Bayesian areal disaggregation regression to predict wildlife distribution and relative density with low-resolution data
biorxiv(2023)
摘要
For species of conservation concern and human-wildlife conflict, it is imperative that spatial population data be available to design adaptive-management strategies and be prepared to meet challenges such as land use and climate change, disease outbreaks, and invasive species spread. This can be difficult, perhaps impossible, if spatially explicit wildlife data are not available. Low-resolution areal counts, however, are common in wildlife monitoring, that is, the number of animals reported for a region, usually corresponding to administrative subdivisions, for example, region, province, county, departments, or cantons. Bayesian areal disaggregation regression is a solution to exploit areal counts and provide conservation biologists with high-resolution species distribution predictive models. This method originated in epidemiology but lacks experimentation in ecology. It provides a plethora of applications to change the way we collect and analyze data for wildlife populations. Based on high-resolution environmental rasters, the disaggregation method disaggregates the number of individuals observed in a region and distributes them at the pixel level (e.g., 5 x 5 km or finer resolution), thereby converting low-resolution data into a high-resolution distribution and indices of relative density. In our demonstrative study, we disaggregated areal count data from hunting bag returns to disentangle the changing distribution and population dynamics of three deer species (red, sika, and fallow) in Ireland from 2000 to 2018. We show an application of the Bayesian areal disaggregation regression method and document marked increases in relative population density and extensive range expansion for each of the three deer species across Ireland. We challenged our disaggregated model predictions by correlating them with independent deer surveys carried out in field sites and alternative deer distribution models built using presence-only and presence-absence data. Finding a high correlation with both independent data sets, we highlighted the ability of Bayesian areal disaggregation regression to accurately capture fine-scale spatial patterns of animal distribution. This study uncovers new scenarios for wildlife managers and conservation biologists to reliably use regional count data disregarded so far in species distribution modeling. Thus, it represents a step forward in our ability to monitor wildlife population and meet challenges in our changing world.
更多查看译文
关键词
Bayesian modeling, count data, deer, deer distribution, deer distribution and density, deer in Ireland, deer overabundance, ecological modeling, low-resolution data, species distribution models, wildlife census data, wildlife conservation and management
AI 理解论文
溯源树
样例
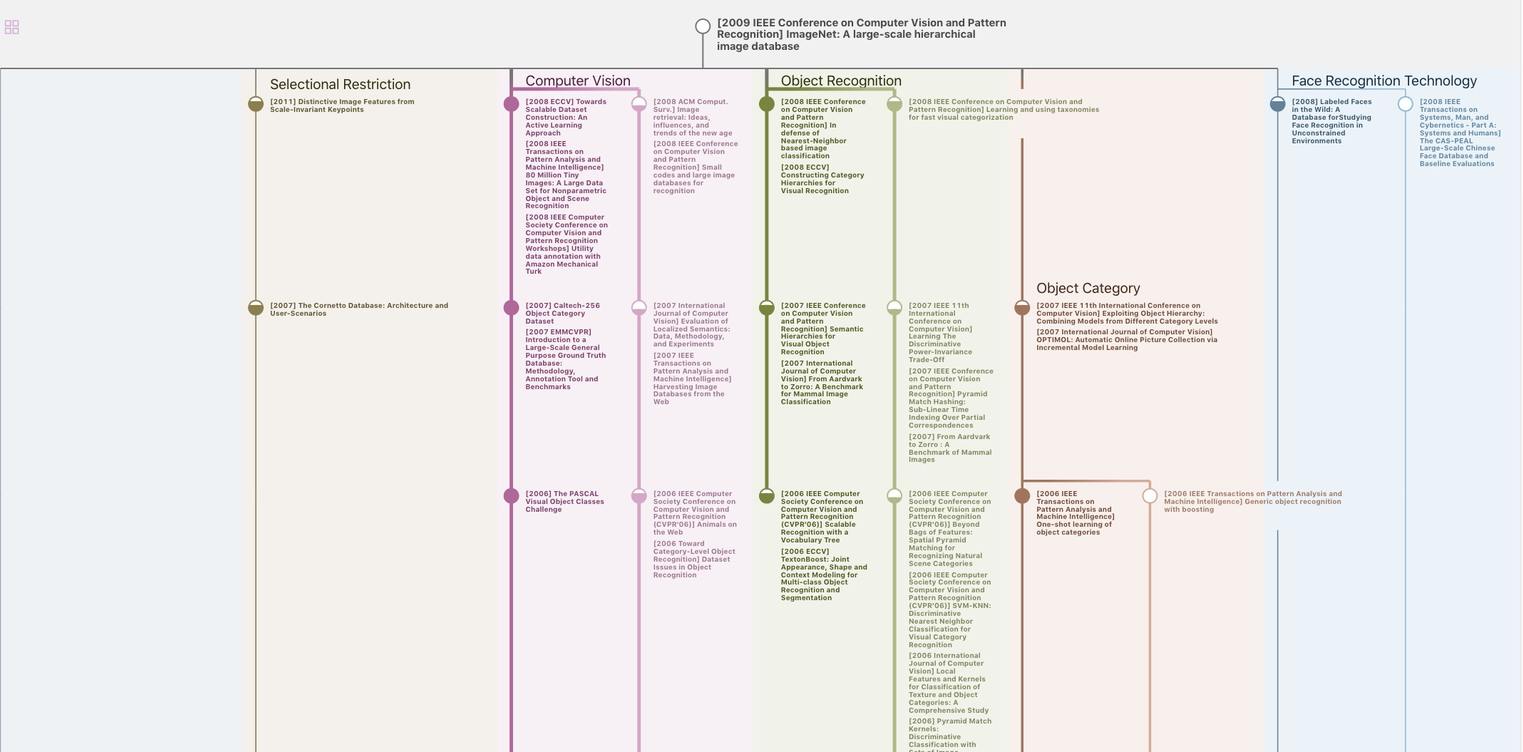
生成溯源树,研究论文发展脉络
Chat Paper
正在生成论文摘要