Citywide Traffic Volume Inference using Traffic Sensing Data with Missing Values
2022 IEEE 8th International Conference on Cloud Computing and Intelligent Systems (CCIS)(2022)
摘要
Sensing the traffic volume in the whole city is a crucial task in smart transportation systems. However, because of the high cost of installation and maintenance of traffic sensors, the coverage of traffic sensors is very low. Due to the influence of various factors such as device failure, network transmission, and bad weather, the traffic state of some road segments with sensors is not observed. In this work, we present a new framework for citywide traffic Volume Inference using traffic sensing data with Missing values (VIM). In VIM, we construct an affinity graph to model feature and spatial similarity of road segments based on spatial and feature information for each time interval, and then use a graph convolution network to learn the embeddings of road segments in each time interval. To capture the strong temporal dependencies, we propose to use a temporal attention network to update the embeddings of road segments. Specifically, we design a data imputation module, which utilizes the periodic data to fill in the missing values on each segment. Furthermore, we also propose a semi-supervised traffic volume objective function to guide the learning of GCN and temporal attention network. Extensive experiments on two real-world datasets in two cities show the effectiveness of the suggested framework in comparison to state-of-the-art baseline approach.
更多查看译文
关键词
Traffic Volume Inference,Traffic Sensing Data,Graph Convolution Network,Missing values
AI 理解论文
溯源树
样例
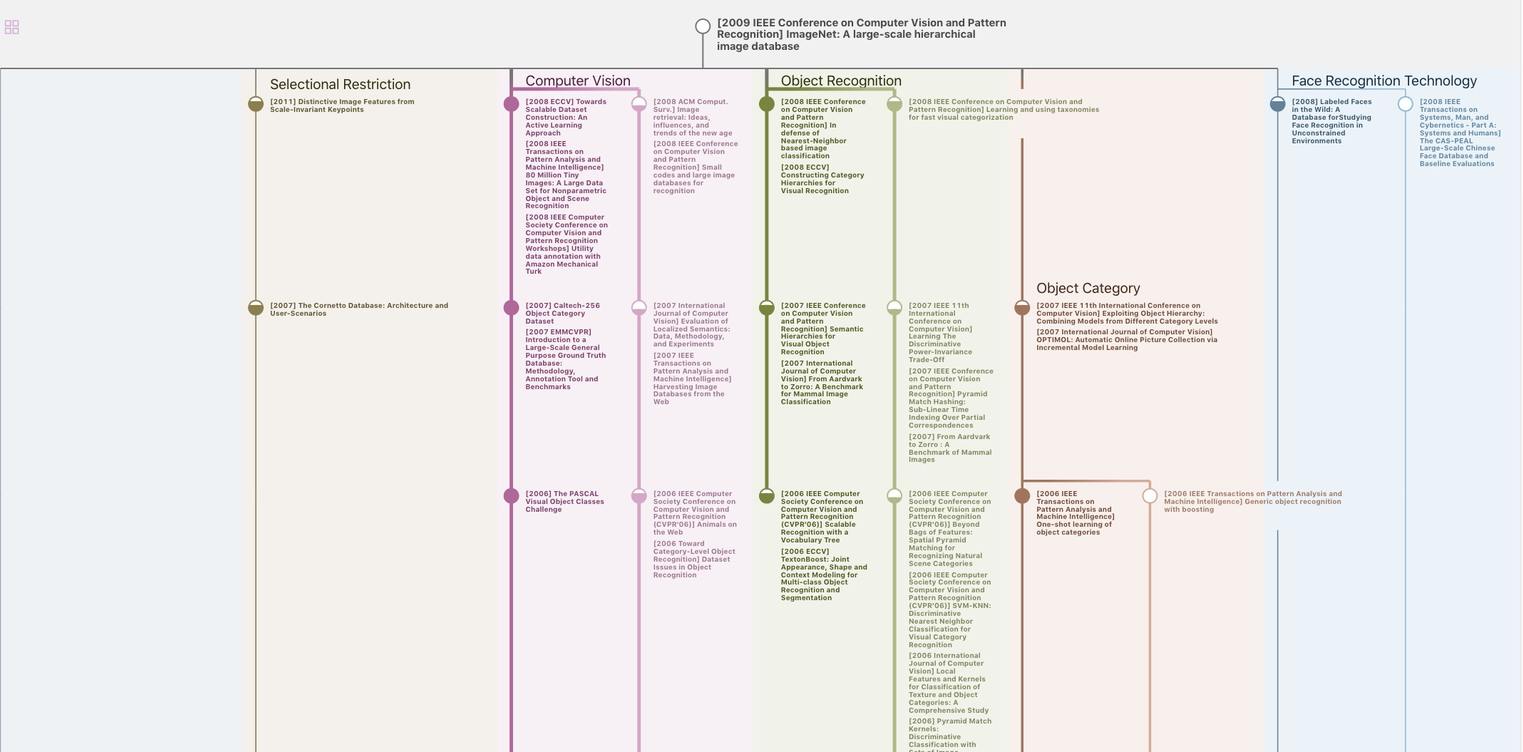
生成溯源树,研究论文发展脉络
Chat Paper
正在生成论文摘要