Improved U-Net based on cross-layer connection for pituitary adenoma MRI image segmentation.
Mathematical biosciences and engineering : MBE(2023)
摘要
Pituitary adenoma is a common neuroendocrine neoplasm, and most of its MR images are characterized by blurred edges, high noise and similar to surrounding normal tissues. Therefore, it is extremely difficult to accurately locate and outline the lesion of pituitary adenoma. To sovle these limitations, we design a novel deep learning framework for pituitary adenoma MRI image segmentation. Under the framework of U-Net, a newly cross-layer connection is introduced to capture richer multi-scale features and contextual information. At the same time, full-scale skip structure can reasonably utilize the above information obtained by different layers. In addition, an improved inception-dense block is designed to replace the classical convolution layer, which can enlarge the effectiveness of the receiving field and increase the depth of our network. Finally, a novel loss function based on binary cross-entropy and Jaccard losses is utilized to eliminate the problem of small samples and unbalanced data. The sample data were collected from 30 patients in Quzhou People's Hospital, with a total of 500 lesion images. Experimental results show that although the amount of patient sample is small, the proposed method has better performance in pituitary adenoma image compared with existing algorithms, and its Dice, Intersection over Union (IoU), Matthews correlation coefficient (Mcc) and precision reach 88.87, 80.67, 88.91 and 97.63%, respectively.
更多查看译文
关键词
U-Net , cross-layer connection , image segmentation , inception-dense , pituitary adenoma
AI 理解论文
溯源树
样例
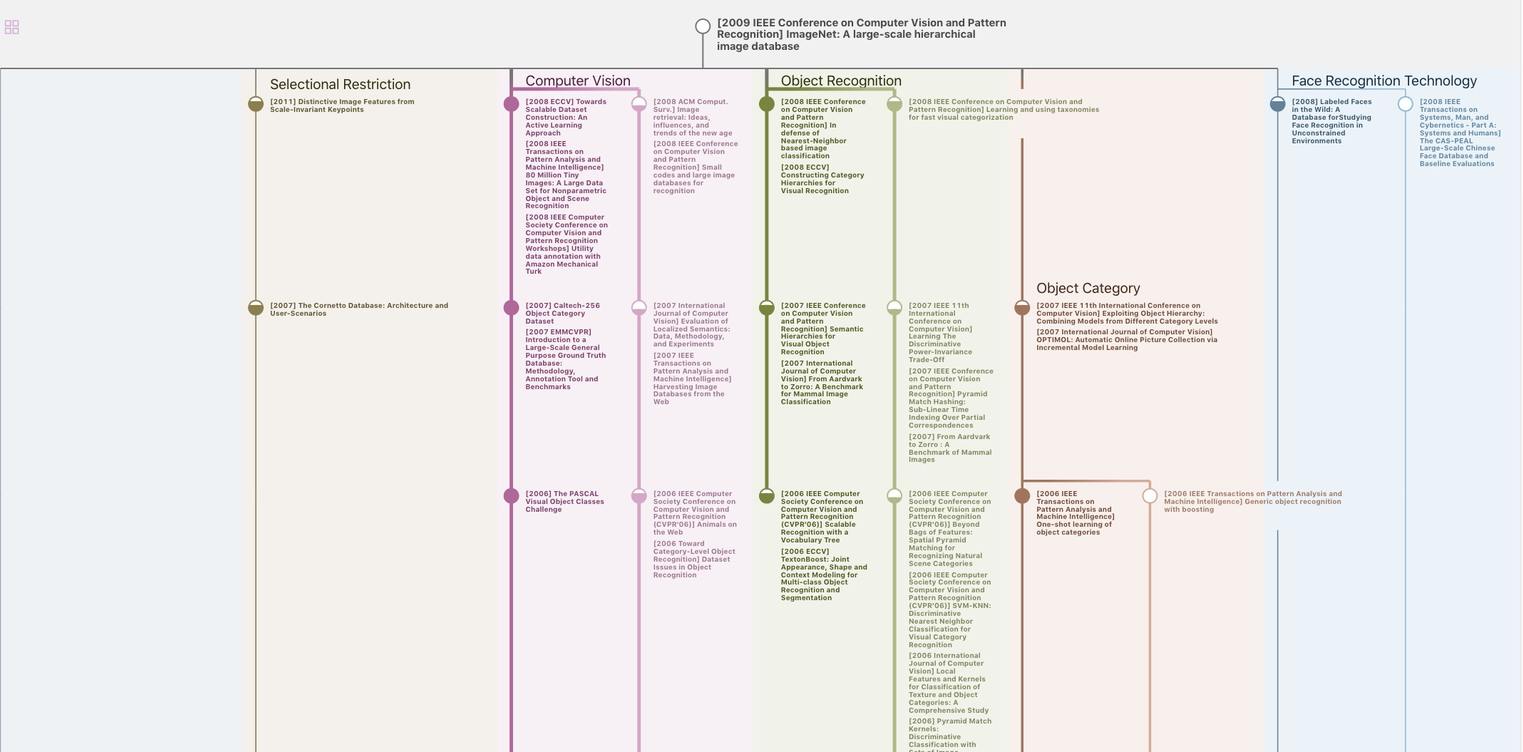
生成溯源树,研究论文发展脉络
Chat Paper
正在生成论文摘要