An LMI Framework for Contraction-based Nonlinear Control Design by Derivatives of Gaussian Process Regression
arxiv(2023)
摘要
Contraction theory formulates the analysis of nonlinear systems in terms of Jacobian matrices. Although this provides the potential to develop a linear matrix inequality (LMI) framework for nonlinear control design, conditions are imposed not on controllers but on their partial derivatives, which makes control design challenging. In this paper, we illustrate this so-called integrability problem can be solved by a non-standard use of Gaussian process regression (GPR) for parameterizing controllers and then establish an LMI framework of contraction-based control design for nonlinear discrete-time systems, as an easy-to-implement tool. Later on, we consider the case where the drift vector fields are unknown and employ GPR for functional fitting as its standard use. GPR describes learning errors in terms of probability, and thus we further discuss how to incorporate stochastic learning errors into the proposed LMI framework.
更多查看译文
关键词
nonlinear control design,lmi framework,contraction-based
AI 理解论文
溯源树
样例
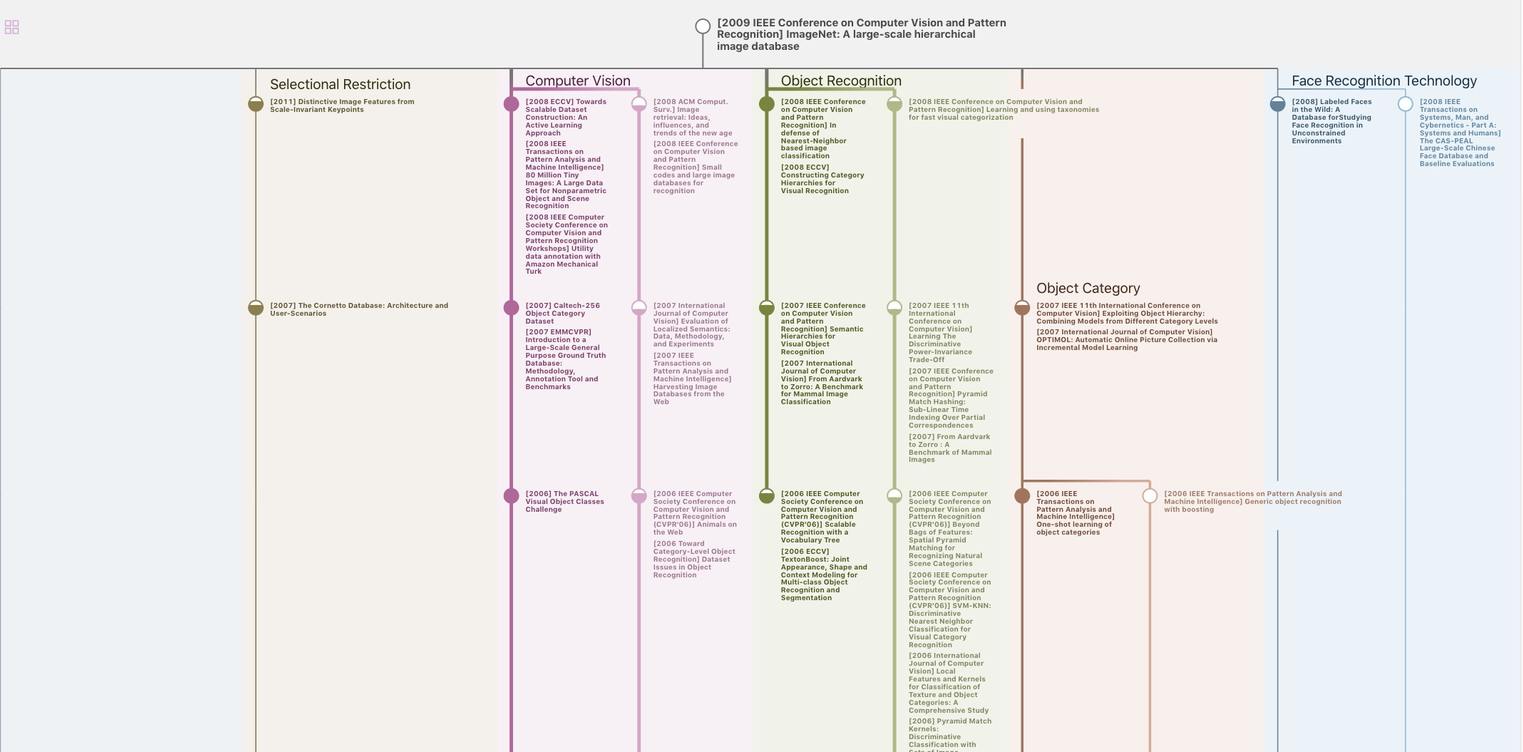
生成溯源树,研究论文发展脉络
Chat Paper
正在生成论文摘要