SCARP: 3D Shape Completion in ARbitrary Poses for Improved Grasping
2023 IEEE INTERNATIONAL CONFERENCE ON ROBOTICS AND AUTOMATION, ICRA(2023)
摘要
Recovering full 3D shapes from partial observations is a challenging task that has been extensively addressed in the computer vision community. Many deep learning methods tackle this problem by training 3D shape generation networks to learn a prior over the full 3D shapes. In this training regime, the methods expect the inputs to be in a fixed canonical form, without which they fail to learn a valid prior over the 3D shapes. We propose SCARP, a model that performs Shape Completion in ARbitrary Poses. Given a partial pointcloud of an object, SCARP learns a disentangled feature representation of pose and shape by relying on rotationally equivariant pose features and geometric shape features trained using a multi-tasking objective. Unlike existing methods that depend on an external canonicalization method, SCARP performs canonicalization, pose estimation, and shape completion in a single network, improving the performance by 45% over the existing baselines. In this work, we use SCARP for improving grasp proposals on tabletop objects. By completing partial tabletop objects directly in their observed poses, SCARP enables a SOTA grasp proposal network improve their proposals by 71.2% on partial shapes. Project page: https://bipashasen.github.io/scarp
更多查看译文
关键词
3d shape completion,grasping,arbitrary poses
AI 理解论文
溯源树
样例
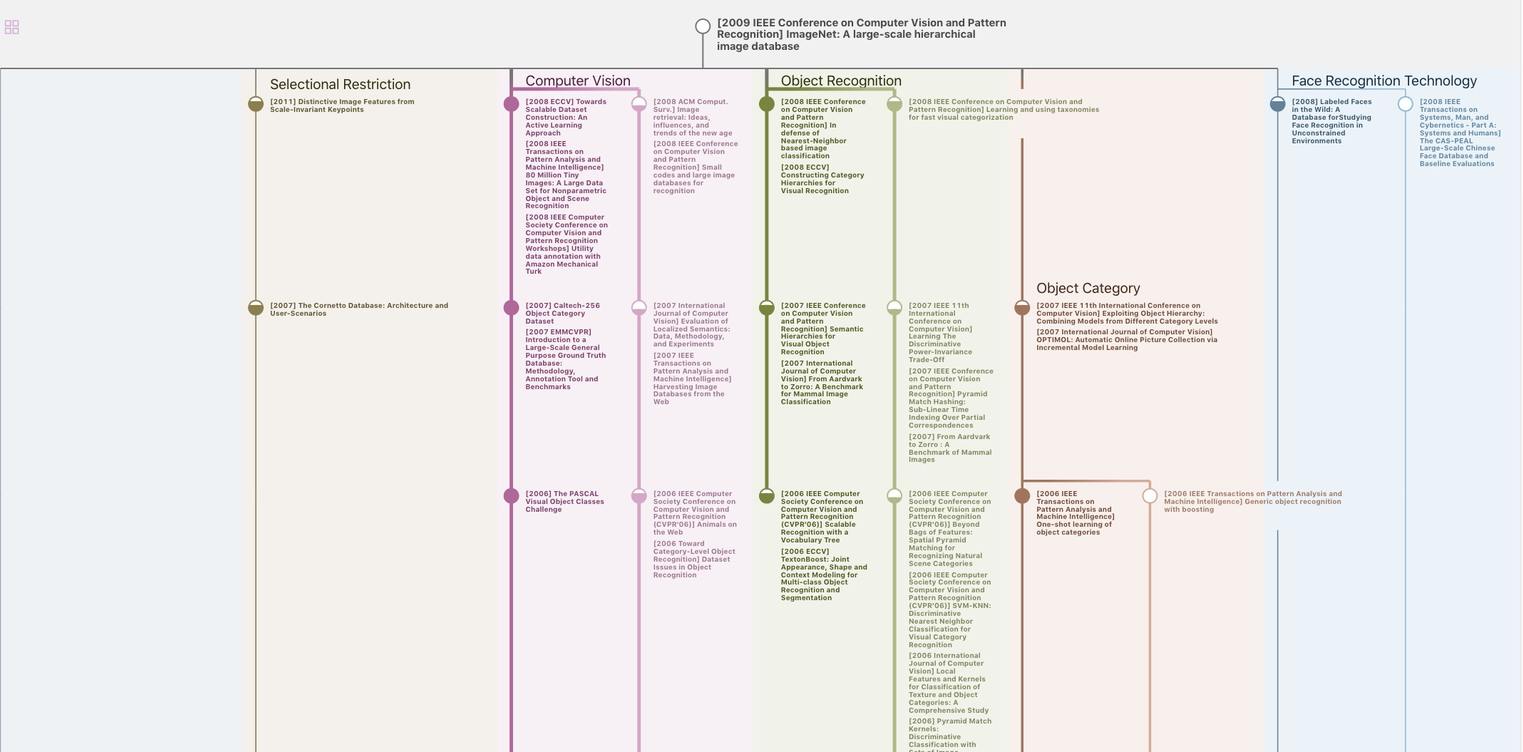
生成溯源树,研究论文发展脉络
Chat Paper
正在生成论文摘要