Application of Innovative Machine Learning Techniques for Long-Term Rainfall Prediction
Pure and Applied Geophysics(2023)
摘要
Rainfall forecasting is critical because it is the componen t that has the strongest link to natural disasters such as landslides, floods, mass movements, and avalanches. The present study focuses on the application of four machine learning techniques, namely, multiple linear regression (MLR), support vector regression (SVR), multivariate adaptive regression splines (MARS), and random forest (RF), for the prediction of daily and mean weekly rainfall at Ranichauri station, located in the Tehri Garhwal district of Uttarakhand. For this, 18 years of data (2000–2017) of the meteorological variables such as maximum and minimum temperature, morning and afternoon relative humidity, wind speed, morning and afternoon vapor pressure, morning &afternoon wind direction, solar radiation, evaporation, and rainfall were collected from Agromet Forest Unit (AMFU), Ranichauri. Validation of the developed models was achieved by using statistical parameters such as root mean square error (RMSE), index of agreement ( d ), correlation coefficient ( r ), and Kling-Gupta efficiency (KGE). For daily and mean weekly rainfall prediction, the overall performance-wise ranking of models was RF, MARS, SVR, and MLR. Among the four models, results showed that the RF model outperformed in both calibration and testing periods with RMSE, d , r , and KGE = 5.70, 0.93, 0.94, and 0.54 for daily and 10.54, 0.97, 0.96, and 0.77 for weekly rainfall prediction. Consequently, it can be surmised that the RF model is the best in class and delivers a strong potential for predicting daily and mean weekly rainfall at Ranichauri station.
更多查看译文
关键词
Rainfall prediction,Gamma test,Multiple linear regression,Support vector machine,Multivariate adaptive regression splines,Random forest
AI 理解论文
溯源树
样例
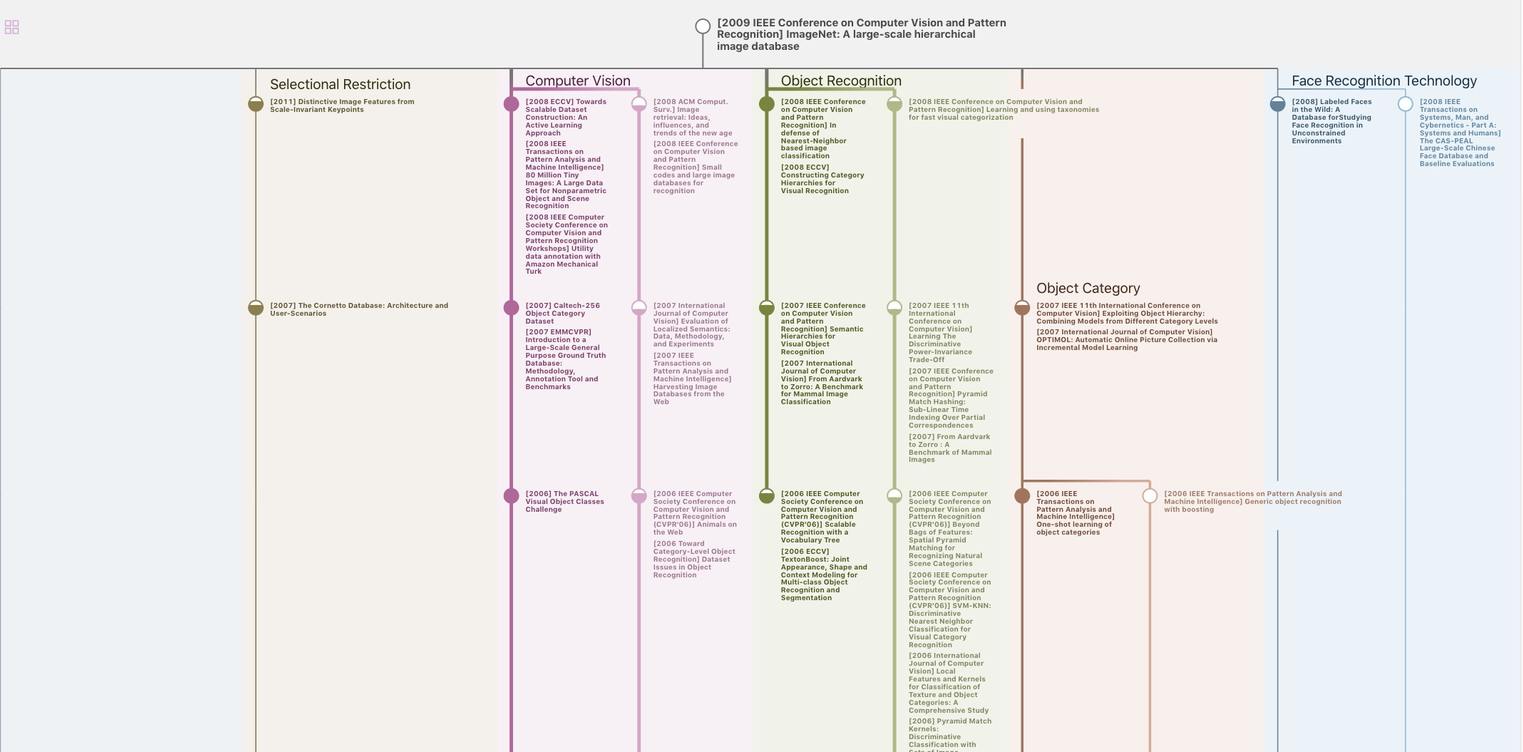
生成溯源树,研究论文发展脉络
Chat Paper
正在生成论文摘要