New Metrics to Encourage Innovation and Diversity in Information Retrieval Approaches
arxiv(2023)
摘要
In evaluation campaigns, participants often explore variations of popular, state-of-the-art baselines as a low-risk strategy to achieve competitive results. While effective, this can lead to local "hill climbing" rather than more radical and innovative departure from standard methods. Moreover, if many participants build on similar baselines, the overall diversity of approaches considered may be limited. In this work, we propose a new class of IR evaluation metrics intended to promote greater diversity of approaches in evaluation campaigns. Whereas traditional IR metrics focus on user experience, our two "innovation" metrics instead reward exploration of more divergent, higher-risk strategies finding relevant documents missed by other systems. Experiments on four TREC collections show that our metrics do change system rankings by rewarding systems that find such rare, relevant documents. This result is further supported by a controlled, synthetic data experiment, and a qualitative analysis. In addition, we show that our metrics achieve higher evaluation stability and discriminative power than the standard metrics we modify. To support reproducibility, we share our source code.
更多查看译文
关键词
Evaluation, Metrics, Information retrieval
AI 理解论文
溯源树
样例
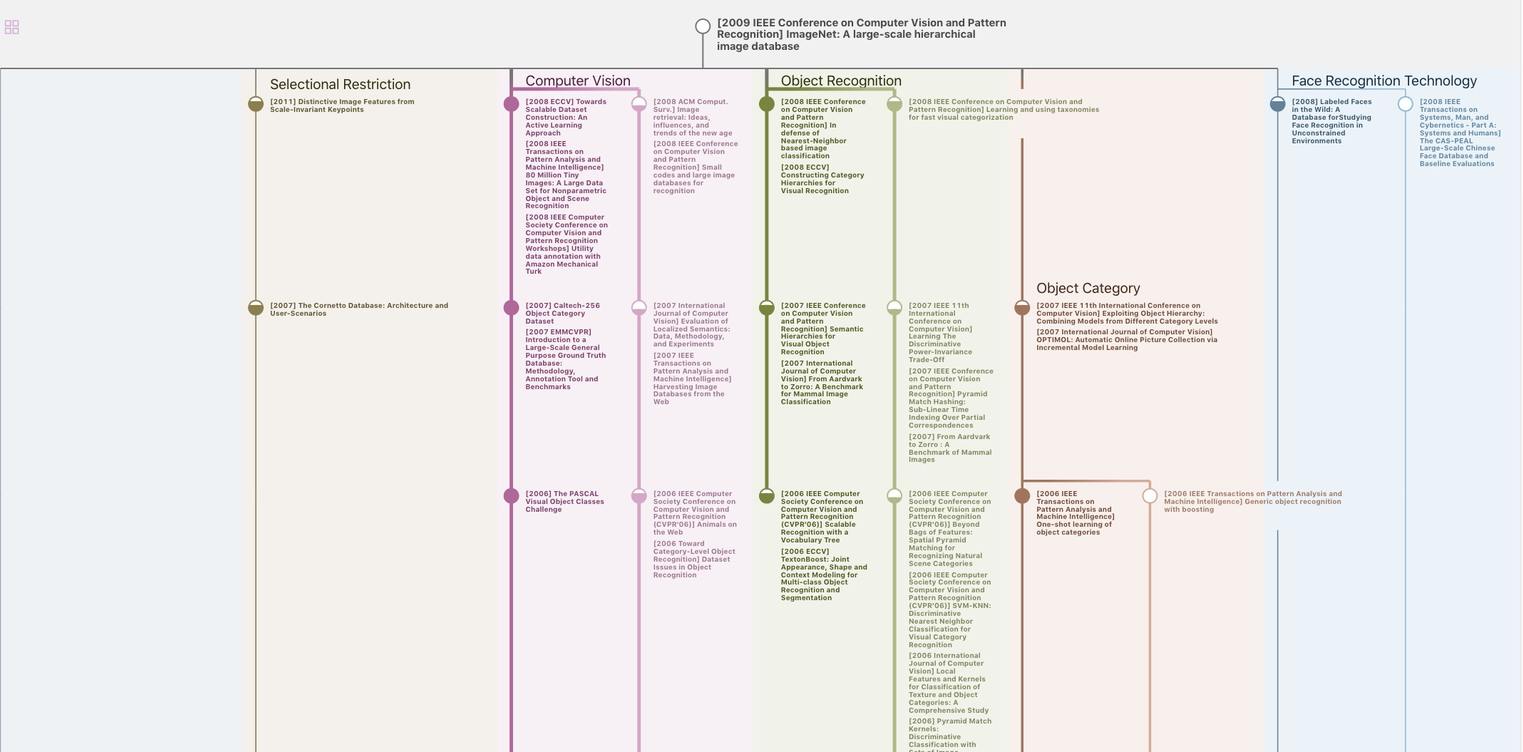
生成溯源树,研究论文发展脉络
Chat Paper
正在生成论文摘要