A variational autoencoder-based nonnegative matrix factorisation model for deep dictionary learning
arxiv(2023)
摘要
Construction of dictionaries using nonnegative matrix factorisation (NMF) has extensive applications in signal processing and machine learning. With the advances in deep learning, training compact and robust dictionaries using deep neural networks, i.e., dictionaries of deep features, has been proposed. In this study, we propose a probabilistic generative model which employs a variational autoencoder (VAE) to perform nonnegative dictionary learning. In contrast to the existing VAE models, we cast the model under a statistical framework with latent variables obeying a Gamma distribution and design a new loss function to guarantee the nonnegative dictionaries. We adopt an acceptance-rejection sampling reparameterization trick to update the latent variables iteratively. We apply the dictionaries learned from VAE-NMF to two signal processing tasks, i.e., enhancement of speech and extraction of muscle synergies. Experimental results demonstrate that VAE-NMF performs better in learning the latent nonnegative dictionaries in comparison with state-of-the-art methods.
更多查看译文
关键词
nonnegative matrix factorisation model
AI 理解论文
溯源树
样例
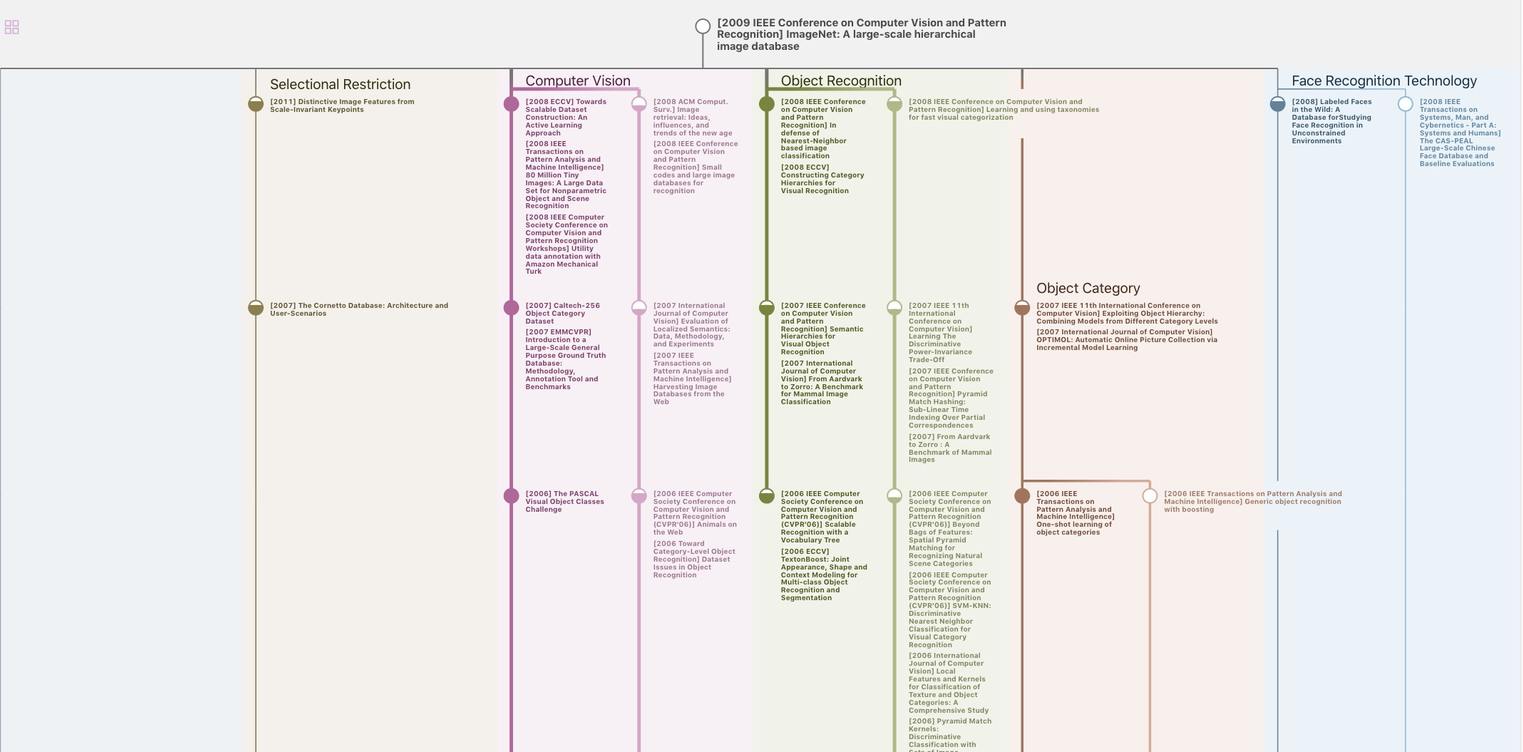
生成溯源树,研究论文发展脉络
Chat Paper
正在生成论文摘要