Improving Noise Robustness for Spoken Content Retrieval Using Semi-Supervised ASR and N-Best Transcripts for BERT-Based Ranking Models
2022 IEEE Spoken Language Technology Workshop (SLT)(2023)
摘要
BERT-based re-ranking and dense retrieval (DR) systems have been shown to improve search effectiveness for spoken content retrieval (SCR). However, both methods can still show a reduction in effectiveness when using ASR transcripts in comparison to accurate manual transcripts. We find that a known-item search task on the How2 dataset of spoken instruction videos shows a reduction in mean reciprocal rank (MRR) scores of 10-14%. As a potential method to reduce this disparity, we investigate the use of semi-supervised ASR transcripts and N-best ASR transcripts to mitigate ASR errors for spoken search using BERT-based ranking. Semi-supervised ASR transcripts brought 2-5.5% MRR improvements over standard ASR transcripts and our N-best early fusion methods for BERT DR systems improved MRR by 3-4%. Combining semi-supervised transcripts with N-best early fusion for BERT DR reduced the MRR gap in search effectiveness between manual and ASR transcripts by more than 50% from 14.32% to 6.58%.
更多查看译文
关键词
spoken content retrieval,BERT re-ranking,BERT dense retrieval,N-best BERT-based retrieval
AI 理解论文
溯源树
样例
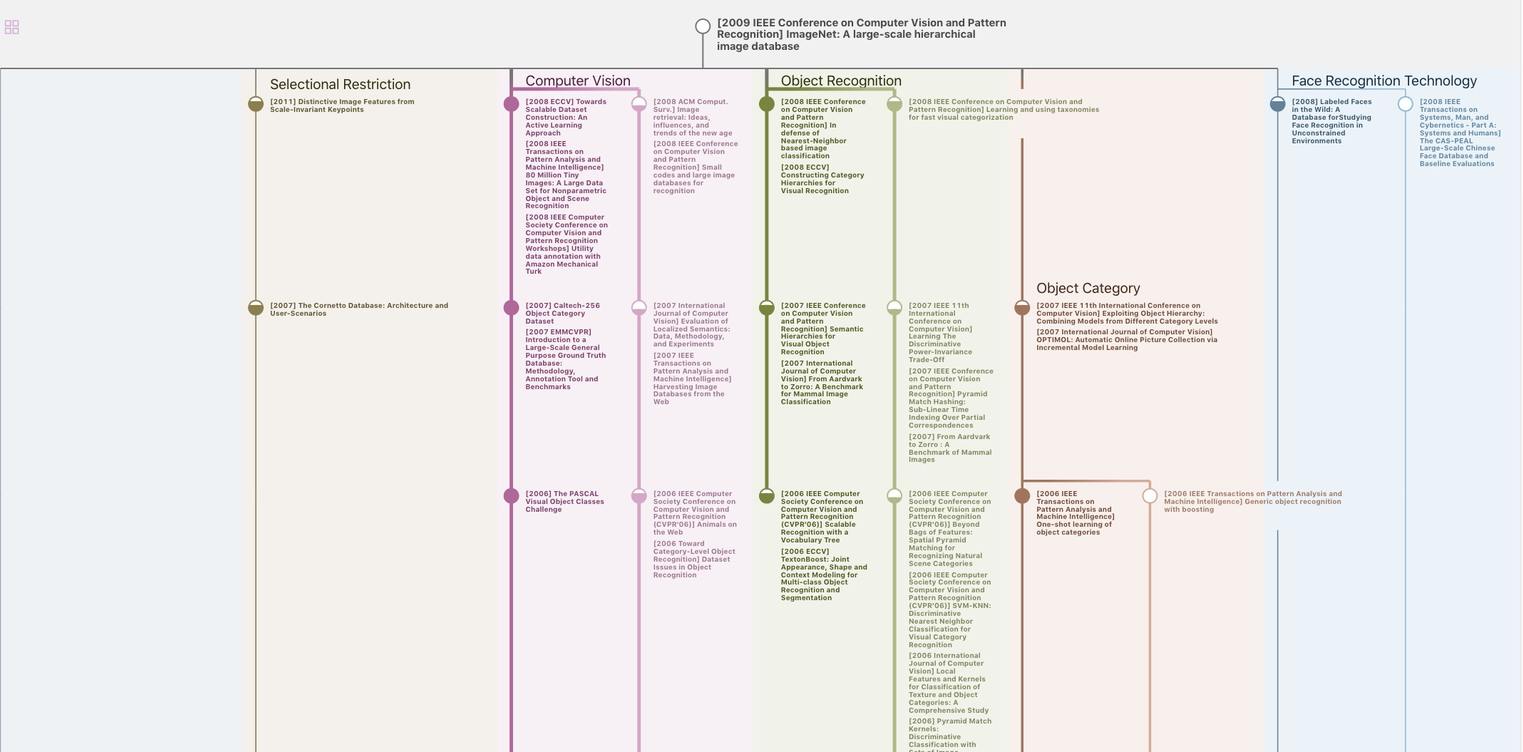
生成溯源树,研究论文发展脉络
Chat Paper
正在生成论文摘要