The Recent Advances in Automatic Term Extraction: A survey
arxiv(2023)
摘要
Automatic term extraction (ATE) is a Natural Language Processing (NLP) task that eases the effort of manually identifying terms from domain-specific corpora by providing a list of candidate terms. As units of knowledge in a specific field of expertise, extracted terms are not only beneficial for several terminographical tasks, but also support and improve several complex downstream tasks, e.g., information retrieval, machine translation, topic detection, and sentiment analysis. ATE systems, along with annotated datasets, have been studied and developed widely for decades, but recently we observed a surge in novel neural systems for the task at hand. Despite a large amount of new research on ATE, systematic survey studies covering novel neural approaches are lacking. We present a comprehensive survey of deep learning-based approaches to ATE, with a focus on Transformer-based neural models. The study also offers a comparison between these systems and previous ATE approaches, which were based on feature engineering and non-neural supervised learning algorithms.
更多查看译文
关键词
automatic term extraction
AI 理解论文
溯源树
样例
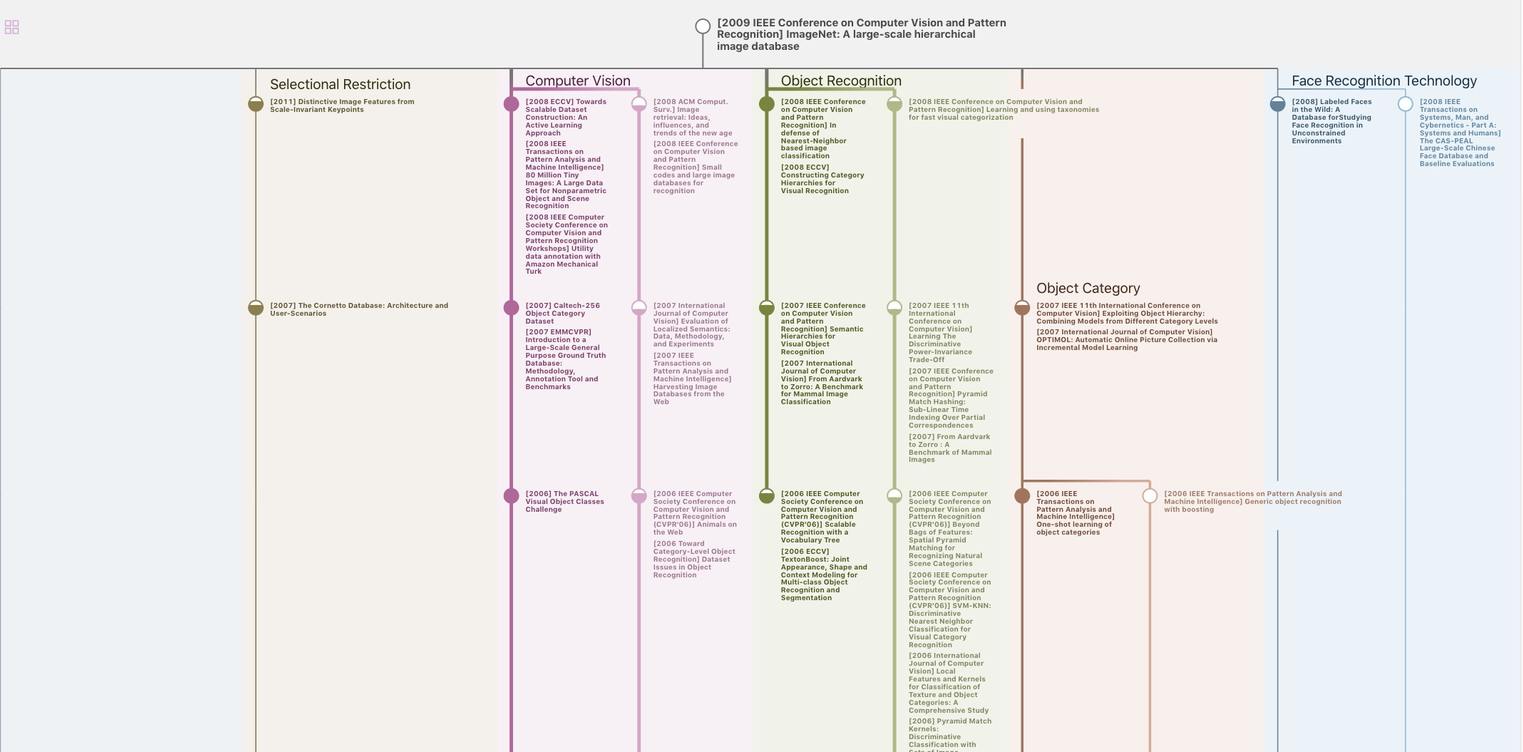
生成溯源树,研究论文发展脉络
Chat Paper
正在生成论文摘要