Weighted Minwise Hashing Beats Linear Sketching for Inner Product Estimation
PROCEEDINGS OF THE 42ND ACM SIGMOD-SIGACT-SIGAI SYMPOSIUM ON PRINCIPLES OF DATABASE SYSTEMS, PODS 2023(2023)
摘要
We present a new approach for independently computing compact sketches that can be used to approximate the inner product between pairs of high-dimensional vectors. Based on the Weighted MinHash algorithm, our approach admits strong accuracy guarantees that improve on the guarantees of popular linear sketching approaches for inner product estimation, such as CountSketch and Johnson-Lindenstrauss projection. Specifically, while our method exactly matches linear sketching for dense vectors, it yields significantly lower error for sparse vectors with limited overlap between non-zero entries. Such vectors arise in many applications involving sparse data, as well as in increasingly popular dataset search applications, where inner products are used to estimate data covariance, conditional means, and other quantities involving columns in unjoined tables. We complement our theoretical results by showing that our approach empirically outperforms existing linear sketches and unweighted hashing-based sketches for sparse vectors.
更多查看译文
关键词
inner product estimation,vector sketching,join-size estimation
AI 理解论文
溯源树
样例
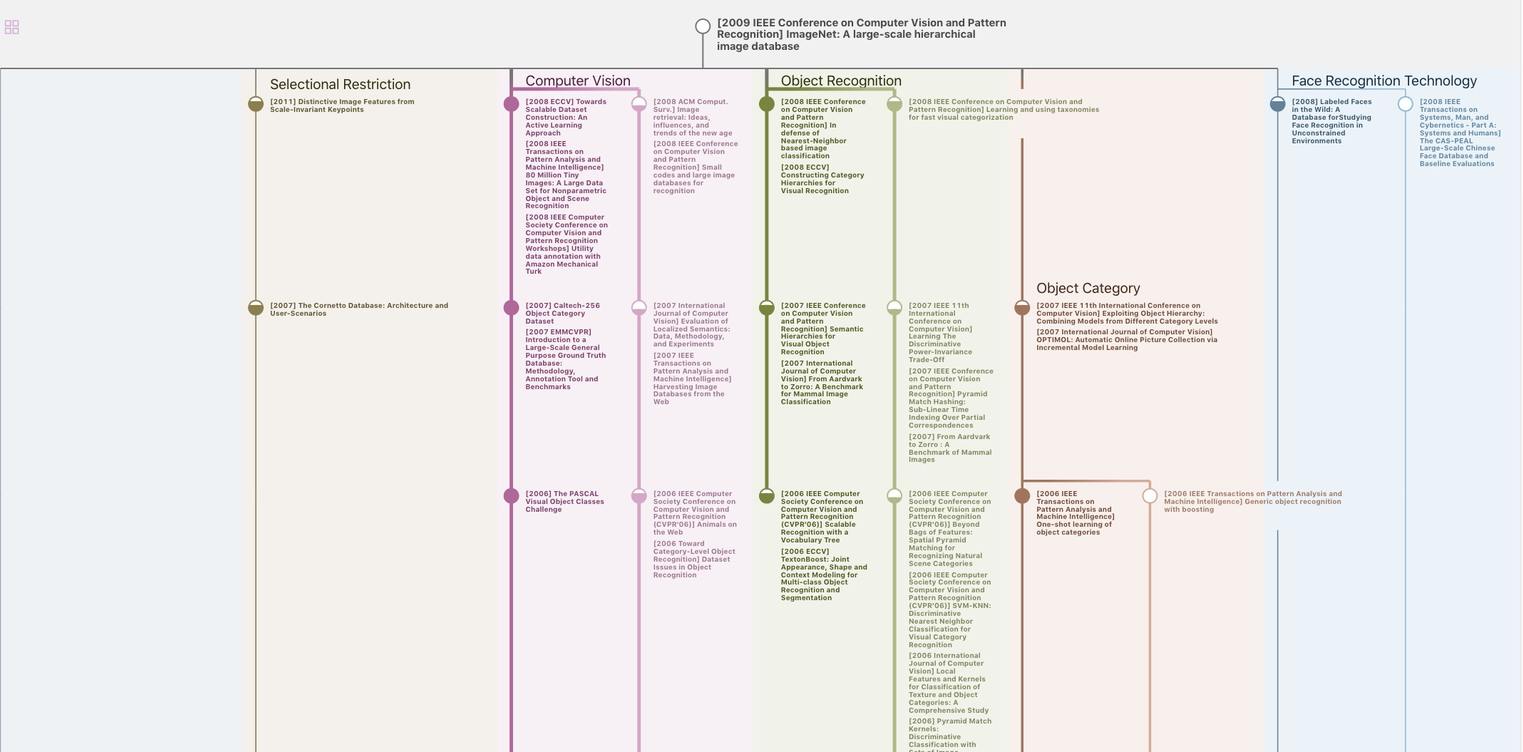
生成溯源树,研究论文发展脉络
Chat Paper
正在生成论文摘要