Deep Hashing via Dynamic Similarity Learning for Image Retrieval
2022 IEEE 8th International Conference on Cloud Computing and Intelligent Systems (CCIS)(2022)
摘要
Hashing has been commonly used in large-scale image retrieval. Due to the explosive expansion of data, traditional deep hashing methods are not able to extract features explicitly, which leads to inefficient learning of hash codes. Accordingly, in the proposed method, we use the latest backbone network called ConvNeXt for feature extraction, which not only has superior performance for feature extraction from larger scales datasets, but also has fewer parameters with higher training efficiency. Consequently, to capture the true similarity among images, different from existing methods that pre-define a similarity matrix, we learn the similarity matrix during training. We perform comprehensive experiments on three widely-studied datasets: CIFAR-10, NUSWIDE, and ImageNet. The proposed method shows superior performance compared with several state-of-the-art techniques.
更多查看译文
关键词
Hashing,Features Extraction,ConvNeXt,Similarity Matrix
AI 理解论文
溯源树
样例
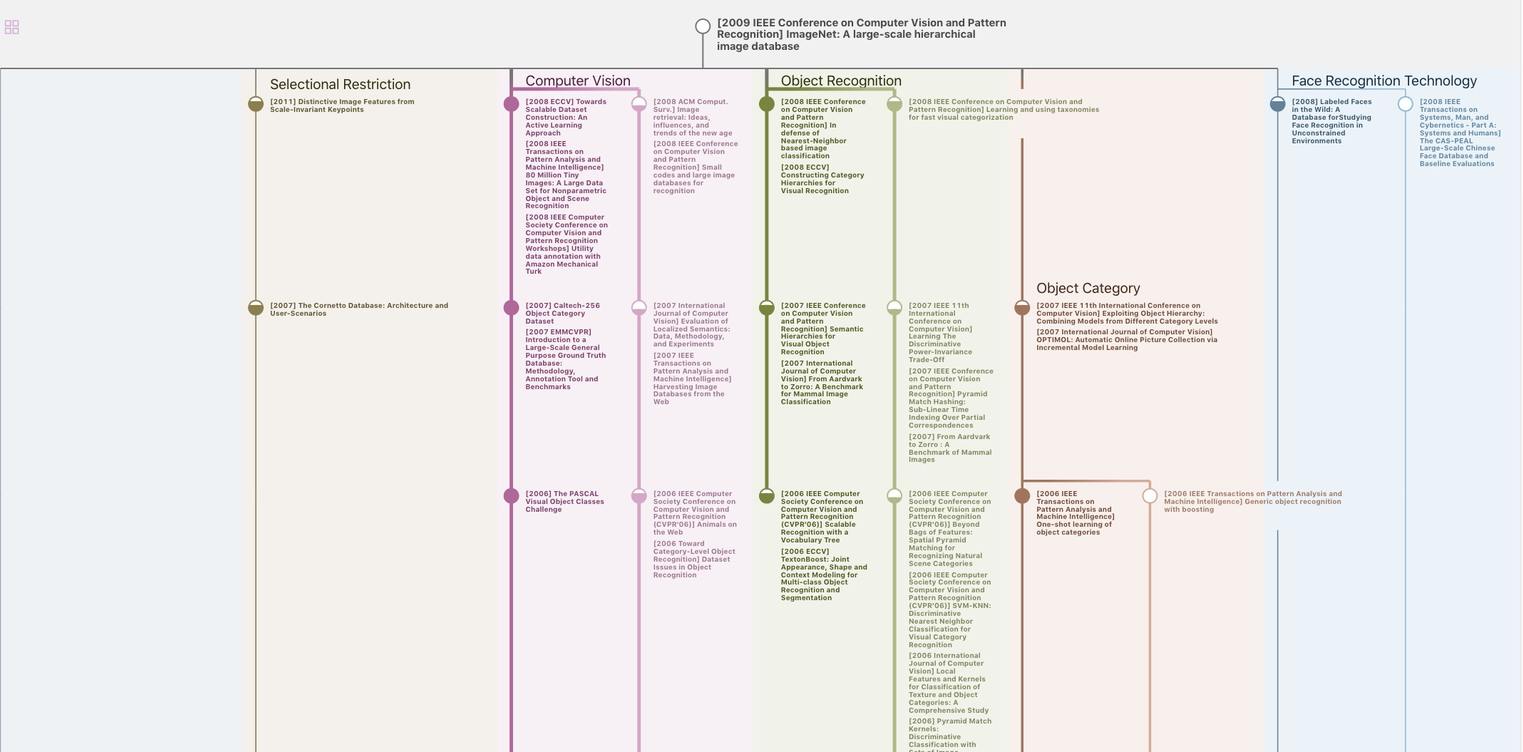
生成溯源树,研究论文发展脉络
Chat Paper
正在生成论文摘要