A Survey of Meta-Reinforcement Learning
arxiv(2023)
摘要
While deep reinforcement learning (RL) has fueled multiple high-profile successes in machine learning, it is held back from more widespread adoption by its often poor data efficiency and the limited generality of the policies it produces. A promising approach for alleviating these limitations is to cast the development of better RL algorithms as a machine learning problem itself in a process called meta-RL. Meta-RL is most commonly studied in a problem setting where, given a distribution of tasks, the goal is to learn a policy that is capable of adapting to any new task from the task distribution with as little data as possible. In this survey, we describe the meta-RL problem setting in detail as well as its major variations. We discuss how, at a high level, meta-RL research can be clustered based on the presence of a task distribution and the learning budget available for each individual task. Using these clusters, we then survey meta-RL algorithms and applications. We conclude by presenting the open problems on the path to making meta-RL part of the standard toolbox for a deep RL practitioner.
更多查看译文
关键词
learning,survey,meta-reinforcement
AI 理解论文
溯源树
样例
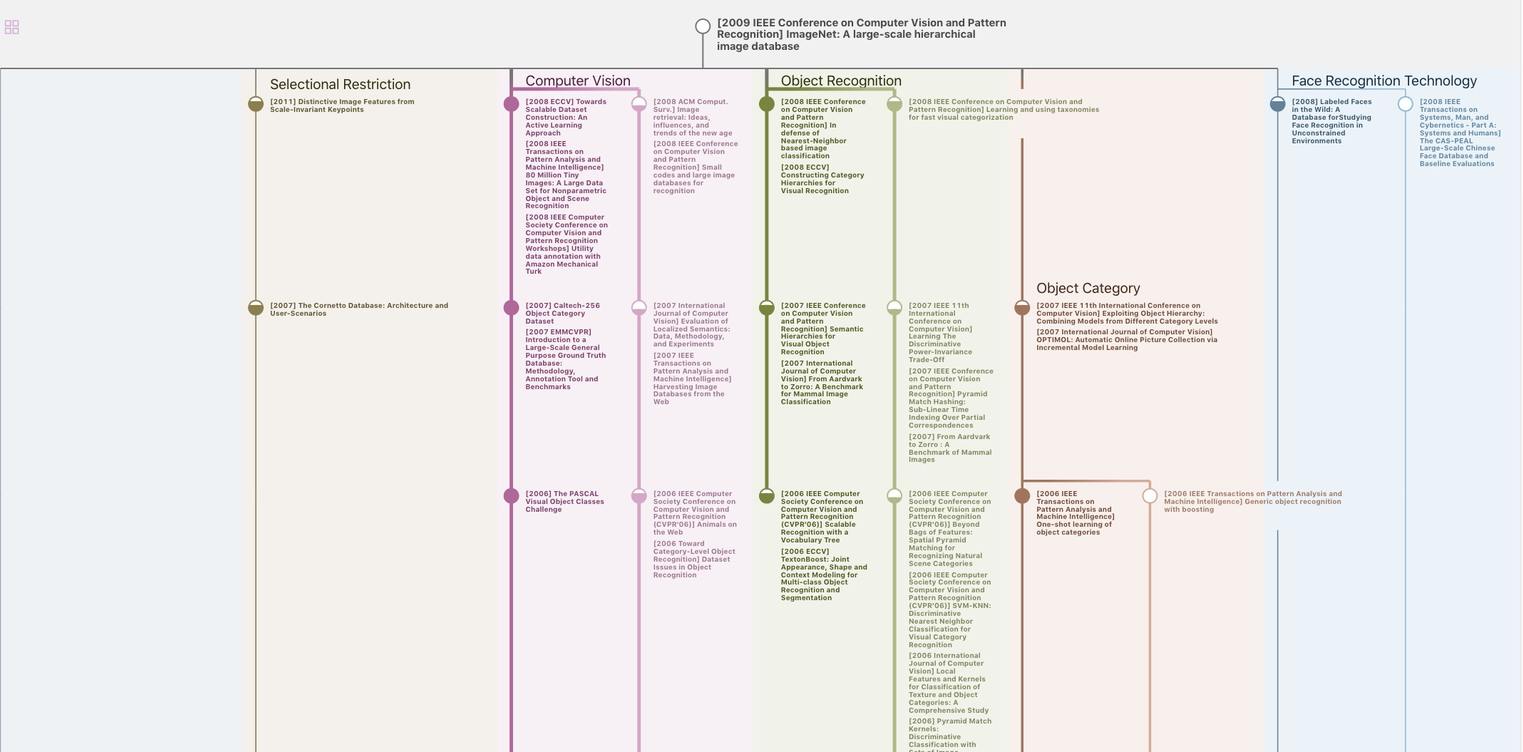
生成溯源树,研究论文发展脉络
Chat Paper
正在生成论文摘要