A further study in the prediction of viscosity for Iranian crude oil reservoirs by utilizing a robust radial basis function (RBF) neural network model
Neural Computing and Applications(2023)
摘要
In this study, a robust radial basis function neural network (RBF-NN) is developed for predicting Iranian crude oil viscosity in an extensive and precise way. Experimental data incorporate the PVT data of 720 samples gathered from Iranian central, southern, and offshore oil fields. The proposed RBF-NN model uses temperature, pressure, and parameters obtained by PVT analyses including oil and gas specific gravity, and solution gas–oil ratio as independent variables. The evaluation process was employed in three different regions; above, at, and below the bubble point pressure ( P b ). The proposed RBF-NN model outputs were evaluated statistically using experimental data, and the results were compared side by side with the results of the previous studies including a multilayer perceptron neural network (MLP-NN) and the five well-established semiempirical equations. Sensitivity analyses were performed using the numeric sensitivity analyses (NSA) method and the results showed the highest and lowest impacts on the predicted crude oil viscosity between input parameters related to oil and gas specific gravity with 42 and 0%, respectively. The results show the proposed RBF-NN model with the average absolute relative deviation (AARD%) of 1.69, 4.56, and 2.04% for above, at, and below the bubble point pressure ( P b ) regions, respectively, is the most precise and consistent method for predicting crude oil viscosity compared with those published in the literature.
更多查看译文
关键词
Crude oil viscosity,Artificial neural network,Empirical equations,Radial basis function
AI 理解论文
溯源树
样例
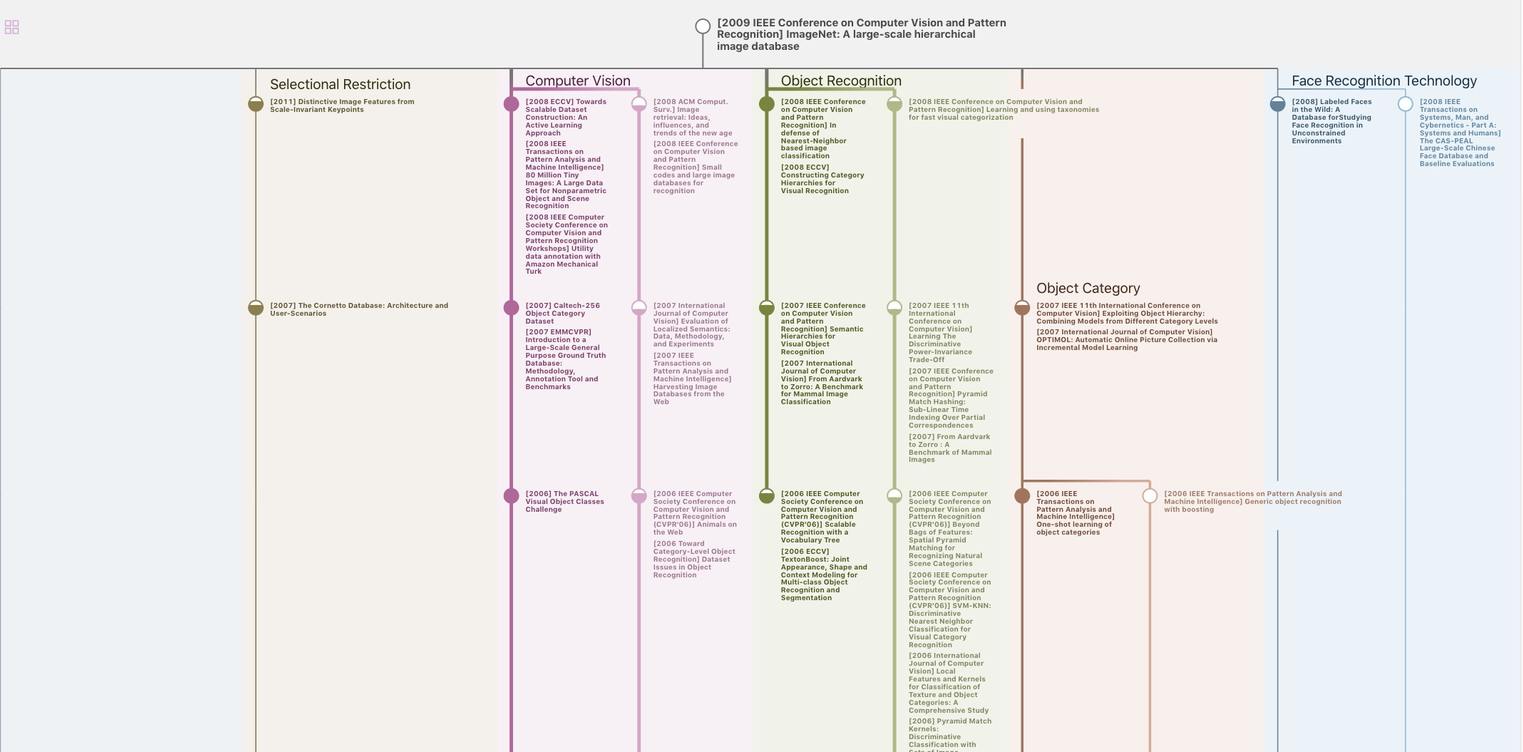
生成溯源树,研究论文发展脉络
Chat Paper
正在生成论文摘要