Long Range Pooling for 3D Large-Scale Scene Understanding
CVPR 2023(2023)
摘要
Inspired by the success of recent vision transformers and large kernel design in convolutional neural networks (CNNs), in this paper, we analyze and explore essential reasons for their success. We claim two factors that are critical for 3D large-scale scene understanding: a larger receptive field and operations with greater non-linearity. The former is responsible for providing long range contexts and the latter can enhance the capacity of the network. To achieve the above properties, we propose a simple yet effective long range pooling (LRP) module using dilation max pooling, which provides a network with a large adaptive receptive field. LRP has few parameters, and can be readily added to current CNNs. Also, based on LRP, we present an entire network architecture, LRPNet, for 3D understanding. Ablation studies are presented to support our claims, and show that the LRP module achieves better results than large kernel convolution yet with reduced computation, due to its non-linearity. We also demonstrate the superiority of LRPNet on various benchmarks: LRPNet performs the best on ScanNet and surpasses other CNN-based methods on S3DIS and Matterport3D. Code will be avalible at https://github.com/li-xl/LRPNet.
更多查看译文
关键词
long range,3d,large-scale large-scale
AI 理解论文
溯源树
样例
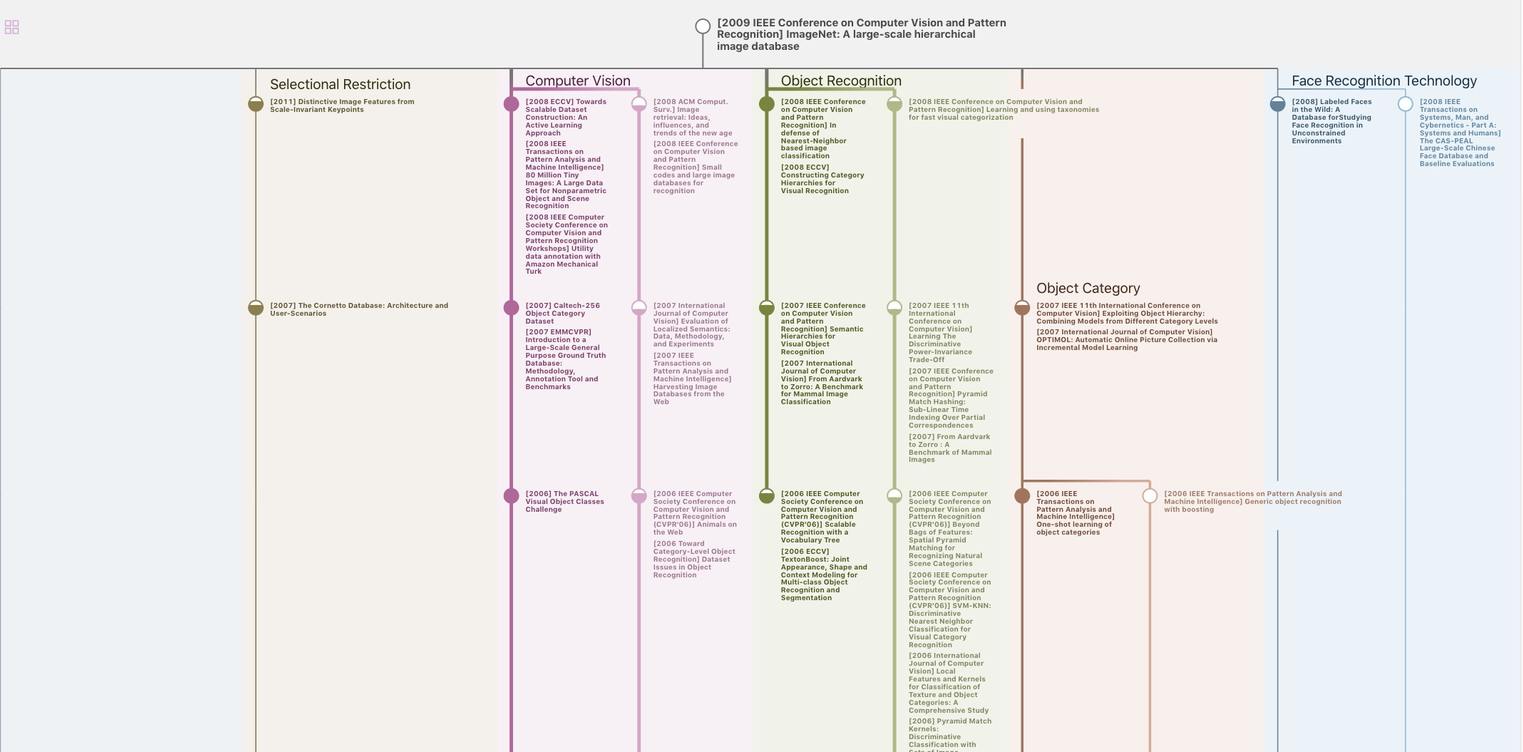
生成溯源树,研究论文发展脉络
Chat Paper
正在生成论文摘要