A novel dataset and a two-stage mitosis nuclei detection method based on hybrid anchor branch
BIOMEDICAL SIGNAL PROCESSING AND CONTROL(2024)
摘要
Mitosis detection is one of the important problems in computational pathology, and mitotic count is an important index of breast cancer grading for pathologists. However, the current count of mitotic nuclei relies on pathologists observing the number of mitotic nuclei in hotspots under a microscope, which is subjective and time-consuming. In this paper, we propose a two-stage cascaded network, named FoCasNet, for mitosis detection. In the first stage, a detection network is proposed to screen as many candidates as possible. In the second stage, a classification network is proposed to refine the results of the first stage. In addition, the attention mechanism, normalization method, and hybrid anchor branch classification subnet are introduced to improve the overall detection performance. Our method achieves the current highest F1-score of 0.888 on the public dataset ICPR 2012. We also evaluated our method on the GZMH dataset released by our research group for the first time and reached the highest F1-score of 0.563, which is also better than the state-of-the-art detection networks. It confirmed the effectiveness and generalization of our method. The code will be available at: https://github.com/antifen/mitosis-nuclei-detection.
更多查看译文
关键词
Pathological image,Mitosis nuclei detection,Cascade network,Hybrid anchor branch,Group normalization
AI 理解论文
溯源树
样例
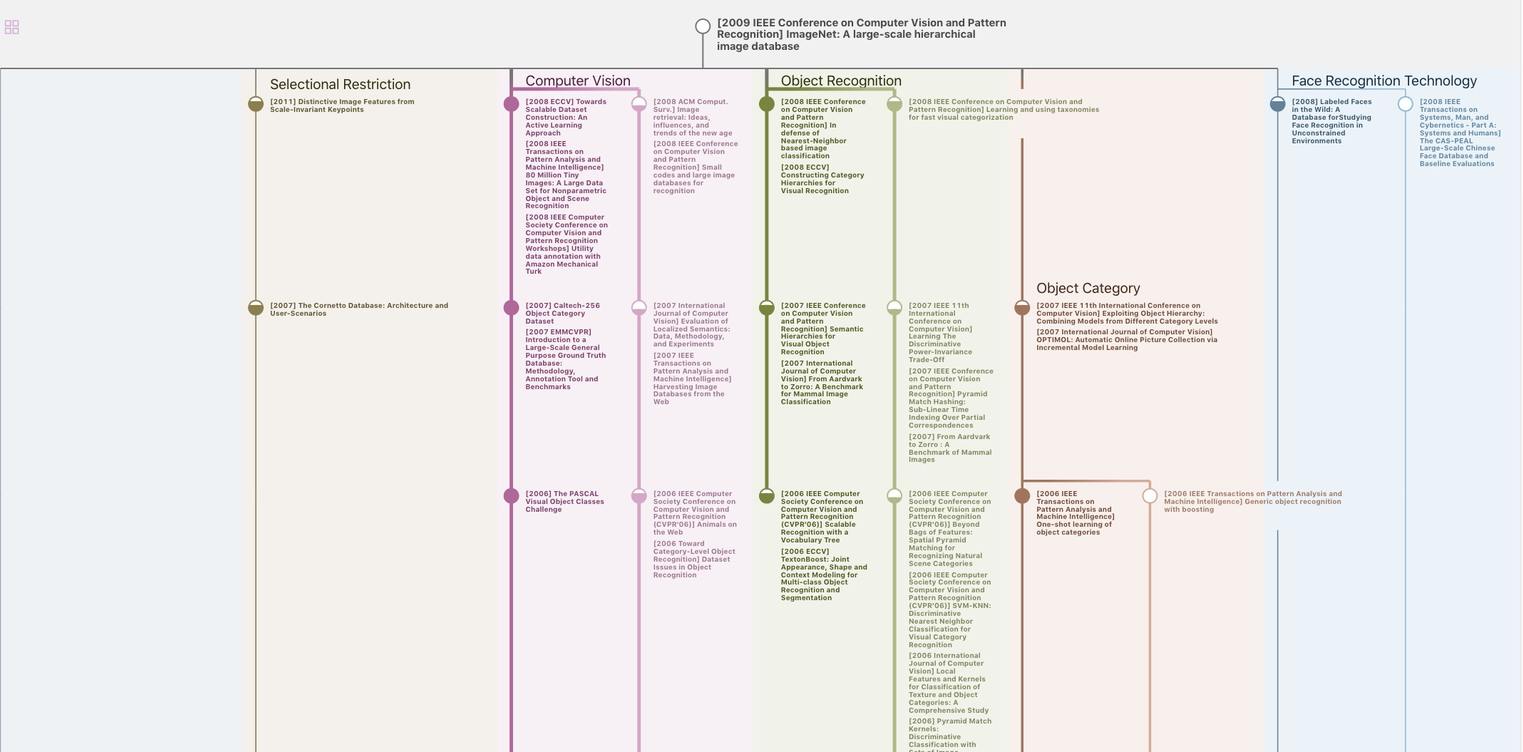
生成溯源树,研究论文发展脉络
Chat Paper
正在生成论文摘要