DIST: spatial transcriptomics enhancement using deep learning.
Briefings in bioinformatics(2023)
摘要
Spatially resolved transcriptomics technologies enable comprehensive measurement of gene expression patterns in the context of intact tissues. However, existing technologies suffer from either low resolution or shallow sequencing depth. Here, we present DIST, a deep learning-based method that imputes the gene expression profiles on unmeasured locations and enhances the gene expression for both original measured spots and imputed spots by self-supervised learning and transfer learning. We evaluate the performance of DIST for imputation, clustering, differential expression analysis and functional enrichment analysis. The results show that DIST can impute the gene expression accurately, enhance the gene expression for low-quality data, help detect more biological meaningful differentially expressed genes and pathways, therefore allow for deeper insights into the biological processes.
更多查看译文
关键词
denoising,imputation,self-supervised learning,spatial transcriptomics,transfer learning
AI 理解论文
溯源树
样例
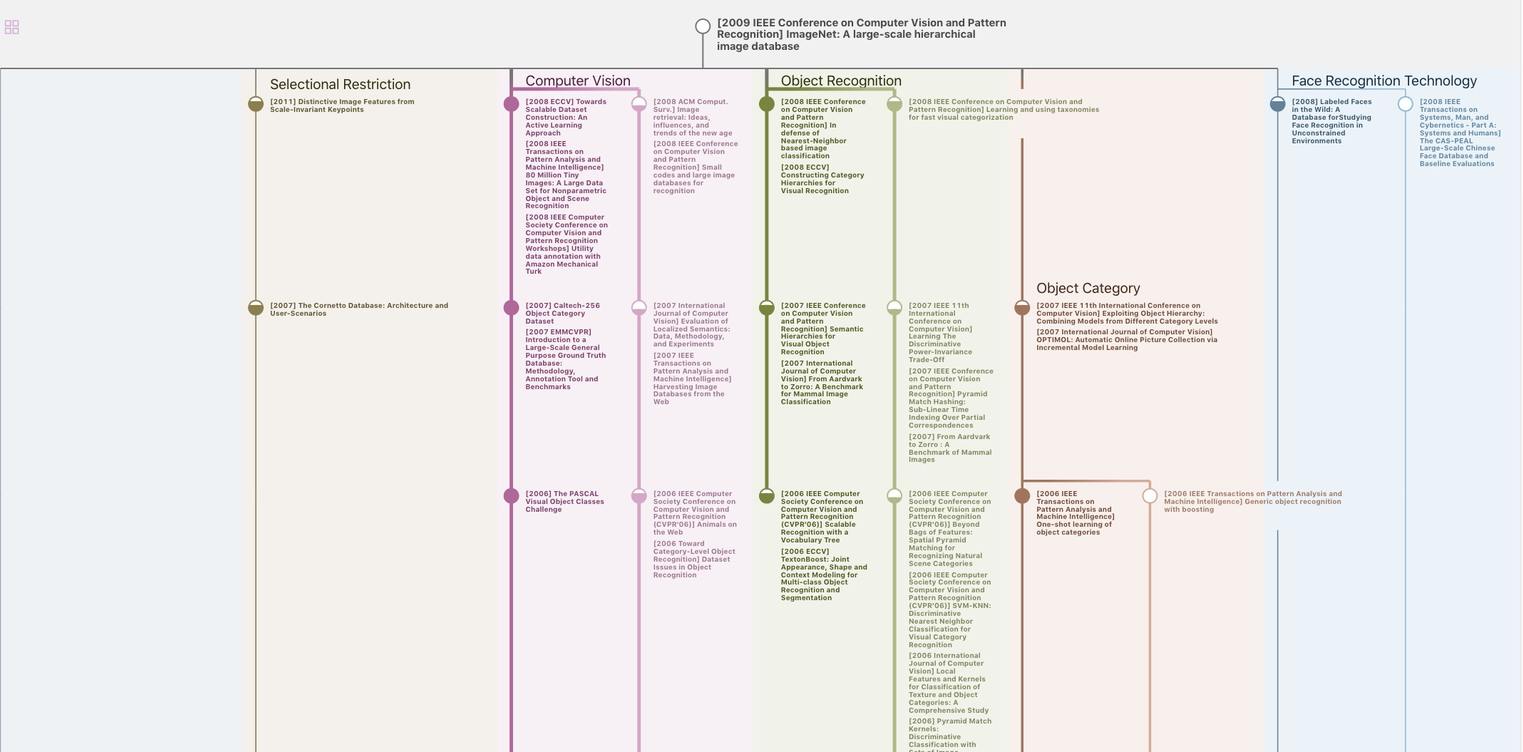
生成溯源树,研究论文发展脉络
Chat Paper
正在生成论文摘要