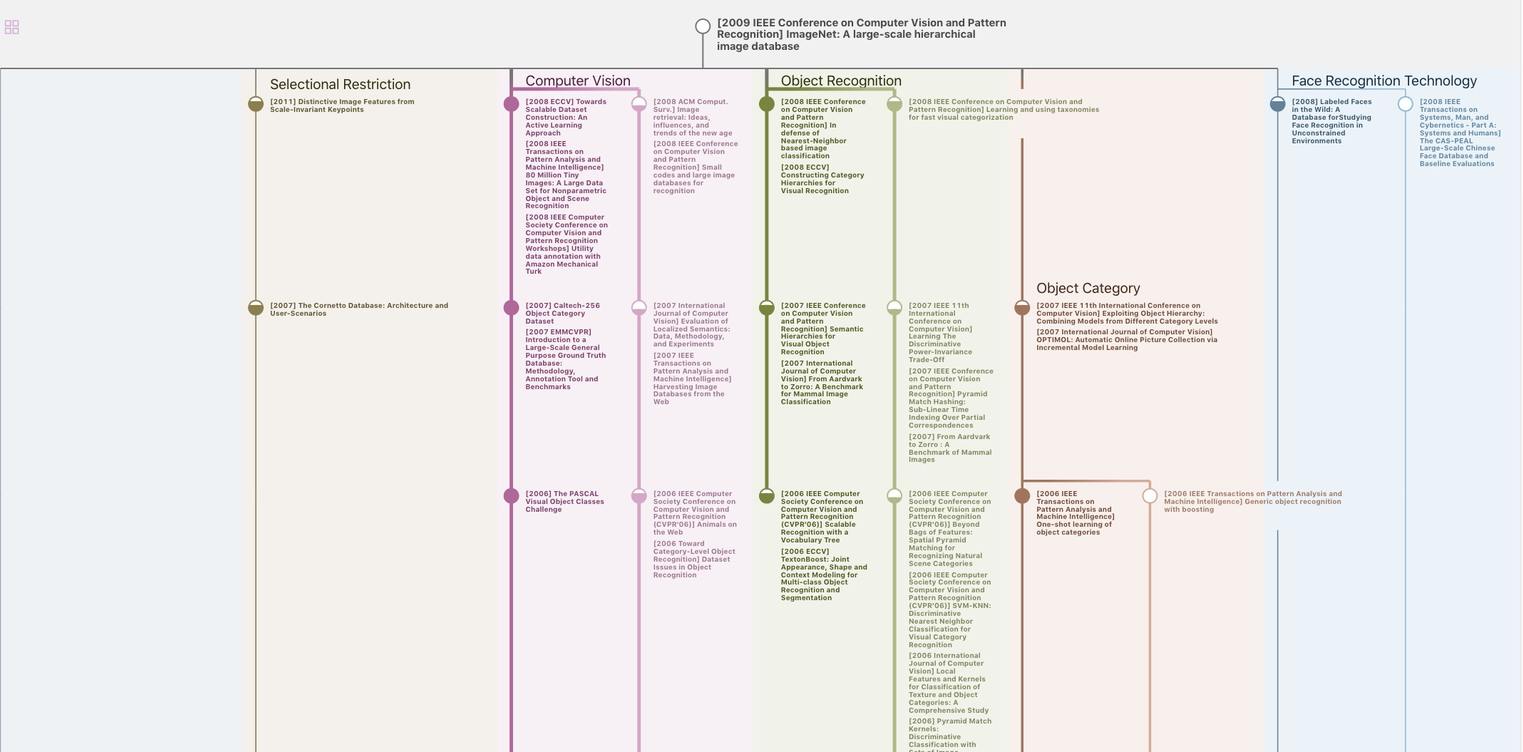
生成溯源树,研究论文发展脉络
Chat Paper
正在生成论文摘要