Failure Tolerant Training With Persistent Memory Disaggregation Over CXL
IEEE Micro(2023)
摘要
This article proposes TrainingCXL that can efficiently process large-scale recommendation datasets in the pool of disaggregated memory while making training fault tolerant with low overhead. To this end, we integrate persistent memory (PMEM) and graphics processing unit (GPU) into a cache-coherent domain as type 2. Enabling Compute Express Link (CXL) allows PMEM to be directly placed in GPU's memory hierarchy, such that GPU can access PMEM without software intervention. TrainingCXL introduces computing and checkpointing logic near the CXL controller, thereby training data and managing persistency in an active manner. Considering PMEM's vulnerability, we utilize the unique characteristics of recommendation models and take the checkpointing overhead off the critical path of their training. Finally, TrainingCXL employs an advanced checkpointing technique that relaxes the updating sequence of model parameters and embeddings across training batches. The evaluation shows that TrainingCXL achieves 5.2x training performance improvement and 76% energy savings, compared to the modern PMEM-based recommendation systems.
更多查看译文
关键词
Training,Checkpointing,Software,Graphics processing units,Computational modeling,Hardware,Task analysis
AI 理解论文
溯源树
样例
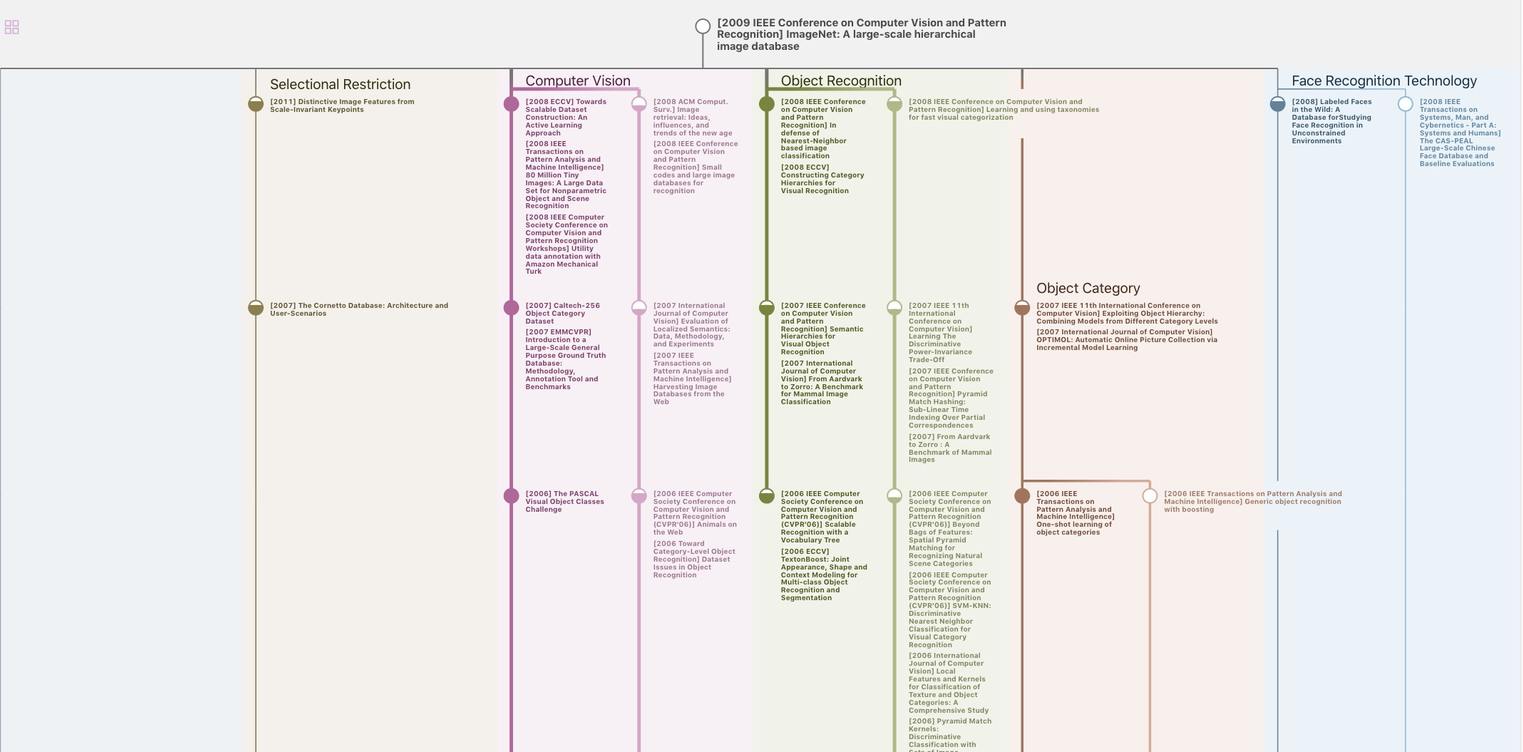
生成溯源树,研究论文发展脉络
Chat Paper
正在生成论文摘要