Plastic Classification With NIR Hyperspectral Images and Deep Learning
IEEE Sensors Letters(2023)
摘要
Because most plastics are incompatible and cannot be blended, polymers must be separated before recycling. We propose a sensor-based classifier that collects NIR wavelengths from polymers to estimate their composition. Unlike prior research, this work aims to apply machine learning to the difficult problem of categorizing low-reflectance black polymers in NIR and without complimentary sensory data. We collected 1491 hyperspectral pictures of roughly 4500 plastic pieces from an industrial recycling operation. Using N-BEATS, an ensemble deep learning approach for waveform decomposition, we achieved an overall f1-score of 0.79 and accuracy of 0.79 for all plastics, 0.9 for colored plastics, and 0.67 for black plastics. The method achieves noteworthy performance results, as black polymer differentiation in the NIR has been deemed unfeasible by the majority of the existing literature.
更多查看译文
关键词
Sensor applications,deep learning,hyperspectral imaging,polymer separation
AI 理解论文
溯源树
样例
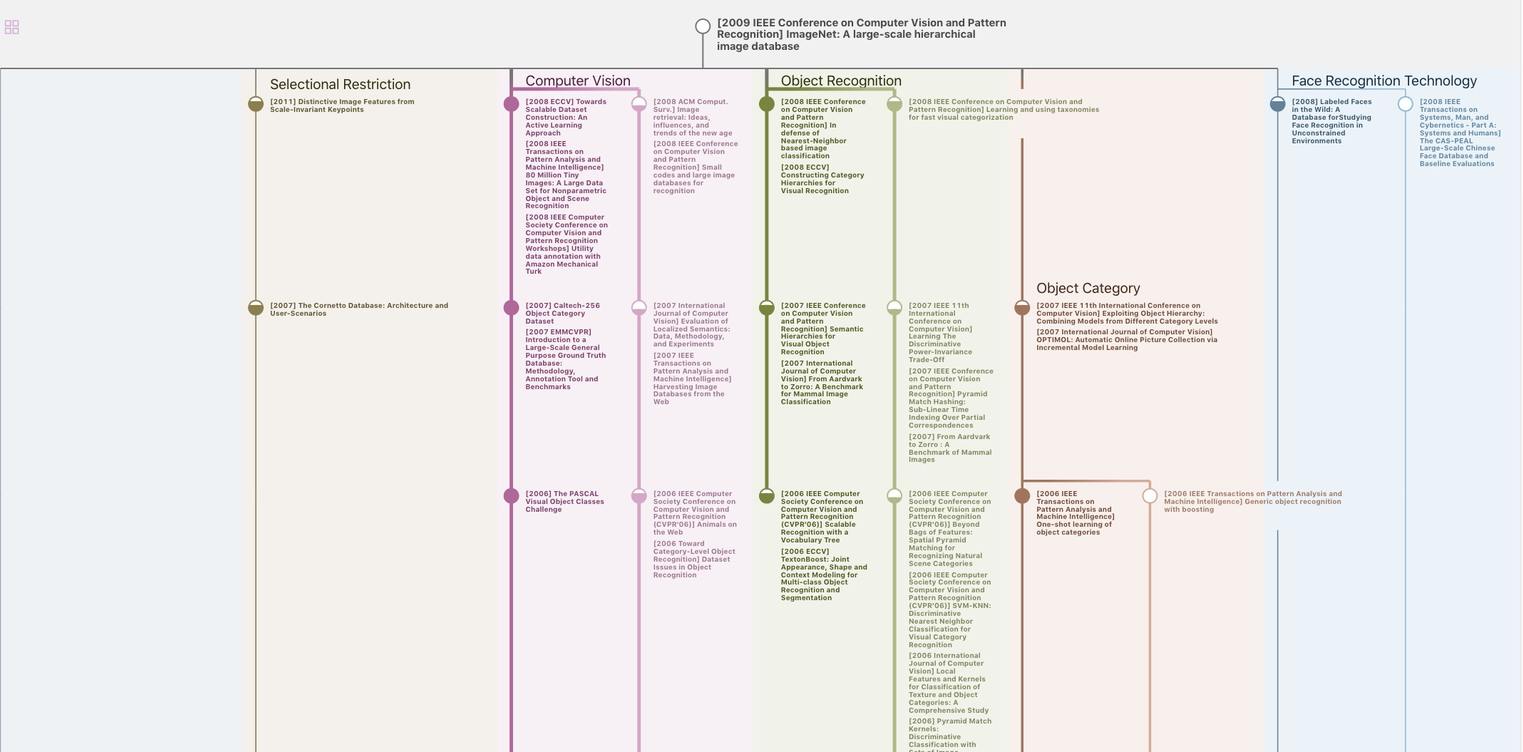
生成溯源树,研究论文发展脉络
Chat Paper
正在生成论文摘要