Distilling Accurate Descriptors from Multi-Source Experimental Data for Discovering Highly Active Perovskite OER Catalysts
arxiv(2023)
摘要
Perovskite oxides are promising catalysts for oxygen evolution reaction (OER), yet the huge chemical space remains largely unexplored due to the lack of effective approaches. Here, we report the distilling of accurate descriptors from multi-source experimental data for accelerated catalysts discovery by using the new method SCMT-SISSO that overcomes the challenge of data inconsistency between different sources. While many previous descriptors for the catalytic activity were proposed based on respective small datasets, we obtained the new 2D descriptor (d_B, n_B) based on 13 experimental datasets collected from different publications and the SCMT-SISSO. Great universality and predictive accuracy, and the bulk-surface correspondence, of this descriptor have been demonstrated. With this descriptor, hundreds of unreported candidate perovskites with activity greater than the benchmark catalyst Ba0.5Sr0.5Co0.8Fe0.2O3 were identified from a large chemical space. Our experimental validations on five candidates confirmed the three highly active new perovskite catalysts SrCo0.6Ni0.4O3, Rb0.1Sr0.9Co0.7Fe0.3O3, and Cs0.1Sr0.9Co0.4Fe0.6O3.
更多查看译文
关键词
perovskite,catalysts,multi-source
AI 理解论文
溯源树
样例
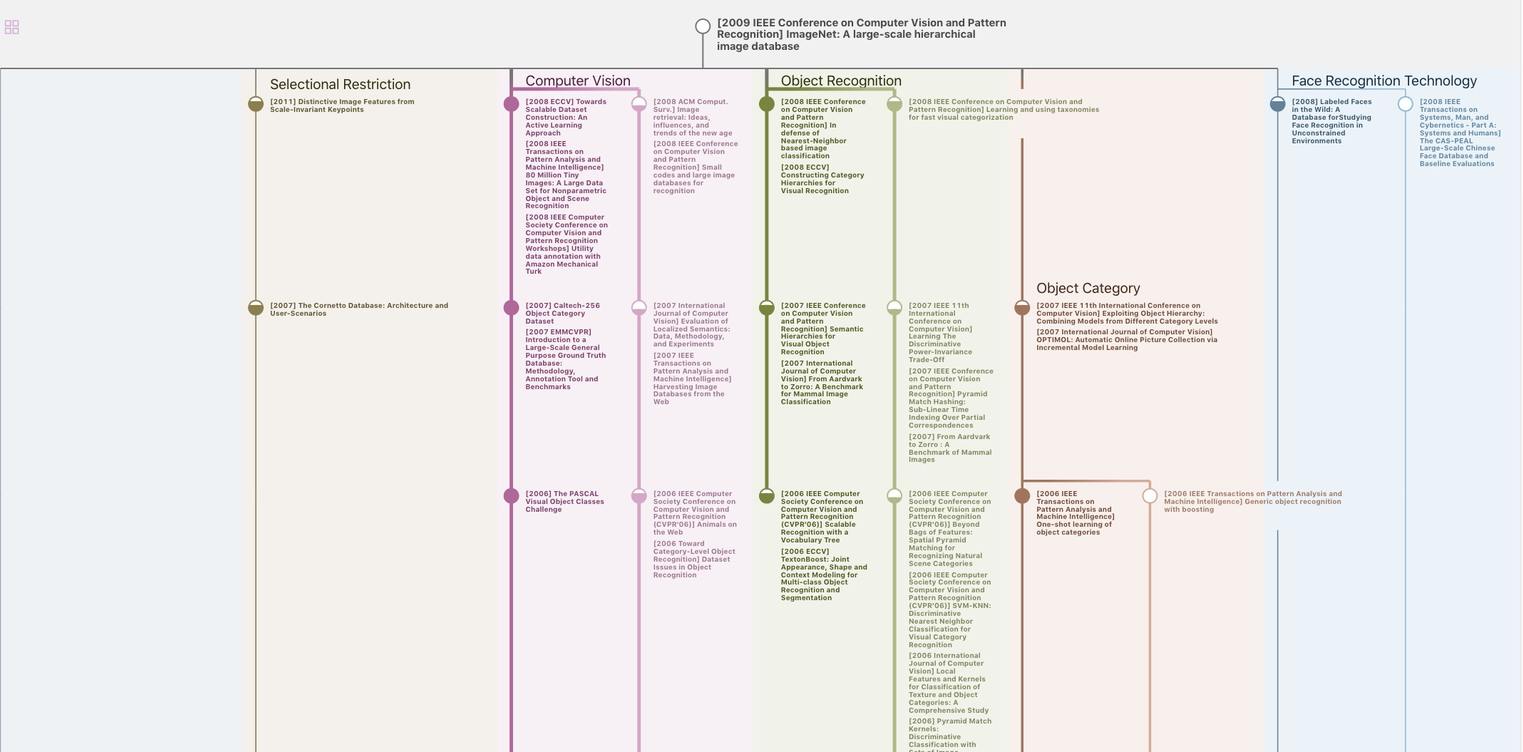
生成溯源树,研究论文发展脉络
Chat Paper
正在生成论文摘要